Abstract
A meta-analysis was performed on 351 studies from 17 articles published between 1990 and 2016 to determine how a water use efficiency (WUE) treatment is affected by irrigation systems and management practices on clay and clay loam soils in a semi-arid environment relative to a rainfed control. Several explanatory variables (moderators) were examined to determine their impact on WUE such as crop type, irrigation capacity, rainfall, soil type, planting time, and nitrogen application. Results were sub-grouped by irrigation system. Overall, the impact of irrigation system on WUE directly correlated with the efficiency of the irrigation system. Subsurface drip and center pivot irrigation systems had the largest impacts on WUE with increases of 147 and 99%, respectively, compared to a 14% increase under furrow irrigation. Corn (Zea mays L.) had a higher response to WUE in subsurface drip irrigation (260%) compared to center pivot irrigation (46%), whereas WUE in cotton (Gossypium hirsutum L.) had a 71% change in center pivot systems compared to 63% under subsurface drip. The biggest increases in WUE relative to a rainfed control were for sorghum (Sorghum bicolor (L.) Moench), which had a 13% change under furrow irrigation, 160% change under center pivot and 341% under subsurface drip.
Similar content being viewed by others
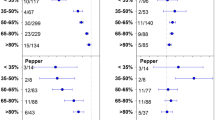
Explore related subjects
Discover the latest articles, news and stories from top researchers in related subjects.Avoid common mistakes on your manuscript.
Introduction
The Texas High Plains region constitutes over 93,000 km2 that covers 48 counties, spanning the Texas panhandle to just north of the Midland–Odessa area (TWDB, 2019). Agriculture contributes approximately $7 billion annually in economic activity to the Texas High Plains economy, producing 25% of United States cotton (Gossypium hirsutum L.) production and annually markets more than 6 million cattle, or 28% of the United States fed cattle production (TCFA, 2019). Other major crops produced in this semi-arid environment include corn (Zea mays L.), sorghum (Sorghum bicolor (L.) Moench), wheat (Triticum aestivum L.), peanut (Arachis hypogaea L.), and sunflower (Helianthus annuus L.). Since pre-development in the 1950s, intensive irrigation for crop production has depleted over half of the saturated thickness of the Ogallala Aquifer (McGuire 2017). Adoption of irrigation technology began with low-pressure systems such as furrow and gravity flow, but more efficient technology such as center pivot systems using Low-Elevation Spray Application (LESA), Mid-Elevation Spray Application (MESA), and Low-Energy Precision Application (LEPA) nozzles and as well as Subsurface Drip Irrigation (SDI) are more widely used (Seo et al. 2008; Amosson et al. 2011). Numerous studies have compared yield potential and efficiencies of these systems. Deficit irrigation strategies are being explored to reduce water inputs, but the impact on crop yield and water use efficiency (WUE) is uncertain (Colaizzi et al. 2009; Rudnick et al. 2019).
Meta-analysis is a quantitative technique that aggregates the results of individual studies to derive greater statistical power (Borenstein et al. 2009). Meta-analyses have been performed in agriculture to determine the impact of various management practices on crop yield and WUE. Fan et al. (2018) performed a meta-regression on WUE for cotton and wheat production in China across various management practices under furrow and micro-irrigation systems. They found maximal WUE in cotton was achieved by reducing water application by 51%, but it resulted in a loss in lint yield of 51%, whereas a 30% reduction in water applied for wheat reduced yield by 15%. Adu et al. (2018) performed a meta-analysis to determine crop yield performance under full, deficit, and partial root-zone drying irrigation for nine crop species globally. Overall, deficit irrigation reduced crop yield, with the least impact on arable field crops (corn, cotton, and potato) and greater impacts on vegetable fruit crops. A meta-regression by Sadras (2009) found that deficit irrigation increased water productivity (analogous to WUE) by 80% relative to high irrigation controls. Zhang et al. (2018) found that for C3 plants, WUE increased by 14% under mild and moderate drought stress, but had zero change under severe stress. C4 crops experienced declines of 3.5, 4.1 and 24% under mild, moderate, and severe stress, respectively.
The objective of this study was to perform a meta-analysis to determine the impact of irrigation system and management operations on WUE in the Texas High Plains. Publications with experiments conducted on corn, cotton, sorghum, and wheat using furrow, center pivot, and subsurface drip systems were analyzed and compared to rainfed systems.
