Abstract
Pace-of-life syndrome (POLS) theory predicts that physiological and behavioral traits coevolve and should fall predictably along a fast-slow life history gradient. Although this theory represents an attractive theoretical framework for exploring ramifications of life history for behavior and physiology, empirical support has been mixed for this theory, and more work is required to determine how well POLS theory explains existing patterns of behavior, particularly for extremely fast or slow life histories. We investigated factors that affect adult risk exposure and nest success during nesting excursions for the Blanding’s turtle, a long-lived ectotherm. We used radio telemetry to track gravid animals to nesting areas while measuring temperature and predation risk across the study site and monitored nest success to connect behavior to current reproductive success. Turtles responded more strongly to thermal gradients than predation risk when moving to nest sites, consistent with their armored morphology and ectothermic physiology, and generally selected relatively warm microclimates during these excursions. Nests placed further from wetland edges were more successful; unexpectedly, these same areas generally had relatively high predator activity, indicating that successful nesting areas may be riskier to adults. Accordingly, turtles did not appear to select for nest sites far from wetlands in areas likely to produce successful nests, instead placing nest sites in areas where predator species richness and activity were lower. Consistent with POLS theory, our study demonstrates that long-lived organisms engage in behavioral strategies that prioritize their own survival at the expense of current reproduction.
Similar content being viewed by others
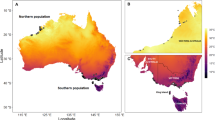
Avoid common mistakes on your manuscript.
Introduction
Pace-of-life syndrome (POLS; Wolf et al. 2007; Réale et al. 2010; Sol et al. 2018) theory has emerged in recent years to explain observed patterns of life history, physiology, and behavior in the context of fitness trade-offs. At its core, POLS theory considers how life history, behavioral, and physiological traits coevolve under species- and population-specific environmental conditions (Dammhahn et al. 2018). Under this theory, any particular pace-of-life (POL) exists along a fast-slow continuum of life history traits that reflect conflicts between maximizing survival and fecundity (Stearns 1983; Sæther 1988; Dammhahn et al. 2018; Sol et al. 2018). On one end of this continuum, fast-living strategies tend to be characterized by short life spans, increased prioritization of current reproductive output, and risk tolerance; on the other, slow-living strategies or POL generally exhibits long lifespans, increased prioritization of future over current reproductive output, and increased risk aversion (Martin et al. 2000; Wolf et al. 2007; Hau et al. 2010; Sol et al. 2018). In this way, life history characteristics, physiological traits, and behavioral tendencies should fall predictably upon a single predictable continuum or syndrome, and this syndrome could explain variation in these traits both among species (Ricklefs and Wikelski 2002) and individuals (Réale et al. 2010).
Current and future reproduction have frequently been found to a trade-off in nesting animals, such as turtles (Rivalan et al. 2005) and birds (Nilsson and Svensson 1996), making examinations of POLS in such systems a promising avenue of research. In oviparous species that construct nests, nest placement often determines offspring survival (e.g., Dunk et al. 1997; Wilson 1998; Mitchell et al. 2015). However, behaviors associated with reproduction and nesting can have substantial effects on maternal survival, as nest placement decisions may influence maternal risk (Spencer 2002). The manner in which nesting females balance this trade-off depends in large part on life history traits (Stearns 1992; Ghalambor and Martin 2001; Schwarzkopf and Andrews 2012; Refsnider et al. 2015), where long-lived species with delayed ages of maturity are generally expected to select habitats that maximize adult survival probability (Spencer 2002). Physiological and morphological traits should also influence the manner with which nesting animals respond to trade-offs; for example, ectothermic physiology places individuals in situations where they must balance predation risk and thermoregulatory behavior, which ultimately affects metabolism and growth rates (Kashon and Carlson 2018). Viewed from a POLS perspective, covariance between life history, physiological, and behavioral traits related to nesting and risk-taking should lead to tangible differences in how animals prioritize reproductive activities in any given season (Southwood 1977; Clark and Shutler 1999; Kolbe and Janzen 2002; Gaillard et al. 2010).
As long-lived organisms on the slow end of the fast-slow life history continuum, freshwater turtles represent particularly intriguing study organisms for studying costs and benefits associated with nest site selection. Nesting female turtles make terrestrial movements over several days to weeks to reach nest sites, often with strong natal philopatry and nest site fidelity (Rowe et al. 2005; Steen et al. 2012; Refsnider et al. 2015). As ectotherms, thermoregulation is important for adult survival, and overexposure of nesting females to high temperatures influences the timing and duration of turtle nesting movements (Burger and Montevecchi 1975; Spotila and Standora 1985; Spotila et al. 1997; Jessop et al. 2000). Efficient thermoregulation may also be particularly important for turtles developing eggs (Litzgus and Brooks 2000; Millar et al. 2012), as internal temperatures during gestation can influence embryo survival in reptiles (Telemeco et al. 2017). While POLS theory predicts that most turtle species should prioritize their own survival prospects over current reproductive output (Congdon et al. 1993, 1994), few studies have assessed how well physiological and behavioral traits match predictions of POLS theory during nesting excursions in turtles or other slow pace-of-life taxa.
We examined hypotheses about habitat selection and nesting choices grounded in POLS theory in Blanding’s turtle (Emydoidea blandingii), integrating information on nesting movements, locations, and success with a landscape-scale sampling of thermal conditions, predator activities, and landscape habitat conditions. Blanding’s turtles may move up to 1 km before selecting terrestrial nest sites in open patches of habitat (Steen et al. 2012) and often exhibit strong nest site fidelity (Congdon et al. 1983). Furthermore, these terrestrial nesting excursions are generally among the longest distances moved within a given season and often represent the only terrestrial excursions made by females (Ross and Anderson 1990; Rowe and Moll 1991). While hardened shells may reduce the sensitivity of turtles to predation risk, many well-armored vertebrates still respond to predation risk (if they fall on the slow end of the fast-slow life history continuum) by shifting nest placement in order to reduce adult encounters with predators (e.g., turtles in Spencer 2002) or by decreasing time spent moving through patches with higher risk (e.g., porcupines in Pokallus and Pauli 2015). Blanding’s turtles also exhibit a lower critical thermal maximum than most other aquatic turtle species (Hutchinson et al. 1966), and as such exposure to extreme temperatures in terrestrial habitats may constitute a serious risk to nesting females. Thus, Blanding’s turtles may be forced to balance factors that influence their own perception of risk to their own survival and that of their nest while navigating the landscape to reach nesting areas.
We hypothesized that females should exhibit risk aversion and select habitat to minimize stress and perception of risk during nesting excursions. Risk aversion during nesting forays may be represented spatially by avoidance of areas associated with increased predator encounters or suboptimal thermal conditions. However, as armored, slow-moving, ectothermic vertebrates, we predicted that turtles should show stronger patterns of habitat selection associated with regulating body temperature than with reducing predator encounters, as avoidance of areas with higher predator encounter probability may be unnecessary when morphological defenses can buffer against fatal predator encounters. We also hypothesized that perceived risk factors encountered during nesting movements will influence turtles’ ultimate choice of nest site. In keeping with POLS theory, we predict that individuals subjected to increased perception of risk and stress will be more likely to nest in areas that minimize additional exposure of adults to risk and stress. We also hypothesized that the combined effects of risk on the terrestrial landscape and risk avoidance behaviors that increase the safety of adult females will result in choices of nest sites that are suboptimal for nest success.
