Abstract
Purpose
An imaging-based stratification tool is needed to identify melanoma patients who will benefit from anti Programmed Death-1 antibody (anti-PD1). We aimed at identifying biomarkers for survival and response evaluated in lymphoid tissue metabolism in spleen and bone marrow before initiation of therapy.
Methods
This retrospective study included 55 patients from two institutions who underwent 18F-FDG PET/CT before anti-PD1. Parameters extracted were SUVmax, SUVmean, HISUV (SUV-based Heterogeneity Index), TMTV (total metabolic tumor volume), TLG (total lesion glycolysis), BLR (Bone marrow-to-Liver SUVmax ratio), and SLR (Spleen-to-Liver SUVmax ratio). Each parameter was dichotomized using the median as a threshold. Association with survival, best overall response (BOR), and transcriptomic analyses (NanoString assay) were evaluated using Cox prediction models, Wilcoxon tests, and Spearman’s correlation, respectively.
Results
At 20.7 months median follow-up, 33 patients had responded, and 29 patients died. Median PFS and OS were 11.4 (95%CI 2.7–20.2) and 28.5 (95%CI 13.4–43.8) months. TMTV (>25cm3), SLR (>0.77), and BLR (>0.79) correlated with shorter survival. High TMTV (>25 cm3), SLR (>0.77), and BLR (>0.79) correlated with shorter survival, with TMTV (HR PFS 2.2, p = 0.02, and HR OS 2.5, p = 0.02) and BLR (HR OS 2.3, p = 0.04) remaining significant in a multivariable analysis. Low TMTV and TLG correlated with BOR (p = 0.03). Increased glucose metabolism in bone marrow (BLR) was associated with transcriptomic profiles including regulatory T cell markers (p < 0.05).
Conclusion
Low tumor burden correlates with survival and objective response while hematopoietic tissue metabolism correlates inversely with survival. These biomarkers should be further evaluated for potential clinical application.
Similar content being viewed by others
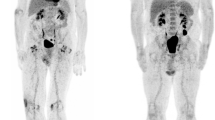
Avoid common mistakes on your manuscript.
Introduction
Immune checkpoint inhibitors (ICI) are revolutionary cancer treatments and have demonstrated remarkable efficacy and manageable toxicity with dramatic clinical results for patients with metastatic melanoma [1, 2]. ICIs specifically targeting programmed cell death 1 receptor (PD-1) have been shown to outperform standard chemotherapy in achieving clinical improvement and durability of treatment response [3]. However, despite such ICI successes, the majority of patients with metastatic melanoma will die of their disease and/or require more aggressive combination therapies. As a result, predicting patient response to standard ICIs has become an area of intensive investigation, both to avoid unnecessary toxicities and aggressive treatments in those likely to benefit from ICIs and accelerate the introduction of more potent treatments in those unlikely to respond.
Medical imaging is essential to the management of melanoma patients. In current practice, pretreatment 18F-Fluorodeoxyglucose positron emission tomography (18F-FDG PET) can stage the malignancy based on tumor extent and identify target lesions as points of reference for future assessment of ICI antitumor activity [4]. Remarkable progress in the field of computer science has transformed medical imaging by enabling automated data mining and analysis of quantitative imaging metric biomarkers extracted from standard-of-care computed tomography (CT) scans or 18F-FDG PET studies. Thus, pretreatment imaging biomarkers could become a standardized and reproducible technique for identifying appropriate candidates for ICI therapy [5].
Three main types of imaging biomarkers can be extracted from 18F-FDG PET: tumor burden, tumor glucose uptake, and nontumoral hematopoietic tissue metabolism. High anatomic and metabolic tumor burden have been proposed as metrics for identifying patients less likely to benefit from ICI in solid tumors [6] and Hodgkin’s lymphoma [7, 8].
There is strong evidence demonstrating that pretreatment anatomic tumor burden as measured on CT-scan [9, 10] and total metabolic tumor volume (TMTV) and total lesion glycolysis (TLG) as measured on 18F-FDG PET [11] are strong prognosticators of survival among patients with primary cutaneous malignant melanoma undergoing ICI treatment, as well as surgical or chemotherapeutic management [11]. Strikingly, the prognostic value of pretreatment TMTV and TLG on 18F-FDG PET remains unknown in metastatic melanoma patients treated with ICI.