Materials and methods
Database preparation
Data were collected from studies where irrigation treatments could be compared to rainfed controls. A systematic and exhaustive literature search was conducted in February and March 2016 using Google Scholar and the Web of Science search engines with the following keywords: water use efficiency, WUE, yield, Ogallala, dryland, rainfed, deficit irrigation, Texas. Articles were restricted to those using data obtained since 1990. Two rounds of screening took place. The first screening of articles contained publications relevant to crop yield and WUE. The second round of screening determined the final papers to be selected to database entry: 1) the experiment characteristics had to include a dryland observation, and 2) the articles had to report yield and WUE for each irrigation treatment. The database ended up with 17 papers for a total of 351 observations located in the Texas High Plains covering corn, cotton, sorghum and wheat on furrow, center pivot with LEPA, LESA and MESA nozzles, and SDI systems (Table 1).
A database was created by listing the management data in each study. The WUE data were arranged as paired observations, in that multiple irrigation treatments were compared to a single rainfed control. Other data collected were related to the experimental design such as location, weather, and number of replications, management data including tillage practices, row spacing, fertilizer and chemical application, and external factors such as rainfall received. Relevant moderators are shown in Table 2. All studies used conventional tillage practices, so this was not included in the list of moderators. Because the meta-analysis cannot be performed on continuous variables, the moderators were sub-divided into categories as defined by Bordovsky (2018).
Data analysis
The meta-analysis was conducted using the Comprehensive Meta-Analysis software Version 3 developed by Borenstein et al. (2013). A random effects model was used to assess WUE under deficit irrigation as we assumed the true effect varied across studies (Borenstein et al. 2009). A response ratio was used as the effect size and was calculated as (Hedges et al. 1999):
where LnRR represents the natural logarithm of the response ratio, and \({WUE}_{trt}\) is the irrigation treatment and \({WUE}_{ctl}\) is the dryland control. WUE was defined as yield/ET, although it was not specified in some papers. Differences such as the way ET was measured or estimated, the measurement of stored water, and the method of harvest (hand vs machine) could effect the outcome of this variable in the meta-analysis. Each observation was weighted using a non-parametric variance, where \({n}_{trt}\) is the number of replications of each treatments, and \({n}_{ctl}\) is the number of replications for each control. Each irrigation treatment, and therefore corresponding WUE, was designated as a single study. These partial observations comparing more than one treatment to a single control often result in dependency within the effect sizes, thereby increasing Type I errors (Scammacca et al. 2014). To address the dependency of the effect sizes, the variances of the studies were downweighted by a factor of \({m}^{0.5}\), where m is the number of trials in each paper. The variance was also adjusted by dividing the control group sample size by \({c}^{0.5}\), where c is the number of treatments under each control (Ashworth et al. 2018).
Heterogeneity and publication bias
Heterogeneity was assessed using Cochran’s Q statistic to test the null hypothesis that all studies share a common effect size, where Q is calculated as
where \({W}_{i}\) is the weight of the inverse-variance, \({ES}_{i}\) is the effect size, and \(M\) is the summary effect across studies \(i\) through \(n\). The Q statistic has a chi-square distribution with number of studies (n) minus one degree of freedom. The null is rejected if p values are less than 0.10. The I2 statistic describes the percentage of variation across studies due to heterogeneity rather than chance and is calculated for each study as
where Q is the Cochran’s heterogeneity statistic and degrees of freedom. Values of I2 range from 0 to 100%, where values of 25, 50, and 75% represent low, medium, and high heterogeneity (Higgins 2003; Borenstein et al. 2009).
Publication bias represents the biggest source of Type I errors in meta-analysis (Harrison 2011). Graphical and statistical methods were used for assessing publication bias. Funnel plots are the primary method used and present the effect size plotted against the standard error, which places the effect sizes of small studies at the bottom of the funnel and larger studies concentrated at the top. In the absence of bias, funnel plots will be symmetrical (Sterne et al. 2005). Egger et al. (1997) developed a statistical method to measure funnel plot asymmetry by regressing the effect size against the inverse standard error. The intercept of the regression line captures the bias while the slope indicates the size and direction of the treatment effect. Duval and Tweedie (2000) developed a non-parametric trim and fill procedure to estimate the number of missing studies and the impact those studies would have had on the summary point estimates. Rosenberg’s (2005) fail-safe N is a method used to determine if the existence of publication bias is likely to impact the analysis. Fail-safe numbers represent the number of missing studies that would nullify the observed treatment effects. If the fail-safe N is very large, then the effects are robust.
Results
Overall
Several explanatory variables (moderators) were examined to determine their impact on WUE. Each moderator represents a categorical variable. Heterogeneity statistics are reported in Table 3. Irrigation capacity and soil type had significant p values indicating the potential for heterogeneity in these moderators; however, all I2 statistics were 0.0%, which makes the Q statistic null and void.