Materials and methods
Study area
Our study was conducted during the summers (nesting seasons) of 2016 and 2017 at Sandhill Wildlife Area (SWA) in Wood County, Wisconsin, which harbors a large, stable, and well-studied Blanding’s turtle population (Reid and Peery 2014; Reid et al. 2016a, 2016b, 2017). Sandhill Wildlife Area is well-suited for studies of fitness trade-offs because preferred nesting areas (restored oak savannah and other open areas) are separated from preferred aquatic habitats by forest and other unsuitable habitat, suggesting that nesting turtles may be exposed to predation risk and suboptimal thermal conditions during long-distance terrestrial nesting movements. Much of the work on nesting ecology at this site was based around three focal nesting areas towards the western, central, and northeastern portions of the site, although nests have been found along roads and near open areas throughout the area (Fig. S1a); in most cases, turtles nested in open habitats associated with loose substrates. For all work involved, it was not possible to record data blindly, because our study involved focal animals in the field.
Radio telemetry and nest location
We captured 20 turtles in early spring of 2016 using baited hoop net traps and through opportunistic encounters on roads, and 24-month radio transmitters were attached to left rear costal scutes of all captured adult females using marine-grade epoxy. A small iButton data logger (DS1921G; Maxim Integrated) was also affixed to the carapace in a similar manner to measure thermal conditions experienced by the turtle, but on right rear costal scutes instead. Before attachment, we waterproofed iButtons using a dark-colored Plasti Dip (Plasti Dip International), and set each iButton to record temperatures every 30 min. In most cases (n = 16 out of 20 originally captured in 2016), females captured during the 2016 field season were tracked again during the 2017 field season, and all female turtles processed in this way were tracked weekly until late May. Three additional animals were captured in 2017 to supplement radio telemetry efforts. Previous research on Blanding’s turtles in Wisconsin suggested that nesting usually begins early or mid-June (BNR pers. obs.). Therefore, starting in late May to early June, turtles were tracked every day to determine the onset of nesting movements. Once a turtle left the water, it was radio-tracked daily until nesting occurred, which typically takes 5–17 days (Ernst and Lovich 2009).
We attached thread spools to the rear vertebral scutes of females when they were near potential nesting grounds to identify nest locations, as nesting females typically buried thread trails when excavating nest cavities (Beaudry et al. 2010; Reid et al. 2016a). At the time of nest location, we recorded information on nest placement using a handheld GPS (to the nearest ± 5 m) and placed markers approximately 2to 3 m away from each estimated nest location. Nest depredation constitutes the single most important determinant of nest success in Blanding’s turtle (causing > 90% of observed nest failures; Congdon et al. 2000). Thus, nests were monitored daily for the first week (as Blanding’s turtle nest depredation rates are highest in the first several days after nest placement; Ernst and Lovich 2009) and then weekly for approximately 1 month to detect patterns of nest success. Nests were deemed successful if no evidence of depredation (determined based on an excavated cavity with egg shells scattered around it) was found between June and July.
Quantifying sub-lethal predation risk and thermal extremes
Females at SWA sometimes exhibit injuries consistent with predator attacks and have a higher overall incidence of injury than males at the same site (Table S1, Fig. S2), although yearly survival rates of males and females are similar (Reid et al. 2016a). While males are slightly larger than females in this species (Ernst and Lovich 2009), the differences in body size between males and females are likely too slight to lead to differences in survival. This suggests that nesting exposes females to additional risks of attack from predators and that these risks can incur costs to females during their nesting forays. Predation risk to adult turtles was measured with 33 wildlife cameras stratified across the landscape using a grid-based sampling design. The entire study area was subdivided into 500 m × 500 m cells, with cell size set to maximize spatial coverage throughout the study site given limited camera availability. If the centroid of a grid cell contained terrestrial habitat (delineated using the WISCLAND dataset; http://dnr.wi.gov/maps/gis/datalandcover.html), we placed a wildlife camera trap (Reconyx HC500 and PC800) baited with fatty acid scent (FAS) predator-survey discs within 20 m of the grid center. Camera traps remained in the field from late May to mid-July of each study year, after which storage cards were removed and photographs were examined for the presence of potential mammalian predators. We identified each predator observed to species; photographs of the same species taken within 5 min of each other were considered temporally autocorrelated and were grouped as a single detection. We quantified two metrics for each camera location: predator species richness and relative predator activity (Table 1). Given that there is considerable uncertainty about how turtles may respond to mammalian predators, we predicted that areas with increased predator species richness may appear riskier to nesting turtles due to a wider variety of cues from more species. In contrast, areas with increased predator activity would appear riskier due to an increased frequency with which cues are refreshed. Given the relatively small number of independent detections across both years (n = 79), we summarized predation risk metrics across both years. An inverse-distance weighting (IDW; Shepard 1968) approach was then used to interpolate both adult predation risk metrics between camera trapping stations. We chose to interpolate across the entire study area regardless of land cover type, as wetland and upland habitat are highly intermingled at this study site.
We measured thermal characteristics across the landscape at 28 temperature monitoring stations using the same 500 m grid used to measure predation risk. As with iButtons deployed on turtles, iButtons planned for environmental temperature measurement were coated in PlastiDip before deployment. We then generated a single random location within each grid cell and sampled that location if it contained terrestrial habitat; in this way, we monitored temperatures in terrestrial habitats likely encountered by turtles moving to nest sites and standardized our thermal monitoring system to include only terrestrial habitats simultaneously assessed for predation risk surveys. At each temperature monitoring station, we suspended a waterproofed iButton from a string approximately 9 cm above the ground to approximate shell height of an adult Blanding’s turtle (Ernst and Lovich 2009). We sampled a mix of both shaded and open areas in this way to capture variability in vegetative cover experienced by turtles.
All iButtons monitored temperatures every 30 min from late May to early July. Before processing thermal data for each year, we first examined reported minimum and maximum temperatures at local National Weather Service (NWS) monitoring stations and excluded any data logger measurements outside of the range of temperatures reported by this NWS station; generally, these observations were unrealistically warm (> 50 °C), suggesting that these measurements either represent periods of extreme solar exposure or data logger malfunction, and < 5% of available data were excluded in this way. We then trimmed thermal records for each turtle and iButton station to the same temporal duration, generally encompassing late May to late June. We extracted environmental temperature data for each time with available telemetry data and used this environmental data to create time-specific interpolated thermal layers to allow instantaneous comparison of shell temperatures with environmental temperatures (Table 1). We then summarized, for each iButton station, the proportion of each day above the 90th percentile and below the 10th percentile of observed temperatures within each study season. These daily estimates of extreme temperature exposure were then averaged throughout each study season for each iButton station. These values were then interpolated across iButton stations using the same procedure outlined for predation risk metrics to produce two metrics of temperature exposure: high temperature exposure and low temperature exposure (Table 1).