An increase in tumor glucose uptake, a measurement made routinely using Maximum Standard Uptake Value (SUVmax), is typically associated with cancer aggressiveness. However, glucose consumption may also reflect an immune response directed against the tumor [12] since the measurement illuminates two cancer hallmarks [13]: deregulation of cellular energetics and tumor-promoting inflammation. SUVmax may therefore provide information about the tumor immune contextures and proinflammatory microenvironments that play key roles in determining the success or failure of ICIs [9, 13]. Moreover, tumor heterogeneity in melanoma is strongly associated with treatment failure [14, 15] and might open new routes for treatment options such as targets for ICI. Tumor heterogeneity could potentially be explored with 18F-FDG PET by the heterogeneity index of SUV (HISUV) [16, 17].
The use of imaging in oncology patients has so far focused on tumor characteristics alone to prognosticate patients’ outcomes. However, as immunotherapy has continued to prove its worth in the medical management of cancer, the study of lymphoid and hematopoietic tissues has gained traction within the research community [18]. A pro-inflammatory immune response is elicited in cancer associated lymphoid tissues such as bone marrow [19], spleen [20] modulating immune cells, in particular myeloid populations such as myeloid-derived suppressor cells (MDSCs), neutrophils [21] and macrophages [22]. Such medullary and extra-medullary hematopoiesis and cancer-related inflammation are able to be estimated on 18F-FDG PET by using bone marrow and splenic FDG uptake measurements as surrogates [23]. Given the clear implication of these tissues in oncologic progression, it was our hypothesis that extending our imaging evaluation to include bone marrow and spleen might provide an untapped source of complementary prognostic information for patients and physicians dealing with metastatic melanoma. Therefore, our study specifically aimed to address a current gap in knowledge. We investigated if pretreatment nontumoral hematopoietic tissue metabolism, as measured on 18F-FDG PET, could help identify patients who might benefit from ICI therapy.
In keeping with our ultimate aim of generating a non-invasive clinical decision-making tool, we evaluated if imaging biomarkers extracted from standard of care, pre-treatment 18F-FDG PET scans could be of value in the prediction of survival and response, allowing for early risk-stratification in patients treated with anti-PD1 ICIs for metastatic melanoma. Our study specifically assessed whether spleen and bone marrow tissue glucose metabolism correlate with patient survival independently of tumor glucose metabolism (SUVmax, HISUV), tumor burden (TMTV, TLG) and American Joint Committee on Cancer (AJCC) stratification, which takes into account the biological tumor load (LDH) and metastatic sites.
Methods
Source of data
We conducted a retrospective review of patients with metastatic melanoma who underwent an imaging protocol of 18F-FDG PET/CT, as part of phase 1 clinical trials at Gustave Roussy Comprehensive Cancer Center, Villejuif, France and as part of a standard of care evaluation at New-York Presbyterian/Columbia University Medical Center (NYP/CUMC), New York, USA. Imaging data were gathered from January 2012 to September 2017. This study was HIPAA compliant with a waiver of informed consent and was approved by the Institutional Review Board for both institutions (IRB AAAR8150).
Participants
Out of 81 patients screened, 55 were included. Figure 1 summarizes patient inclusion and exclusion criteria. Inclusion criteria were: (i) treatment with monoclonal IgG targeting PD1; (ii) biopsy-proven malignant metastatic melanoma; and (iii) availability of pre-treatment PET data. Twenty-six patients were excluded based on the following criteria (some patients met multiple exclusion criteria): (i) patients with a delay of greater than 8 weeks between pretreatment PET and first ICI infusion (n = 12); (ii) patients lost to follow-up (n = 4); (iii) patients with treatment or disease that could potentially influence metabolic activities in the spleen or bone marrow such as corticosteroids, lithium, G or GM-CSF received over the last two months, history of chronic inflammatory, auto-immune, or hematologic disease (malignant hemopathy or hemolytic anemia) or acute or chronic infection (n = 3); (iv) patients with no measurable disease or no FDG-avid tumor (n = 2), including those with brain metastases detected on MRI but not detected on 18F-FDG PET/CT (n = 4); and (v) patients with other primary malignancies (n = 1).
FDG PET/CT protocol
18F-FDG PET/CT scans were performed according to current guidelines (details in supplemental text). Two different PET/CT scanners were used: General Electric Discovery-690 (GE Healthcare, Waukesha, WI) at GRCC and Siemens PET/CT Biograph 40 LSO crystal at NYP-CUMC. Prior to 18F-FDG PET scans, patients fasted for at least 6 h and blood glucose levels were confirmed to be <180 mg/dL. Patients were injected according to current guidelines (2.5–3.5 MBq/kg). FDG (median activity 312 MBq [187–540]) and images were acquired 60 min later. A whole-body CT scan was obtained first and a whole-body PET scan was consecutively obtained using two different PET/CT scanners: General Electric Discovery-690 (GE Healthcare, Waukesha, WI) with LYSO-based detectors and Siemens PET/CT Biograph 40 LSO crystal. PET images were reconstructed using iterative algorithms. Each de-identified and anonymized patient was analyzed onsite by a pair of experienced nuclear medicine physicians who regularly perform and review 18F-FDG PET scans according to the same protocol.