The effect sizes and their confidence intervals and p values for the overall response of WUE is shown in Fig. 1. There was a 73% overall increase in irrigated WUE from dryland controls (n = 351; CI 1.407–2.133; p value: < 0.001). Across crop type, the irrigation response was the greatest for corn, which has the greatest crop water requirements. Corn had a mean effect of 2.25 (n = 22; CI 0.99–5.14; p value: 0.054) followed by a mean response in sorghum of 1.99 (n = 171; CI 1.47–2.70; p value: < 0.001), 1.69 (n = 68; CI 1.13–2.52; p value: 0.010) in cotton and 1.20 (n = 90; CI 0.75–1.90; p value: 0.450) in wheat. These resulted in changes of 125, 69, 99 and 20%, respectively. Furrow only had a 14% (n = 76; CI 0.77–1.68; p value: 0.517) increase in WUE compared to a 99% (n = 235; CI 1.52–2.59; p value: < 0.001) increase in center pivot and 147% (n = 40; CI 1.30–4.72; p value: 0.006) increase with SDI. Systems with average (medium) irrigation applied had the greatest WUE response than compared to low and high applied irrigation. In the low irrigation application category, there were 22 corn, 53 cotton, 85 sorghum, and 22 wheat observations, representing 6, 31, 50, and 13%, respectively. The medium irrigation application category had 6 corn, 15 cotton, 68 sorghum, and 16 wheat observations, representing 6, 14, 65, and 155%, respectively. The high irrigation application category had five corn, 18 sorghum, and 52 wheat observations, representing 7, 24, and 52%, respectively. Rainfall changes for low, average, and high rainfall amounts were 78% (n = 173; CI 1.32–2.40; p value: 0.002), 52% (n = 109; CI 1.06–2.19; p value: 0.023) and 102% (n = 69; CI 1.25–3.28; p value: 0.004). The high rainfall category generated the greatest change in WUE. However, the low rainfall category had 26% greater change than the medium rainfall category. Average applied irrigation was comparable across all rainfall categories. The soil type was 91% Pullman clay loam in the high rainfall category compared to 86 and 82% for the low and medium, respectively. The impact of four soil types indicated Pullman soils had the greatest change. Pullman silty clay loam had the greatest impact on WUE, resulting in a 250% (n = 15; CI 1.89–6.49; p value: < 0.001) change compared to the rainfed control. Pullman clay loam, Abilene clay loam, and Olton loam had a change of 63% (n = 300; CI 1.29–2.06; p value: < 0.001), 41% (n = 12; CI 0.51–3.84; p value: 0.507) and − 5% (n = 24; CI 0.33–2.73; p value: 0.927), meaning WUE on Olton loam soils performed better under rainfed conditions.
Summary effect sizes of moderator variables for water use efficiency (WUE) in the Texas High Plains. Vertical line represents a null effect and is placed at the “1” due to the logarithmic form of the effect sizes. Horizontal bars represent 95% confidence intervals, change reflects the raw percent changes between the treatment and control, and the p value represents the statistical significance of each moderator
Crops planted early (n = 177; CI 0.32–0.98; p value: 0.000) were likely to benefit more from spring rainfall than crops planted later (n = 174; CI 0.21–0.75; p value: 0.000) in the season. The impact of low amounts of nitrogen applied resulted in the greatest increase in WUE of 126% (n = 70; CI 1.40–3.66; p value: 0.001) compared to the dryland control. High applied nitrogen has the least effect at 87% (n = 95; CI 1.23–2.84; p value: 0.004) compared to average nitrogen application at 54% (n = 186; CI 1.17–2.03; p value: 0.002). An analysis of the year interval of planting was used to account for weather and potential technology impacts. Studies with observations planted from 1990–1995 had a 36% (n = 109; CI 0.92–2.00; p value: 0.122) change compared to studies planted more recently 89% (n = 35; CI 1.14–3.11; p value: 0.013). Studies with planted observations between 2000 and 2005 had a 177% (n = 68; CI 1.65–4.65; p value: < 0.001) change, but also had the largest confidence interval.
Subgroup analysis
Results were sub-grouped by irrigation system to understand its influence on WUE. The summary effects in Fig. 1 for irrigation systems indicate that only the center pivot and SDI systems had significant results. Effect sizes for each irrigation system are presented in Figs. 2, 3 and 4.