Delineating used and available habitat
Before testing hypotheses, we first processed both movement and nesting data to facilitate habitat selection analyses. For movement habitat selection analyses, we first calculated the average distance between consecutive telemetry locations for all turtles with > 5 locations in a year for both 2015 and 2016. We then used the largest of these two distances (350 m in 2016) to generate a buffer zone around each turtle location, and randomly sampled five points around each telemetry location (see Fig. S3). We consider these randomly generated points to represent habitat available to each turtle, according to a use vs. availability design (Design III in Manly et al. 2002), and assigned 1 to each used location and 0 to each available location. After data filtering, we retained 187 telemetry locations for Blanding’s turtles across 14 unique animals, which were compared with 1023 random locations across the 2 years of study.
For nest site selection analyses, we drew on a dataset of 141 nests located between 2011 and 2017. Techniques used for locating these nests are described in Reid et al. (2016a), but briefly involved searching for nesting individuals in the evening between May and July, and using thread spools to track turtles to nesting locations. Before assessing nest site selection, we first delineated the boundaries of each nesting patch using a kernel density approach with individual nests as observations. We then randomly selected 50 locations within each nesting patch to represent within-patch nesting habitat availability and assigned 1 to used locations and 0 to available locations (see Fig. S3). For each used and available location, we calculated the distance to forests, wetlands, open water, and roads (Table 1).
Analysis
To test hypothesis 1 (that female movement behavior pre-nesting was more strongly influenced by temperature than by predation risk), we evaluated multiple competing models of habitat selection using an information theoretic approach. Prior to building models, we calculated correlation coefficients between thermal and predation risk covariates, and covariates with r > |0.6| were not included simultaneously in the same model statements to reduce multicollinearity. Covariates were not correlated between categories (thermal or predation risk), but the following pairs of covariates were highly correlated: predator species richness and relative predator activity and high and low temperature exposure probabilities. We then used the function “clogit” in the package survival in R (Therneau 2015) and conditional logistic regression to evaluate a suite of a priori model statements that describe differences in temperature and predator metrics (Table 1) between used and available locations (Table S2). Conditional logistic regression allows used locations to be paired with nearby available locations and is considered more suitable for paired use-availability designs (Compton et al. 2002; Duchesne et al. 2010). Due to issues with mixed-effect model convergence, we chose to compute robust standard errors instead, which have been used to control for individual-level effects in paired regression models (Fortin et al. 2005; Forester et al. 2009; Zeller et al. 2017). We used Akaike’s Information Criterion, corrected for small sample size (AICc; Sugiura 1978; Hurvich and Tsai 1991), to evaluate models best supported by this dataset. For this, as well as analyses described below, we considered any model within 2 ΔAICc of the top model to be competitive with the top model and interpreted 95% confidence intervals for covariate estimates (Burnham and Anderson 2002). We calculated McFadden’s pseudo-R2 for each model as a metric of model fit (McFadden 1973).
To test hypothesis 2 (that female risk aversion should lead to nest placement decisions that are related to reducing the perception of risk and stress), we compared predation risk and temperatures experienced by nesting turtles before nesting between successful and unsuccessful nests and related these same movement covariates to patterns of nest placement. We first calculated predation risk and thermal covariates for each turtle with known nests (n = 26 nesting excursions across 17 individual turtles), regardless of the number of locations available for each turtle within each year. Since some turtles with known nest locations did not have available turtle-specific thermal data due to data logger loss and malfunctions, we used only indirectly-measured covariates (predator species richness and activity and high and low temperature exposure) for this analysis and summarized averaged levels of each covariate for each pre-nesting telemetry path. We then modeled the influence of a priori combinations of predator and temperature covariates on nest fate using logistic regression (Table S3). As in analyses for hypothesis 1, no models included more than one each of predation risk and exposure-based thermal covariates, and each model statement was run multiple times for each combination of predator and temperature covariates. We repeated this same model selection approach with each nest selection covariate as the response variable using linear regression; in this case, we naturally log-transformed each nest selection covariate before modeling the effects of adult experience on nest selection to improve model fit.
To test hypothesis 3 (that combined effects of risk on the terrestrial landscape and risk avoidance behaviors that increase safety of adult females will result in choices of nest sites that are suboptimal for nest success), we used the full nest success dataset (encompassing 2011–2017) to identify factors on the landscape that maximize nest success, and used a use vs. availability analysis to determine whether turtles select for factors that reduce nest mortality or adult exposure to predators when selecting nest sites. Because we aimed to link patterns of habitat selection to nest success, we also compared these same covariates between successful and unsuccessful nests (with 1 assigned to successful habitat and 0 to unsuccessful nests). For analyses of land cover effects on nest selection and nest success, correlation coefficients between covariates were calculated before model construction, and any covariates with r > |0.6| were not included in the same model statements. We then modeled the effects of a priori combinations of landscape covariates (Table S4) using unpaired logistic regression. As for hypothesis 1, we used AICc to rank models. We also explored how predator covariates influence nest selection and nest success using a similar approach to determine if turtles are actively selecting areas that either (a) reduce nest depredation, which would suggest prioritization of current reproductive output, or (b) reduce adult exposure to predators, which would suggest prioritization of future reproductive output. In order to consider if turtles select nests to reduce nest depredation, we created a separate interpolation layer that represents nest predator activity, focusing on the primary documented nest predator at this site and for this species, the raccoon (Ernst and Lovich 2009). In order to consider if turtles select nests to reduce adult exposure to predators, we used the same predator species richness and activity surfaces as for analyses of pre-nesting habitat selection, as all predator species included in this surface represent animals that turtles may perceive as a threat. Then, we modeled the effects of predation risk to nests and adults on nest selection and nest success using unpaired logistic regression and used AICc to rank models. As in analyses of hypothesis 1, we calculated McFadden’s pseudo-R2 for each nest selection and nest success model. For all analyses involving p-values, we set α = 0.05, and means are presented ± 1 SD unless otherwise noted. All data analyses were completed in R, version 3.4.3.
Results
Interpolations of predation and thermal covariates
A total of 79 independent predator detections were made between May and June across both years of study (55 in 2016 and 24 in 2017), representing seven total species: badger (Taxidea taxus), black bear (Ursus americanus), bobcat (Lynx rufus), coyote (Canis latrans), fisher (Martes pennanti), raccoon (Procyon lotor), and striped skunk (Mephitis mephitis). The most records were made for coyotes (40/79), followed by raccoons (25/79), bear (5/79), and bobcat (4/79), while the remaining three species were only detected on one or two occasions (5/79 total). Interpolated estimates of predator species richness suggested the presence of more predator species around the largest nesting patches in the western, central, and northeastern portions of the site (Fig. S1). Conversely, interpolated estimates of predator activity suggested a single hotspot of activity in several of the northeastern nesting patches, with varying levels of predator activity throughout the study area. After data filtering, a total of 36,542 turtle shell temperature measurements (21,581 in 2017 and 14,961 in 2016) and 59,481 environmental temperatures (34,045 in 2017 and 25,436 in 2016) collected from May to June 2016 and 2017 were available for interpolation and data analysis. Across all temperature measurements in both years, the average shell temperature was 24.53 ± 3.93 °C and average environmental temperature was 22.87 ± 5.58 °C. Estimates of high temperature exposure varied from 0% to 6.41%, and estimates of low temperature exposure varied from 0% to 6.63% (Fig. S1).