Measurement of imaging biomarkers
Radiologists and nuclear medicine physicians analyzed 18F-FDG PET/CT and segmented 18F-FDG PET scans without knowledge of the historical or pathological background, imaging features, or clinical outcome of the patient subjects.
Metabolic tumor burden
All hypermetabolic metastatic lesions were selected for analysis, while hypermetabolic foci explained by inflammatory or physiologic activity were excluded. For each metastatic lesion, SUVmax, SUVmean, Metabolic Tumor Volume (MTV) and Total Lesion Glycolysis (TLG) values were measured. SUV was calculated in a pixel as (radioactivity) / (injected dose/body weight). TLG was calculated as (mean SUV) x (MTV), in which MTV was measured with setting a margin threshold as 40% of SUVmax. All values of SUVmax, MTV, and mean SUV were automatically measured by the analysis software for each lesion. A patient’s SUVmax was defined as the highest SUVmax recorded among all lesions detected in a patient. TMTV was defined as the sum of the metabolic tumor volume of all those lesions.
Nontumoral hematopoietic tissues: Bone marrow and spleen
To avoid cofounding variables, bone marrow and spleen uptake were normalized by computing the bone marrow to liver ratio (BLR) and spleen to liver ratio (SLR), respectively, by dividing the bone marrow SUVmax by the liver SUVmax and the spleen SUVmax by the liver SUVmax [24]. In bone marrow VOIs, all vertebrae with severe lumbar osteoarthritis, vertebral fractures, hemangiomas or histories of lumbar spine surgery were excluded from the analysis. Liver and spleen SUVmax values were computed by drawing a spherical VOI in the liver (3 cm-VOI/liver SUVmax) and in the spleen (2 cm-VOI/spleen SUVmax). For BM, spherical VOI with a diameter of 15 mm were placed within the center of L1 to L4 (lumbar) vertebral bodies. Bone marrow metabolic uptakes (bone marrow SUVmax) were defined as the averages of the maximum standardized uptake values of all vertebral bodies. All VOIs were drawn to exclude metastatic lesions.
Outcomes: PFS, OS and response evaluation criteria
The primary end point was to develop and validate a multivariable prediction model using pretreatment 18F-FDG PET imaging to predict overall survival (OS). OS was defined as the time from the first ICI perfusion to the date of death, due to any cause, or to the date of censoring at the last time the patient was known to be alive. The imaging interval between each consecutive CTs was approximately 12 weeks. The length of follow-up was calculated from the date of the initial PET/CT to the date of the last clinical consultation. The assessment of the outcome to be predicted was blinded.
The secondary end-point analyses were to evaluate the accuracy of pretreatment 18F-FDG PET for the prediction of progression free survival (PFS) and best overall response (BOR) classification in metastatic patients treated with anti-PD1 immunotherapy. PFS was defined as the time from first ICI perfusion to disease progression or death from any cause. Responses were evaluated with contrast-enhanced CT-scan according to the RECIST1.1 which was the reference standard when patients were evaluated.
Patients who achieved an objective partial or complete response at any time during the treatment were defined as the ‘objective response’ group. In contrast, patients who achieved stability or progression as their BOR at any time were classified as the ‘no objective response’ group.
Because melanoma is an aggressive malignancy and patients with stable disease can be assumed to benefit from immunotherapy, we also studied the predictive value of imaging biomarkers to predict disease control. Patients who achieved objective partial or complete response or stable disease at any time during the treatment were defined as patients with ‘disease controlled’ by ICI. In contrast, patients who achieved progression as their BOR at any time were classified as patients with ‘non-controlled disease’.