Summary effect sizes of moderator variables for water use efficiency (WUE) sub-grouped by furrow irrigation (n = 76) in the Texas High Plains. Vertical line represents a null effect and is placed at the “1” due to the logarithmic form of the effect sizes. Horizontal bars represent 95% confidence intervals, change reflects the raw percent changes between the treatment and control, and the p value represents the statistical significance of each moderator
Summary effect sizes of moderator variables for water use efficiency (WUE) sub-grouped by center pivot irrigation (n = 235) in the Texas High Plains. Vertical line represents a null effect and is placed at the “1” due to the logarithmic form of the effect sizes. Horizontal bars represent 95% confidence intervals, change reflects the raw percent changes between the treatment and control, and the p value represents the statistical significance of each moderator
Summary effect sizes of moderator variables for water use efficiency (WUE) sub-grouped by subsurface drip irrigation (SDI) (n = 40) in the Texas High Plains. Vertical line represents a null effect and is placed at the “1” due to the logarithmic form of the effect sizes. Horizontal bars represent 95% confidence intervals, change reflects the raw percent changes between the treatment and control, and the p value represents the statistical significance of each moderator
Furrow irrigation
Furrow irrigation was used in 76 out of the 351 studies (Table 1), and their effect sizes were small in all moderator categories (Fig. 2). Sorghum (n = 48) and wheat (n = 28) were the only crops grown under the furrow irrigation system. Sorghum exhibited a 13% (CI 0.67–1.90; p value: 0.639) change and a 14% (CI: 0.64–2.06; p value: 0.655) change in wheat. There was also no large influence in WUE between low irrigation applied (10%; n = 43; CI 0.65–1.84; p value: 0.731) and high irrigation applied (12%; n = 22; CI 0.56–2.26; p value: 0.752). Average/medium irrigation application had a 37% change between irrigated and dryland controls (n = 11; CI: 0.48–3.92; p value: 0.560). In dry years (n = 28), furrow irrigation had a 6% (CI 0.57–1.99; p value: 0.853) change in WUE compared to a 22% change (CI 0.72–2.07; p value 0.468) in average rainfall years (n = 40) and no change in wet rainfall years (n = 8; CI 0.26–3.88; p value: 0.997). Field experiments using furrow irrigation were only conducted on Pullman clay loam soils, where the change was 14% (n = 76; CI 0.77–1.67; p value 0.517). Water use efficiency was influenced more with crops planted early (26%; n = 34; CI 0.70–2.27; p value: 0.433) compared to crops planted on time (5%; n = 42; CI 0.62–1.76; p value: 0.867). There were small differences on the impact of nitrogen on WUE of irrigation compared to the dryland control. Medium amounts of irrigation had a 15% change (n = 64; CI 0.74–1.81; p value: 0.533) compared to a 9% change (n = 12; CI 0.50–2.37; p value: 0.831) with high nitrogen application. Results for the year interval in which the studies were planted do not indicate improvements in WUE. Studies with observations planted between 1990 and 1995 had a 22% change (n = 48; CI 0.73–2.02; p value: 0.448). Studies with observations planted between 2005 and 2010 had a negligible effect size with a change of − 1% (n = 14; CI 0.42–2.33; p value: 0.988) and studies planted between 2010 and 2015 had a 7% change (n = 14; CI 0.46–2.52; p value: 0.871). All effect sizes for WUE under furrow irrigation had no statistically significant results due to their being only 76 observations from three field studies.
Center pivot irrigation
Observations for LEPA, LESA, MESA delivery modes were combined for the analysis of center pivot systems (Fig. 3). Plantings of corn, cotton, sorghum and wheat comprised 235 observations. Corn had a 46% change (n = 10; CI 0.47–4.60; p value: 0.514) in WUE compared to the dryland control, while cotton had a 71% change (n = 52; CI 1.09–2.67; p value: 0.02). Sorghum had the greatest response to irrigation with a change of 160% (n = 111; CI 1.76–3.83; p value: < 0.001) and wheat had the smallest mean effect change of 29% (n = 62; CI 0.61–2.74; p value: 0.511). Low and high irrigation capacities were similar with a change of 73% (n = 113; CI: 1 0.21–2.46; p value: 0.002) and 61% (n = 47; CI 0.73–3.54; p value: 0.238), respectively. Average amounts of water applied had the greatest effect of 175% (n = 75; CI 1.71–4.41; p value: < 0.001). The medium level of irrigation consisted of 52 sorghum, 14 wheat, seven cotton, and two corn observations. The sorghum observations likely inflated the effect. The high irrigation level had 30 out of 47 observations planted to wheat, which had the smallest effect size based on crop type. Conversely, WUE improved in dry and wet years, with changes of 101% (n = 133; CI 