Predator encounter-thermal microclimate trade-offs
The top pre-nesting habitat selection model suggested that turtles were generally warmer than random locations (β = 2.248, 95% CI = 1.492 to 3.003, Fig. 1a), but avoided areas exposed to extremely high temperatures during pre-nesting movements (β = − 0.497, 95% CI − 0.838 to − 0.156, Fig. 1b). Although the interaction term between these two variables was negative, its confidence intervals overlapped with zero (β = − 0.479, 95% CI = − 1.044 to 0.087). Three models had ΔAICc < 2.0, and each model included an interaction between T and H (Table S5). Although the remaining two models with ΔAICc < 2.0 included variables related to predation risk, confidence intervals for each predation risk covariate estimate overlapped with zero.
a Pre-nesting habitat selection for time-specific temperatures, based on the top habitat selection model. Light red shading indicates 95% CIs. b Plot of the effects of high temperature exposure on pre-nesting habitat selection based on the top habitat selection model. Light red shading indicates 95% CIs, and gray points indicate observed data used in model fitting
Relationships between movement and nesting behavior
The distance nests were placed from forests was positively associated with average predator species richness experienced by turtles before nesting (β = 1.37, 95% CI = 0.49 to 2.25; Fig. 2; Table S6). No models that related movement covariates to distance of nests to wetlands, open water, or roads outperformed null models (see Table S7). No covariates related to movement habitat selection appeared to have an impact on nest success, as the null model was either the top model or within 2 AICc of the top model in each comparison (see Table S8 for a full summary of these results).
Plot of the relationship between average predator species richness (Rav) pre-nesting and natural-log-transformed nest distance to forest edges (DF), based on the top model relating pre-nesting habitat selection to nest selection. Light red shading indicates 95% CIs, and gray points indicate observed data used in model fitting
Nest selection and nest success
The most supported nest selection model suggested that turtles nest closer to forests (β = − 0.0037, 95% CI = − 0.0049 to − 0.0020; Fig. 3a) and farther from open water (β = 0.0017, 95% CI = 0.0010 to 0.0024; Fig. 3a) than expected. Although distance to wetlands and distance to roads were included in this top model, each had confidence intervals that overlapped with zero (wetlands: β = 0.0000, 95% CI = −0.0011 to 0.0013; roads: β = −0.0030, 95% CI = −0.0082 to 0.0019, Fig. 3a). This model had AICc weight = 1 and ΔAICc = 19.14 relative to the second best land cover-based nest selection model (Table S9). Selected nests were also generally in areas with less overall predator activity (β = − 0.08112, 95% CI = − 0.1563 to − 0.0242, Fig. 3a), and no support was found for the raccoon model (ΔAICc = 7.62, β = − 0.1789, 95% CI = − 0.5140 to 0.1280; Table S10).
The top land cover-based nest success model suggested that nests were more successful when laid further from wetlands (β = 0.004886, 95% CI = 0.002190 to 0.007793; Fig. 3b; Table S9). This model also indicated increasing nest success probability closer to open water; however, as 95% confidence intervals for this effect overlapped with zero (β = − 0.001043, 95% CI = − 0.002369 to 0.000231) and a model with only distance to wetlands was within 0.47 ΔAICc of the top model, we interpret this open water effect as an uninformative parameter, and interpret only the second best model with distance to wetlands as the only predictor (Arnold 2010). In this model, as in the top model, nests were more likely to survive further from wetlands (β = 0.004162, 95% CI = 0.001612 to 0.006993). These areas were also associated with more overall predator activity (β = 0.3730, 95% CI = 0.1582 to 0.6095; Fig. 3b), with no clear effects of raccoon activity on nest success (ΔAICc = 13.16, β = 0.2291, 95% CI = −0.5448 to 1.014; Table S10). Although an outlier was detected in the predator activity model, removal of this outlier did not substantially impact the predator activity covariate estimate (β = 0.3728, 95% CI = 0.1553 to 0.6094).
Discussion
According to POLS theory, an animal’s life history traits and position along a fast-slow life history continuum should lead to predictable covariance in behavioral and physiological traits (Dammhahn et al. 2018). In this study, we hypothesized three core ways in which the Blanding’s turtle’s relatively slow life history should influence behavior during nesting: (i) risk avoidance, particularly of areas that may expose the animal to increased perception of risk (due to predators) or stress (due to suboptimal thermal microclimates) during nesting excursions, and with greater avoidance of areas that expose animals to physiological stress rather than predator encounters due to morphological defenses; (ii) behavioral trade-offs between current reproduction and risk avoidance, leading to nest placement decisions that minimize adult perception of risk and stress with negative or minimal effects on nest success; and (iii) nest site preferences that do not appear to maximize current nest success.
Risk avoidance during movements
In support of our first hypothesis, animals exhibited risk avoidance when moving overland to nesting areas, but selected more strongly for habitats with optimal thermal microclimates rather than those that reduced exposure to predators. Thermally-motivated habitat selection could represent a suite of behavioral strategies to maximize both fitness components—adult survival and nest success—simultaneously. Gravid animals exhibit increased frequency and intensity of thermoregulatory behaviors (Shine 1980; Congdon 1989; Lefevre and Brooks 1995; Millar et al. 2012), and thermoregulatory behaviors appear to impact embryo survival in many species (e.g., Shine 1980; Gutzke and Packard 1987; Gutzke et al. 1987; Telemeco et al. 2017). Thus, preferences for higher temperatures overall may represent adaptations to maximize embryo survival. However, adult overexposure influences the duration of nesting excursions (Burger and Montevecchi 1975; Spotila and Standora 1985; Spotila et al. 1997; Jessop et al. 2000), and efficient thermoregulation impacts adult survival in many reptiles (Adolph and Porter 1993; Nutting and Graham 1993), suggesting that adult survival is partially dependent on accurate thermoregulation during nesting excursions. Since we did not disturb nests to determine cases of developmental mortality associated with thermal conditions experienced by nests or adult females, we were unable to determine whether patterns of thermally-motivated habitat selection in this study were more for the benefit of the mother or offspring. However, given that female thermoregulation has documented positive impacts on both embryo survival and adult survival, we interpret these risk-averse behaviors as potentially associated with both fitness components.
Although patterns of habitat selection before nesting suggested stress aversion in keeping with POLS theory, we failed to document avoidance of areas with higher predicted predator species richness or activity before nesting. On its face, this appears to run counter to POLS theory, since animals with a slow pace-of-life may generally be expected to avoid areas with more predators; however, we emphasize that morphology must be considered in tandem with life history traits to predict behavioral outcomes when exposed to predators (Royauté et al. 2018). Armored vertebrates are generally less likely to exhibit flight responses when they encounter predators, often leaning heavily on their morphological defenses to avoid harm (Stankowich and Blumstein 2005). Although some evidence suggests that armored vertebrates may exhibit avoidance of predation risk during foraging behaviors (Pokallus and Pauli 2015), the relatively abbreviated duration of nesting excursions in this study (1 to 2 weeks), slow metabolic rates of most turtles (Gibbons 1987; Avise 1992), and frequent aquatic foraging in this species (Ernst and Lovich 2009) likely mean that foraging requirements should have little effect on the behavior of nesting turtles in upland habitat. Although females did not appear to avoid areas with more predator species or activity in our study, some of the larger animals documented on our cameras (bears, bobcats, and coyotes, for example) likely could kill and consume an adult Blanding’s turtle, and predators such as raccoons have been documented to occasionally consume or attack female Blanding’s turtles (Standing et al. 1999). Thus, we caution that this lack of documented avoidance of areas with more predators before nesting may not reflect the potential for mortality or injury due to predators and acknowledge that failure to avoid areas associated with increased predation risk may instead indicate that encounter rates between predators and nesting turtles may be relatively low and unimportant for survival. Keeping in mind the relative rarity of observations of adults being consumed by predators in this species, we do interpret the lack of predator avoidance pre-nesting documented in this study as consistent with morphological defenses of turtles and suggest that morphology may produce patterns of risk-taking that may not be consistent with hypotheses based solely on life history traits.
Nest placement and current reproductive success
Nest sites that were further from wetlands had a higher probability of nest success, a finding consistent with accounts for other turtle species (Kolbe and Janzen 2002; Spencer 2002; Reid et al. 2016a). Successful nest sites were, in turn, associated with more predator activity across all species, with no clear differences in activity of the primary nest predator at this site, the raccoon, between successful and unsuccessful nests. This result does appear contradictory at first, as areas with reduced nest depredation and increased nest success may be expected to have less predator activity overall; however, much of this difference in predator activity between successful and unsuccessful nests was driven by the presence of coyotes, which do not appear to consume Blanding’s turtle nests (Ernst and Lovich 2009). This suggests that increased coyote activity around nesting areas is not due to the nests themselves, rather, habitat features that Blanding’s turtles find desirable for nesting may be simultaneously but incidentally more profitable for coyote foraging. Although coyotes have not been found to consume adult Blanding’s turtles during nesting events, attacks by a variety of other species, such as foxes and raccoons, have been documented for adult turtles, and it is likely that coyotes would be no exception (Ernst and Lovich 2009). As in other studies of turtle nest success (Kolbe and Janzen 2002; Spencer 2002), these results indicate that areas associated with successful nests are riskier for nesting adults and indicate that turtles cannot select habitats that both maximize nest success and minimize adult risk at the same time (Spencer 2002).
We failed to find evidence that nest placement maximizes current reproductive success. Turtles exhibited a preference for nest sites close to forests, with no significant preference for nest sites close to or far away from wetlands, and the suite of covariates that predicted nest success appeared to differ entirely from the suite of covariates that predicted nest placement. This was surprising, as several other studies have found that nest preferences are positively correlated with nest success (Fontaine and Martin 2006; Chalfoun and Martin 2007), nest temperature (Kolbe and Janzen 2002), and offspring phenotype (Shine and Harlow 1996; Brown and Shine 2004), and this decoupling of nest site selection and nest success suggests that factors more related to future than current reproduction may be important in driving nest placement. Indeed, although nest success was higher in areas exposed to more predators, turtles chose to nest in areas less exposed to predators. This may indicate that nest placement is more influenced by risk avoidance in adults than maximizing nest success. Nesting behaviors are often costly for many vertebrates and increase adult mortality in some birds (Martin 1993; Merilä and Wiggins 1995; Ghalambor and Martin 2001) and turtles (Spencer 2002; Refsnider et al. 2015). While armored vertebrates are often less susceptible to non-consumptive effects of predation risk (Brown and Kotler 2004), previous studies of armored species indicate that predation risk can shape movement and habitat selection behaviors (Spencer 2002; Pokallus and Pauli 2015).
Placement of nests close to forests reduced the average number of predator species encountered before nesting. While this result appears somewhat at odds with the lack of avoidance of predators during nesting movements, we interpret this result as evidence that turtles respond to risk differently before and during nesting, and that nest placement decisions can have ramifications for the overall amount of risk experienced by turtles. When moving to nesting areas, female turtles can retreat into shells to avoid predation, and thus would be unlikely to exhibit avoidance of areas with more predators before nesting; furthermore, anecdotal observations of turtles during nesting excursions indicated that animals generally hid under leaf litter and thick understory cover in forested areas when not actively moving, suggesting that forests provide opportunities for cover during nesting excursions. In contrast, animals that are actively nesting may be less capable of retreating into shells or finding cover, are in areas with unobscured lines of sight, and may be acutely concerned about exposure to predators during nesting. Spencer (2002) found that Emydura macquarii adjusted the distance of its nests from water in the presence of predators, as predators upon adults in their system were generally more common near water. Outside of reptiles, birds do appear to adjust nesting behavior in response to perceived predation risk (Eggers et al. 2006; Fontaine and Martin 2006), suggesting that female well-being is a factor that is considered when animals select nest sites, particularly for species with a relatively slow pace-of-life (Spencer 2002).
POLS theory and nesting behavior in ectotherms
Given relatively mixed support for POLS theory in existing literature (Dammhahn et al. 2018), why did we document support for POLS theory in this current study? First, key assumptions of POLS theory—trade-offs between current and future reproduction and connections between physiology/behavior and life history decisions—are well-maintained in nesting systems, particularly for turtles and other long-lived vertebrates. Nesting excursions often tangibly impact adult predation risk, and multiple studies have suggested that animals may make nest placement decisions that reduce adult mortality while increasing nest mortality, suggesting that current and future reproduction often trade-off (Ghalambor and Martin 2001; Spencer 2002). Furthermore, physiological stress is often higher in nesting animals, particularly when brood size is large (Bókony et al. 2009), and behavioral decisions related to basking do appear to differ between nesting and non-nesting animals (Millar et al. 2012). Second, we incorporated gradients of risk and temperature in our study, which allowed us to ground our system in the underlying environmental gradients presumably producing behavioral patterns (Dammhahn et al. 2018). Third, the fitness currency in this present study—nest success—is directly tied to reproductive investment and risk-taking, making it appropriate for explorations grounded in POLS theory. Fourth, we chose to study turtles; as reptiles, they have among the slowest life histories of any vertebrates, and life history may particularly constrain behavior and physiology at the extremes of the fast-slow continuum (Gibbons 1987; Nelson et al. 2002).