1.41–2.85; p value: 0.114) and 123% (n = 55; CI 1.30–3.84; p value: 0.004), respectively. Average rainfall conditions had the smallest effect of 65% (n = 47; CI 0.89–3.09; p value: < 0.001). Crops were irrigated on three soils in the field experiments using pivot irrigation. Olton loam had a negative effect of − 5% (n = 24; CI 0.33–2.73; p value: 0.927) while Pullman clay loam and Pullman silty clay loam had increases in WUE of 84% (n = 196; CI 1.35–2.50; p value: < 0.001) and 250% (n = 15; CI 1.89–6.49; p value: < 0.001). Crops planted early had a greater change in effects compared to crops planted on time, with changes of 118% (n = 128; CI 1.43–3.33; p value: < 0.001) and 87% (n = 107; CI 1.32–2.63; p value: < 0.001), respectively. Low and medium nitrogen application amounts had similar effects with a 95% (n = 53; CI 1.13–3.36; p value: 0.017) and 82% (n = 100; CI 1.24–2.68; p value: 0.002) change relative to the rainfed control. High amounts of nitrogen had a 133% (n = 82; CI 1.42–3.85; p value: 0.001) change. Water use efficiency generally improved over time as studies planted from 2010 to 2015 had a greater water response than studies planted between 1990 and 1995. The largest change in WUE was 264% (n = 9; CI 1.66–8.00; p value: 0.001) from studies with experiments planted the most recently. Studies with experiments planted between 2000 and 2005 had the second largest change of 157% (n = 52; CI 1.44–4.58; p value: 0.001). Studies planted between 2005 and 2010 had an unexpectedly small change, potentially resulting from observations primarily in the low irrigation category.
Subsurface drip irrigation
SDI accounted for 40 of the total observations. Results on the effects for SDI are presented in Fig. 4. Crops planted under SDI were corn, cotton, and sorghum. Effects for WUE between the irrigation treatments and the dryland control in corn and sorghum were quite large with a 260% change (n = 10: CI p value: 0.036) and 341% change (n = 12; CI 1–19.56; p value: 0.051), respectively. Results for cotton showed a much smaller effect with a 63% change (n = 16; CI 0.67–3.98; p value: 0.285) in WUE. The WUE response to irrigation was equally large for the low and medium irrigation application categories with a 138% change (n = 15; CI 0.85–6.70; p value: 0.01) and 153% (n = 25; CI 1.11–5.79; p value: 0.027) change, respectively. The addition of rainfall with SDI had the biggest change in dry years of 223% (n = 12; CI 0.85–12.29; p value: 0.085) and comparable effects between average and wet rainfall years with changes of 127% (n = 22; CI 1.00–5.14; p value: 0.050) and 134% (n = 6; CI 0.43–12.69; p value: 0.324). The low rainfall category had the largest change in effect size, but also had the largest confidence interval, which could be due to the low number of observations. Field experiments were conducted on two soil types. Abilene clay loam soils had a 41% (n = 12; CI 0.51–3.84; p value: 0.507) in WUE compared to a 268% (n = 28; CI 1.59–8.54; p value: 0.002) change in WUE on Pullman clay loam soils. The effects for Pullman clay loam were similar when comparing center pivot to SDI. Early planting increased WUE by 285% (n = 15; CI 1.17–12.63; p value: 0.026) compared to a 106% (n = 25; CI 0.95–4.43; p value: 0.066) change with crops planted on time. Low amounts of nitrogen application had a much greater response on WUE with a change of 282% (n = 17; CI 1.37–10.62; p value: 0.010). Medium nitrogen application had a change on WUE of 86% (n = 23; CI 0.81–4.27; p value: 0.145). The low nitrogen category had 10 corn, three sorghum, and four cotton observations, whereas the medium nitrogen category had nine sorghum, two corn, and 12 cotton observations. Studies published from 1990 to 1995 and 2000 to 2005 had similar effects with changes of 260% (n = 12; CI 1.09–11.88; p value: 0.036) and 277% (n = 16; CI 1.15–12.32; p value: 0.0287), respectively. One article by Howell et al. (1997) had observations planted from 1990 to 1995 and two articles (Colaizzi et al. 2013, 2003) had observations planted between 2000 and 2005. Studies with experiments planted more recently between 2010 and 2015 had a 41% (n = 12; CI 0.51–3.84; p value: 0.507) change on WUE. The observations in this period came from one paper written by Attia et al. (2015) that focused on cotton.
Publication bias
Visual inspection of the funnel plot indicated the presence of publication bias; however, Egger’s regression intercept was 0.025 with a two-tailed p value of 0.684. Duval and Tweedie’s Trim and Fill method indicated 48 studies were missing, which would shift the summary effect size from 0.549 to 0.656. Rosenberg’s classic fail-safe N had a Z value of 4.99 with a p value < 0.05. The number of missing studies needed to bring the p value > alpha was 1931, suggesting the estimated effects are robust.