Nesting turtles in this study exhibited habitat selection patterns that are consistent with predictions of POLS theory, namely, that long-lived organisms with life history traits associated with a slower pace-of-life should exhibit behavioral and physiological traits that maximize future reproductive output at the expense of current reproductive success (Ghalambor and Martin 2001; Spencer 2002; Dammhahn et al. 2018). However, it should be noted that we only address POLS theory predictions that stem from the relative position of turtles along a fast-slow life history trait continuum. Recent studies have suggested that, even in long-lived species such as turtles, personality and inter-individual differences may explain differences in behavior (Ibáñez et al. 2018; Kashon and Carlson 2018), and a great deal of recent work on POLS theory has asserted that covariance between life history, physiology, and behavior should be maintained both between species and between individuals within the same taxonomic group or species (Dammhahn et al. 2018; Royauté et al. 2018). Additional studies should explore whether life history differences among species within the same taxonomic group, such as species within the freshwater turtle family Emydidae, lead to predictable differences in risk-aversion during nesting excursions or other behaviors.
Furthermore, an important consideration in studies of POLS theory is that environmental characteristics should drive covariance in life history, behavioral, and physiological traits, as these environmental characteristics should shape adaptive responses (Ricklefs and Wikelski 2002; Wikelski et al. 2003; Dammhahn et al. 2018). Thus, individuals of the same species yet occupying different sites may exhibit different behaviors due to differing environmental characteristics and adaptive pressures (Salzman et al. 2018), and future extensions of our work could explore site-level variation in risk-aversion and nesting behavior. Furthermore, our study only considered a single physiological trait (ectothermy) and only explored whether animals behaviorally select habitat in ways consistent with their ectothermic physiology. Numerous other physiological traits, such as metabolic rates, growth rates, and immune function, should also correlate with behavior and life history in long-lived species, and further work is needed to elucidate how often this is the case (Dammhahn et al. 2018; Salzman et al. 2018; Tieleman 2018).
References
Adolph SC, Porter WP (1993) Temperature, activity, and lizard life histories. Am Nat 142:273–295. https://doi.org/10.1086/285538
Arnold TW (2010) Uninformative parameters and model selection using Akaike's Information Criterion. J Wildl Manag 74:1175–1178. https://doi.org/10.1111/j.1937-2817.2010.tb01236.x
Avise JC (1992) Molecular population structure and the biogeographic history of a regional fauna: a case history with lessons for conservation biology. Oikos 63:62–76. https://doi.org/10.2307/3545516
Beaudry F, deMaynadier PG, Hunter ML Jr (2010) Nesting movements and the use of anthropogenic nesting sites by spotted turtles (Clemmys guttata) and Blanding’s turtle’s (Emydoidea blandingii). Herpetol Conserv Biol 5:1–8
Bókony V, Lendvai AZ, Liker A, Angelier F, Wingfield JC, Chastel O (2009) Stress response and the value of reproduction: are birds prudent parents? Am Nat 173:589–598. https://doi.org/10.1086/597610
Brown JS, Kotler BP (2004) Hazardous duty pay and the foraging cost of predation. Ecol Lett 7:999–1014. https://doi.org/10.1111/j.1461-0248.2004.00661.x
Brown GP, Shine R (2004) Maternal nest-site choice and offspring fitness in a tropical snake (Tropidonophis mairii, Colubridae). Ecology 85:1627–1634. https://doi.org/10.1890/03-0107
Burger J, Montevecchi WA (1975) Nest site selection in the terrapin Malaclemys terrapin. Copeia 1975:113–119. https://doi.org/10.2307/1442413
Burnham KP, Anderson DR (2002) Model selection and multimodel inference: a practical information-theoretic approach. Springer-Verlag, New York
Chalfoun AD, Martin TE (2007) Assessments of habitat preferences and quality depend on spatial scale and metrics of fitness. J Appl Ecol 44:983–992. https://doi.org/10.1111/j.1365-2664.2007.01352.x
Clark RG, Shutler D (1999) Avian habitat selection: pattern from process in nest-site use by ducks? Ecology 80:272–287. https://doi.org/10.2307/176996
Compton BW, Rhymer JM, McCollough M (2002) Habitat selection by wood turtles (Clemmys insculpta): an application of paired logistic regression. Ecology 83:833–843. https://doi.org/10.2307/3071885
Congdon JD (1989) Proximate and evolutionary constraints on energy relations of reptiles. Physiol Zool 62:356–373. https://doi.org/10.1086/physzool.62.2.30156175
Congdon JD, Tinkle DW, Breitenbach GL, van Loben Sels RC (1983) Nesting ecology and hatching success in the turtle Emydoidea blandingi. Herpetologica 39:417–429. https://doi.org/10.2307/1564063
Congdon JD, Dunham AE, van Loben Sels RC (1993) Delayed sexual maturity and demographics of Blanding’s turtles (Emydoidea blandingii): implications for conservation and management of long-lived organisms. Conserv Biol 7:826–833. https://doi.org/10.1016/0006-3207(94)90202-x
Congdon JD, Dunham AE, van Loben Sels RC (1994) Demographics of common snapping turtles (Chelydra serpentina): implications for conservation and management of long-lived organisms. Am Zool 34:397–408. https://doi.org/10.1093/icb/34.3.397
Congdon JD, Nagle RD, Kinney OM, Osentoski M, Avery H, van Loben Sels RS, Tinkle DW (2000) Nesting ecology and embryo mortality: implications for hatching success and demography of Blanding’s turtles (Emydoidea blandingii). Chelonian Conserv Bi 3:569–579
Dammhahn M, Dingemanse NJ, Niemelä PT, Réale D (2018) Pace-of-life syndromes: a framework for the adaptive integration of behaviour, physiology and life history. Behav Ecol Sociobiol 72:62. https://doi.org/10.1007/s00265-018-2473-y
Duchesne T, Fortin D, Courbin N (2010) Mixed conditional logistic regression for habitat selection studies. J Anim Ecol 79:548–555. https://doi.org/10.1111/j.1365-2656.2010.01670.x
Dunk JR, Smith RN, Cain SL (1997) Nest-site selection and reproductive success in common ravens. Auk 114:116–120. https://doi.org/10.2307/4089073
Eggers S, Griesser M, Nystrand M, Ekman J (2006) Predation risk induces changes in nest-site selection and clutch size in the Siberian jay. Proc R Soc Lond B 273:701–706. https://doi.org/10.1098/rspb.2005.3373
Ernst CH, Lovich JE (2009) Turtles of the United States and Canada, 2nd edn. JHU Press, Baltimore
Fontaine JJ, Martin TE (2006) Habitat selection responses of parents to offspring predation risk: an experimental test. Am Nat 168:811–818. https://doi.org/10.2307/4122252