Discussion
The overall meta-analysis results (Fig. 1) could have been impacted by publication bias and did not consistently produce clear differences among moderator variables, owing likely to the restricted choice of publications causing low representation of studies in some categories. Comparisons of effects sizes for crop species and irrigation systems followed general knowledge across the literature and in practice. Among crop species, wheat had the smallest WUE gains from irrigation, which speaks to its suitability in rainfed cropping systems in the Texas High Plains, where most of winter wheat is non-irrigated (USDA NASS 2020). Low seasonal rainfall in relation to potential ET and variability of rainfall timing prevent rainfed corn production, which contributes to explaining why the effect size of WUE was the greatest for corn (Fig. 1). The contrast among irrigation systems was clear in that furrow was less efficient than center pivot and SDI. The relatively low water-application efficiency of furrow is due to greater runoff, evaporation, and deep percolation losses than of the more advanced systems (USDA NRCS, 1997). Colaizzi et al. (2013) indicated greater WUE in deficit-irrigated cotton with SDI than with LEPA or LESA. Such differences in irrigation application efficiency are important factors in calculating cost–benefit ratios for decisions on improving irrigation systems (Amosson et al. 2011).
Data on WUE from several papers in the analysis produced some outlier observations that impacted results across several moderators. Although corn had the greatest percentage change in WUE overall (Fig. 1), when analyzed by irrigation system, sorghum had the largest percentage change with center pivot (160%, Fig. 3) and SDI (341%, Fig. 4) compared to other crops. The small effect size for sorghum under furrow irrigation (Fig. 2) reduced its overall effect in Fig. 1. Colaizzi et al. (2003) evaluated sorghum during 2000–2002 by comparing SDI and LEPA, LESA and MESA center pivot nozzles. The relatively high rainfall in 2002 resulted in greater yields and large WUE effect sizes. Subsurface drip represented only 11% of the total number of observations and 7% of the sorghum observations, which came from the Colaizzi et al. (2003) study. The favorable rainfall during one year created an unusually wide confidence interval for sorghum observations in SDI (Fig. 4). The outlier observations from the Colaizzi et al. (2013) studies also resulted in a large overall effect in the 2000–2005 planted year interval (Fig. 1).
Soil type is an example of a moderator that was inconsistently represented across moderator combinations, and therefore, defies generalization. Pullman silty clay loam showed the largest change in effect size while representing 300 of the 351 observations. Experiments performed on Olton loam were reported in one paper (Bordovsky and Lyle 1996), and only for center pivot. Pullman clay loam was the only soil type studied in all three irrigation systems, showing highly significant effect sizes for center pivot and SDI.
Planting time showed positive effects on WUE across all irrigation systems (Fig. 1), but those effects were not significant when furrow irrigated (Fig. 1). The potential advantage of early planting to exploit favorable rainfall and temperatures was apparently augmented by the greater water-application efficiencies of center pivot and SDI. As with soil type and planting time, rainfall and nitrogen showed no significant effects in WUE with furrow irrigation, but did with center pivot and SDI. Again, the greater application efficiencies of the latter systems could capitalize on favorable management and growing conditions.
Conclusions
Across all observations, furrow irrigation had the smallest changes in WUE between the irrigation treatments relative to the rainfed control and no significant effect sizes. Moderators with subsurface drip irrigation systems generated greater effects than center pivot irrigation with the exception of cotton, medium irrigation, and papers published between 2010 and 2015. Pullman clay loam soils had the greatest increase in WUE compared to rainfed controls, and early planting exhibited 30% more change in WUE relative to planting on time. This quantitative summary of research provides insight into the magnitudes and variability of changes in crop WUE across typical combinations of crop choices, irrigation management, and growing conditions. As water availability declines in the High Plains of Texas, innovative water-conservation practices and greater transition to deficit irrigation, rainfed production, and alternative land uses will have to be adopted to sustain gains in WUE afforded by advanced irrigation technologies.