Forester JD, Im HK, Rathouz PJ (2009) Accounting for animal movement in estimation of resource selection functions: sampling and data analysis. Ecology 90:3554–3565. https://doi.org/10.1890/08-0874.1
Fortin D, Beyer HL, Boyce MS, Smith DW, Duchesne T, Mao JS (2005) Wolves influence elk movements: behavior shapes a trophic cascade in Yellowstone National Park. Ecology 86:1320–1330. https://doi.org/10.1890/04-0953
Gaillard JM, Hebblewhite M, Loison A, Fuller M, Powell R, Basille M, Van Moorter B (2010) Habitat-performance relationships: finding the right metric at a given spatial scale. Philos Trans R Soc B 365:2255–2265. https://doi.org/10.1098/rstb.2010.0085
Ghalambor CK, Martin TE (2001) Fecundity-survival trade-offs and parental risk-taking in birds. Science 292:494–497. https://doi.org/10.1126/science.1059379
Gibbons JW (1987) Why do turtles live so long? BioScience 37:262–269. https://doi.org/10.2307/1310589
Gutzke WH, Packard GC (1987) The influence of temperature on eggs and hatchlings of Blanding's turtles, Emydoidea blandingii. J Herpetol 21:161–163. https://doi.org/10.2307/1564476
Gutzke WH, Packard GC, Packard MJ, Boardman TJ (1987) Influence of the hydric and thermal environments on eggs and hatchlings of painted turtles (Chrysemys picta). Herpetologica 43:393–404. https://doi.org/10.1086/physzool.59.3.30156105
Hau M, Ricklefs RE, Wikelski M, Lee KA, Brawn JD (2010) Corticosterone, testosterone and life-history strategies of birds. Proc R Soc Lond B 277:3203–3212. https://doi.org/10.1098/rspb.2010.0673
Hurvich CM, Tsai CL (1991) Bias of the corrected AIC criterion for underfitted regression and time series models. Biometrika 78:499–509. https://doi.org/10.1093/biomet/78.3.499
Hutchinson VH, Vinegar A, Kosh RJ (1966) Critical thermal maxima in turtles. Herpetologica 22:32–41
Ibáñez A, Martín J, Gazzola A, Pellitteri-Rosa D (2018) Freshwater turtles reveal personality traits in their antipredatory behaviour. Behav Process 157:142–147. https://doi.org/10.1016/j.beproc.2018.08.011
Jessop TS, Hamann M, Read MA, Limpus CJ (2000) Evidence for a hormonal tactic maximizing green turtle reproduction in response to a pervasive ecological stressor. Gen Comp Endocrinol 118:407–417. https://doi.org/10.1006/gcen.2000.7473
Kashon EAF, Carlson BE (2018) Consistently bolder turtles maintain higher body temperatures in the field but may experience greater predation risk. Behav Ecol Sociobiol 72:9. https://doi.org/10.1007/s00265-017-2428-8
Kolbe JJ, Janzen FJ (2002) Impact of nest-site selection on nest success and nest temperature in natural and disturbed habitats. Ecology 83:269–281. https://doi.org/10.2307/2680137
Lefevre K, Brooks RJ (1995) Effects of sex and body size on basking behavior in a northern population of the painted turtle, Chrysemys picta. Herpetologica 51:217–224
Litzgus JD, Brooks RJ (2000) Habitat and temperature selection of Clemmys guttata in a northern population. J Herpetol 34:178–185. https://doi.org/10.2307/1565413
Manly BFJ, McDonald LL, Thomas DL, MacDonald TL, Erickson WP (2002) Resource selection by animals: statistical design and analysis for field studies. Kluwer Academic Publisher, London
Martin TE (1993) Nest predation and nest sites. BioScience 43:523–532. https://doi.org/10.2307/1311947
Martin TE, Scott J, Menge C (2000) Nest predation increases with parental activity: separating nest site and parental activity effects. Proc R Soc Lond B 267:2287–2293. https://doi.org/10.1098/rspb.2000.1281
McFadden D (1973) Conditional logit analysis of qualitative choice behavior. In: Zarembka P (ed) Frontiers in econometrics. Academic Press, New York, pp 105–142
Merilä J, Wiggins DA (1995) Interspecific competition for nest holes causes adult mortality in the collared flycatcher. Condor 97:445–450. https://doi.org/10.2307/1369030
Millar CS, Graham JP, Blouin-Demers G (2012) The effects of sex and season on patterns of thermoregulation in Blanding’s turtles (Emydoidea blandingii) in Ontario, Canada. Chelonian Conserv Bi 11:24–32. https://doi.org/10.2744/ccb-0918.1
Mitchell TS, Maciel JA, Janzen FJ (2015) Maternal effects influence phenotypes and survival during early life stages in an aquatic turtle. Funct Ecol 29:268–276. https://doi.org/10.1111/1365-2435.12315
Nelson NJ, Keall SN, Brown D, Daugherty CH (2002) Establishing a new wild population of tuatara (Sphenodon guntheri). Conserv Biol 16:887–894. https://doi.org/10.1046/j.1523-1739.2002.00381.x
Nilsson JǺ, Svensson E (1996) The cost of reproduction: a new link between current reproductive effort and future reproductive success. Proc R Soc Lond B 263:711–714. https://doi.org/10.1098/rspb.1996.0106
Nutting WL, Graham TE (1993) Preferred body temperatures in five nearctic freshwater turtles: a preliminary study. Comp Biochem Phys A 104:243–246. https://doi.org/10.1016/0300-9629(93)90311-q
Pokallus JW, Pauli JN (2015) Predation shapes the movement of a well-defended species, the North American porcupine, even when nutritionally stressed. Behav Ecol 2015:1–6. https://doi.org/10.1093/beheco/arv176
Réale D, Garant D, Humphries MM, Bergeron P, Careau V, Montiglio PO (2010) Personality and the emergence of the pace-of-life syndrome concept at the population level. Philos Trans R Soc Lond B 365:4051–4063. https://doi.org/10.1098/rstb.2010.0208
Refsnider JM, Reedy AM, Warner DA, Janzen FJ (2015) Do trade-offs between predation pressures on females versus nests drive nest-site choice in painted turtles? Biol J Linn Soc 116:847–855. https://doi.org/10.1111/bij.12671
Reid BN, Peery MZ (2014) Land use patterns skew sex ratios, decrease genetic diversity, and trump the effects of recent climate change in an endangered turtle. Divers Distrib 20:1425–1437. https://doi.org/10.1111/ddi.12243
Reid BN, Thiel RP, Peery MZ (2016a) Population dynamics of endangered Blanding's turtles in a restored area. J Wildl Manag 80:553–562. https://doi.org/10.1002/jwmg.1024
Reid BN, Thiel RP, Palsbøll PJ, Peery MZ (2016b) Linking genetic kinship and demographic analyses to characterize dispersal: methods and application to Blanding’s turtle. J Hered 107:603–614. https://doi.org/10.1093/jhered/esw052
Reid BN, Mladenoff DJ, Peery MZ (2017) Genetic effects of landscape, habitat preference and demography on three co-occurring turtle species. Mol Ecol 26:781–798. https://doi.org/10.1111/mec.13962
Ricklefs RE, Wikelski M (2002) The physiology/life-history nexus. Trends Ecol Evol 17:462–468. https://doi.org/10.1016/s0169-5347(02)02578-8
Rivalan P, Prevot-Julliard AC, Choquet R, Pradel R, Jacquemin B, Girondot M (2005) Trade-off between current reproductive effort and delay to next reproduction in the leatherback sea turtle. Oecologia 145:564–574. https://doi.org/10.1007/s00442-005-0159-4