References
Adu MO, Yawson DO, Armah FA, Asare PA, Frimpong KA (2018) Meta-analysis of crop yields of full, deficit, and partial root-zone drying irrigation. Agric Water Manag 197:79–90
Allen RR, Musick JT (1993) Planting date, water management, and maturity length relations for irrigated grain sorghum. Transac ASAE 36(4):1123–1129
Amosson S, Almas L, Girase JR, Kenny N, Guerrero B, Vimlesh K, Marek T (2011) Economics of irrigation systems. AgriLife Extension Service Report B-6113
Ashworth AJ, Toler HD, Allen FL, Augé RM (2018) Global meta-analysis reveals agro-grassland productivity varies based on species diversity over time. PLoS ONE 13(7):e0200274. https://doi.org/10.1371/journal.pone.0200274
Attia A, Rajan N, Ritchie G, Cui S, Ibrahim A, Hays D, Xue Q, Wilborn J (2015) Yield, quality, and spectral reflectance responses of cotton under subsurface drip irrigation. Agron J 107(4):1355–1364. https://doi.org/10.2134/agronj14.0502
Bordovsky JP (2018) Common management groupings in Texas. Personal communication
Bordovsky JP, Lyle WM (1996) LEPA irrigation of grain sorghum with varying water supplies. Transac ASAE 39(6):2033–2038
Bordovsky JP, Mustian JT, Cranmer AM, Emerson CL (2011) Cotton-grain sorghum rotation under extreme deficit irrigation conditions. Appl Eng Agric 27(3):359–371
Borenstein M, Hedges L, Higgins JPT, Rothstein HR (2009) Introduction to meta-analysis. John Wiley & Sons, Ltd
Borenstein M, Hedges L, Higgins J, Rothstein H (2013) Comprehensive meta-analysis version 3. Biostat, Englewood, NJ
Colaizzi P, Schneider AD, Howell TA, Evett SR (2003) Comparison of SDI, LEPA, and spray efficiency for grain sorghum. 2003 ASAE Annual International Meeting, Las Vegas, NV
Colaizzi PD, Gowda PH, Marek TH, Porter DO (2009) Irrigation in the Texas High Plains: a brief history and potential reductions in demand. Irrig Drain 58:257–274
Colaizzi PD, Evett SR, Howell TA (2013) Comparison of SDI, LEPA, and spray irrigation performance for cotton in the North Texas High Plains. USDA ARS Report 459–474
Duval S, Tweedie R (2000) Trim and fill: a simple funnel-plot-based method of testing and adjusting for publication bias in meta-analysis. Biometrics 56:455–463
Egger M, Smith GD, Schneider M, Minder C (1997) Bias in meta-analysis detected by a simple graphical test. BMJ 315:629–634
Fan Y, Wang C, Nan Z (2018) Determining water use efficiency of wheat and cotton: a meta-regression analysis. Agric Water Manag 199:48–60
Hao B, Xue Q, Bean BW, Rooney WL (2014) Biomass production, water and nitrogen use efficiency in photoperiod-sensitive sorghum in the Texas High Plains. Biomass Bioenerg 62:108–116
Harrison F (2011) Getting started with meta-analysis. Methods Ecol Evol 2:1–10. https://doi.org/10.1111/j.2041-210X.2010.00056.x
Hedges LV, Gurevitch J, Curtis PS (1999) The meta-analysis of response ratios in experimental ecology. Ecology 80(4):1150–1156
Higgins JPT, Thompson SG, Deeks JJ, Altman DG (2003) Measuring inconsistency in meta-analysis. BMJ 327:557–560. https://doi.org/10.1136/bmj.327.7414.557
Howell TA, Yazar A, Schneider D, Dusek DA, Copeland KS (1995) Yield and water use efficiency of corn in response to LEPA irrigation. Transac ASAE 36(6):1737–1747
Howell TA, Schneider AD, Evett SR (1997) Subsurface and surface microirrigation of corn—Southern High Plains. Transac ASAE 40(3):635–641
Howell TA, Evett SR, Tolk JA, Schneider AD (2004) Evapotranspiration of full, deficit-irrigated, and dryland cotton on the Northern Texas High Plains. J Irrig Drain Eng July/August 2004:277–285
McGuire VL (2017) Water-level and recoverable water in storage changes, High Plains Aquifer, predevelopment to 2015 and 2013–2015. Groundwater and Streamflow Information Program, Scientific Investigations Report 2017–5040, U.S. Department of the Interior and U.S. Geological Survey
USDA NASS (2020) Quickstats. https://quickstats.nass.usda.gov/. Accessed 7 July 2020.
USDA NRCS (1997) Irrigation Guide. National Engineering Handbook. United States Department of Agriculture Natural Resources Conservation Service. https://directives.sc.egov.usda.gov/OpenNonWebContent. aspx?content=17837.wba. Accessed 13 July 2020
O’Shaughnessy SA, Evett SR (2010) Canopy temperature based system effectively schedules and controls center pivot irrigation of cotton. Agric Water Manag 97:1310–1316. https://doi.org/10.1016/j.agwat.2010.03.012
O’Shaughnessy SA, Evett SR, Colaizzi PD, Tolk JA, Howell TA (2014) Early and late maturing grain sorghum under variable climate conditions in the Texas High Plains. Transac Am Soc Agri Eng 57(6):1583–1594. https://doi.org/10.13031/trans.57.10424
Rosenberg MS (2005) The file-drawer problem revisited: a general weighted method for calculating fail-safe numbers in meta-analysis. Evolution 59(2):464–468
Rudnick DR, Irmak S, West C, Chavez JL, Kisekka I, Marek TH, Schneekloth JP, Mitchell-McCallister D, Sharma V, Djaman K, Aguilar J, Schipanski ME, Rogers DH, Schlegel A (2019) Deficit irrigation management of maize in the high plains aquifer region: a review. J Am Water Resour Assoc 55(1):38–55. https://doi.org/10.1111/1752-1688.12723
Sadras VO (2009) Does partial root-zone drying improve irrigation water productivity in the field? A meta-analysis. Irrig Sci 27:183–190
Scammacca N, Roberts G, Stuebing KK (2014) Meta-analysis with complex research designs: dealing with dependence from multiple measures and multiple group comparisons. Rev Educ Res 84(3):328–364
Schneider AD, Howell TA (1996) Methods, amounts, and timing of sprinkler irrigation for winter wheat. Transac Am Soc Agri Eng 40(1):137–142
Schneider AD, Howell TA (2000) Surface runoff due to LEPA and spray irrigation of a slowly permeable soil. Am Soc Agri Eng 43(5):1089–1095
Schneider AD, Howell TA (2001) Scheduling deficit wheat irrigation with data from an evapotranspiration network. Transac Am Soc Agri Eng 44(6):1617–1623
Seo S, Segarra E, Mitchell PD, Leatham DJ (2008) Irrigation technology adoption and its implication for water conservation in the Texas High Plains: a real options approach. Agri Econ 38(1):47–55
Sterne JAC, Becker BJ, Egger M (2005) The funnel plot. In: Rothstein HR, Sutton AJ, Borenstein M (eds) Publication bias in meta-analysis-prevention, assessment, and adjustments, Wiley, pp 73–98
Texas Cattle Feeders Association (TCFA) (2009) TCFA. https://www.tcfa.org/. Accessed 3 March 2020.
Texas Water Development Board (TWDB) (2019) Ogallala Aquifer. https://www.twdb.texas.gov/groundwater/aquifer/majors/ogallala.asp. Accessed 1 October 2019
Xue Q, Zhu ZZ, Musick JT, Stewart BA, Dusek DA (2006) Physiological mechanisms contributing to the increased water-use efficiency in winter wheat under deficit irrigation. J Plant Physiol 163:154–164. https://doi.org/10.1016/j.jplph.2005.04.026
Xue Q, Rudd JC, Liu S, Jessup KE, Devkota RN, Mahan JR (2014) Yield determination and water-use efficiency of wheat under water-limited conditions in the U.S Southern High Plains. Crop Sci 54:34–47. https://doi.org/10.2135/cropsci2013.02.0108
Zhang J, Jiang H, Song X, Jin J, Zhang X (2018) The responses of plant leaf CO2/H2O exchange and water use efficiency to drought: a meta-analysis. Sustainability 10(551):1–13
Acknowledgements
Funding for this research was jointly provided by the USDA ARS Ogallala Aquifer Program and USDA Project No. 2016–68007-25066, through the National Institute for Food and Agriculture’s Agriculture and Food Research Initiative, Water for Agriculture Challenge Area. Project website: http://www.ogallalawater.org/. The project, “Sustaining agriculture through adaptive management to preserve the Ogallala aquifer under a changing climate” (Ogallala Water CAP), is a regional, integrated project comprising the work of individuals from nine institutions: Colorado State University, Kansas State University, New Mexico State University, Oklahoma State University, University of Nebraska-Lincoln, Texas A&M University, Texas Tech University, West Texas A&M University, and USDA-ARS. The authors would also like to thank Jim Bordovsky for his input.
Author information
Authors and Affiliations
Corresponding author
Ethics declarations
Conflict of interest
The authors declare they have no conflict of interest.
Additional information
Publisher's Note
Springer Nature remains neutral with regard to jurisdictional claims in published maps and institutional affiliations.
Rights and permissions
About this article
Cite this article
Mitchell-McCallister, D., Cano, A. & West, C. Meta-analysis of crop water use efficiency by irrigation system in the Texas High Plains. Irrig Sci 38, 535–546 (2020). https://doi.org/10.1007/s00271-020-00696-x
Received:
Accepted:
Published:
Issue Date:
DOI: https://doi.org/10.1007/s00271-020-00696-x