Ross DA, Anderson RK (1990) Habitat use, movements, and nesting of Emydoidea blandingi in Central Wisconsin. J Herpetol 24:6–12. https://doi.org/10.2307/1564283
Rowe JW, Moll EO (1991) A radiotelemetric study of activity and movements of the Blanding's turtle (Emydoidea blandingi) in northeastern Illinois. J Herpetol 25:178–185. https://doi.org/10.2307/1564646
Rowe JW, Coval KA, Dugan MR (2005) Nest placement, nest-site fidelity and nesting movements in midland painted turtles (Chrysemys picta marginata) on Beaver Island, Michigan. Am Midl Nat 154:383–397. https://doi.org/10.1674/0003-0031(2005)154[0383:npnfan]2.0.co;2
Royauté R, Berdal MA, Garrison CR, Dochtermann NA (2018) Paceless life? A meta-analysis of the pace-of-life syndrome hypothesis. Behav Ecol Sociobiol 72:64. https://doi.org/10.1007/s00265-018-2472-z
Sæther BE (1988) Pattern of covariation between life-history traits of European birds. Nature 311:616–617. https://doi.org/10.1038/331616a0
Salzman TC, McLaughlin AL, Westneat DF, Crowley PH (2018) Energetic trade-offs and feedbacks between behavior and metabolism influence correlations between pace-of-life attributes. Behav Ecol Sociobiol 72:54. https://doi.org/10.1007/s00265-018-2460-3
Schwarzkopf L, Andrews RM (2012) Are moms manipulative or just selfish? Evaluating the “maternal manipulation hypothesis” and implications for life-history studies of reptiles. Herpetologica 68:147–159. https://doi.org/10.1655/HERPETOLOGICA-D-11-00009.1
Shepard D (1968) A two-dimensional interpolation function for irregularly-spaced data. In: Proceedings of the 23rd ACM national conference. ACM, New York, pp 517–524
Shine R (1980) “Costs” of reproduction in reptiles. Oecologia 46:92–100. https://doi.org/10.1007/bf00346972
Shine R, Harlow PS (1996) Maternal manipulation of offspring phenotypes via nest-site selection in an oviparous lizard. Ecology 77:1808–1817. https://doi.org/10.2307/2265785
Sol D, Maspons J, Gonzalez-Voyer A, Morales-Castilla I, Garamszegi LZ, Møller AP (2018) Risk-taking behavior, urbanization and the pace of life in birds. Behav Ecol Sociobiol 72:59. https://doi.org/10.1007/s00265-018-2463-0
Southwood TRE (1977) Habitat, the templet for ecological strategies? J Anim Ecol 46:336–365. https://doi.org/10.2307/3817
Spencer RJ (2002) Experimentally testing nest site selection: fitness trade-offs and predation risk in turtles. Ecology 83:2136–2144. https://doi.org/10.2307/3072045
Spotila JR, Standora E (1985) Environmental constraints on the thermal energetics of sea turtles. Copeia 1985:694–702. https://doi.org/10.2307/1444763
Spotila JR, O’Connor MP, Paladino FV (1997) Thermal biology. In: Lutz PL, Musick JA (eds) The biology of sea turtles. CRC Press, Boca Raton, pp 297–314
Standing KL, Herman TB, Morrison IP (1999) Nesting ecology of Blanding's turtle (Emydoidea blandingii) in Nova Scotia, the northeastern limit of the species' range. Can J Zool 77:1609–1614. https://doi.org/10.1139/z99-122
Stankowich T, Blumstein DT (2005) Fear in animals: a meta-analysis and review of risk assessment. P Roy Soc Lond B Bio 272:2627–2634. https://doi.org/10.1098/rspb.2005.3251
Stearns SC (1983) The influence of size and phylogeny on patterns of covariation among life-history traits in the mammals. Oikos 41:173–187. https://doi.org/10.2307/3544261
Stearns SC (1992) The evolution of life histories. Oxford University Press, Oxford
Steen DA, Gibbs JP, Buhlmann KA, Carr JL, Compton BW, Congdon JD, Doody JS, Godwin JC, Holcomb KL, Jackson DR, Janzen FJ, Johnson G, Jones MT, Lamer JT, Langen TA, Plummer MV, Rowe JW, Saumure RA, Tucker JK, Wilson DS (2012) Terrestrial habitat requirements of nesting freshwater turtles. Biol Conserv 150:121–128. https://doi.org/10.1016/j.biocon.2012.03.012
Sugiura N (1978) Further analysis of the data by Akaike’s information criterion and the finite corrections. Commun Stat Theory 7:13–26
Telemeco RS, Fletcher B, Levy O, Riley A, Rodriguez-Sanchez Y, Smith C, Teague C, Waters A, Angilletta MJ, Buckley LB (2017) Lizards fail to plastically adjust nesting behavior or thermal tolerance as needed to buffer populations from climate warming. Glob Chang Biol 23:1075–1084. https://doi.org/10.1111/gcb.13476
Tieleman BI (2018) Understanding immune function as a pace of life trait requires environmental context. Behav Ecol Sociobiol 72:55. https://doi.org/10.1007/s00265-018-2464-z
Therneau T (2015) A package for survival analysis in S, version 2.38, https://CRAN.R-project.org/package=survival
Wikelski M, Spinney L, Schelsky W, Scheuerlein A, Gwinner E (2003) Slow pace of life in tropical sedentary birds: a common-garden experiment on four stonechat populations from different latitudes. Proc R Soc Lond B 270:2383–2388. https://doi.org/10.1098/rspb.2003.2500
Wilson DS (1998) Nest-site selection: microhabitat variation and its effects on the survival of turtle embryos. Ecology 79:1884–1892. https://doi.org/10.2307/176696
Wolf M, van Doorn GS, Leimar O, Weissing FJ (2007) Life-history trade-offs favour the evolution of animal personalities. Nature 447:581–584. https://doi.org/10.1038/nature05835
Zeller KA, Vickers TW, Ernest HB, Boyce WM, Boyce MS (2017) Multi-level, multi-scale resource selection functions and resistance surfaces for conservation planning: pumas as a case study. PLOS ONE 12:e0179570. https://doi.org/10.1371/journal.pone.0179570
Funding
This work was supported by a United States Department of Agriculture Hatch Act formula grant to MZP (WIS01865).
Author information
Authors and Affiliations
Corresponding author
Ethics declarations
Conflicts of interest
The authors declare that they have no conflict of interest.
Ethical approval
All applicable institutional and/or national guidelines for the care and use of animals were followed, and this work was approved by the UW-Madison Institutional Animal Care and Use Committee (IACUC #A005358) and the Wisconsin Department of Natural Resources (SRL-SOD-001-2016).
Data availability
The datasets generated during and/or analyzed during the current study are available from the corresponding author on reasonable request.
Additional information
Communicated by T. Madsen
Publisher’s note
Springer Nature remains neutral with regard to jurisdictional claims in published maps and institutional affiliations.
Electronic supplementary material
ESM 1
(DOCX 1559 kb)
Rights and permissions
About this article
Cite this article
Byer, N.W., Reid, B.N. & Peery, M.Z. Implications of slow pace-of-life for nesting behavior in an armored ectotherm. Behav Ecol Sociobiol 73, 47 (2019). https://doi.org/10.1007/s00265-019-2658-z
Received:
Revised:
Accepted:
Published:
DOI: https://doi.org/10.1007/s00265-019-2658-z