Association with melanoma-infiltrated lymph nodes transcriptome
In order to better understand the prognostic and predictive value of pretreatment PET biomarkers, we performed a quantitative transcriptomic analysis of 17 melanoma formalin-fixed paraffin-embedded tumor biopsies (Fig. S1). Quantitative transcriptomics analysis was evaluated using NanoString nCounter Gene Expression Assay (Institute for Systems Biology (ISB), Seattle, USA) across a representative set of 770 major genes involved in tumor immunity (Pan Cancer Immune Pathways). For each patient, 2–5 slides of ten microns were extracted with selected tumor tissue, providing sufficient material to isolate 100 ng total RNAs for an nCounter assay. Samples which had more than 30% of their fragments larger than 300 bp (as measured by the Bioanalyzer) were used for further analysis. Purification of RNAs, Nanostring analysis, and quality control were performed at Institut Curie Genomics Platform, Paris, France. Eleven predefined clusters totalling 42 genes were evaluated based on previously published methods in melanoma patients treated with immunotherapy [25]: (1) B-cells, (2) Plasma cells, (3) Monocytes/Macrophages, (4) Dendritic cells, (5) Lymphocytes, (6) Exhausted CD8+ T-cells, (7) Regulatory T-cells, (8) Cytotoxicity, (9) Exhausted CD8+ T-cells, (10) Memory T-cells, (11) Lymphocytic exhausted/cell-cycle.
Statistical analysis
Continuous variables were dichotomized at their median value. Mean values of pretreatment PET biomarkers between patients with ‘objective response’ and ‘no objective response’ group, as well as between disease controlled and non-controlled patients were compared using Wilcoxon tests. The prognostic value for survival of all pretreatment imaging biomarkers was studied with Cox models for survival. Multivariate analyses were performed using Cox proportional hazard regression models in stepwise manner for independent significant factors. Survival time was defined as the time from PET/CT to the date of death or last follow-up. Spearman’s rho coefficient was used to test the correlation between PET biomarkers and transcriptomics analysis. Transcriptomics analysis was performed and displayed using a heatmap with scaled expression values of discriminative gene sets per cluster as defined in this study [25]. A list of representative genes is shown per cluster. All reported p values were adjusted to account for the multiple comparisons issue using the Holm–Bonferroni method (adjusted p), and significant p values below 5% were considered. Analyses were performed with PASW Statistics for Windows (version 25, SPSS Inc, Chicago, USA).
Results
Patients characteristics
Pretreatment patient characteristics are summarized in Table 1. The distribution of PET parameters is described in Fig. S2. A total of 55 patients with metastatic melanoma were included as illustrated in Fig. 1. Sixty-four percent of patients (n = 35) were classified M1c according to the AJCC classification. Median age was 63 years (range, 20–85 years). After a median follow-up of 20.7 months (range, 1.0–72.6; average 25.9 months), 38 patients had disease progression or death and 29 patients died. The characteristics of the population were comparable to historical cohorts [1, 2].
Correlation between PET and clinical parameters
Significant correlations (p < 0.05) between PET biomarkers, extracted from tumor lesions and nontumoral hematopoietic tissues is displayed in Fig. S3. As expected, we found that markers of tumor burden correlate significantly with each other. We also found a weaker correlation between SLR and BLR (rank[rho] = 0.31; p < 0.05). TMTV also correlated with SLR (rank[rho] = 0.34; p < 0.05) and BLR (rank[rho] = 0.44; p < 0.05) (Fig. 2). BLR (continuous variable) was not correlated with any clinical or biological variables (Fig. S4). No clinical or biological variable was statistically significatively different in patients with high BLR as compared to patients with low BLR (Table S1).
Prognostic and theranostic imaging biomarkers: cross talk and thresholds identified. a Illustration of low and high total metabolic tumor volume using maximal intensity projection on FDG-PET images of two patients. Illustration of high spleen and bone marrow metabolism in a patient using maximal intensity projection on FDG-PET images. b Illustration of low versus high TMTV and low versus high BLR using maximal intensity projection on FDG-PET images of eight patients. These two biomarkers can be appraised visually and can be combined in a metabolic score (low-rosk, intermediate-risk, high-risk. c Kaplan-Meier curve of overall survival (OS) according to a metabolic score combining TMTV and BLR. The combined score comprising two binary risk variables was defined as follows: 0 risk variable = low-risk group (TMTV ≤ 25 cm3 AND BLR ≤ 0.79); 1 risk variable = intermediate-risk group (TMTV > 25 cm3 OR BLR > 0.79); left = low TMTV and high BLR; right = high TMTV and low BLR; 2 risk variables = high-risk group (TMTV > 25 cm3 AND BLR > 0.79)
PET biomarkers correlated with survival
Next, we tested whether the identified PET biomarkers correlate with survival in patients treated with anti-PD1.
At the time of analysis, median PFS was 11.4 months (95%CI 2.7–20.2) and 38 patients progressed or died. In univariate analysis, high TMTV (> 25cm3) and high BLR (>0.79) were significantly associated with shorter PFS (adjusted p-values = 0.021 and 0.042 respectively) (Table 2, Fig. S5). In multivariate analysis, high TMTV remained the only independent statistically significant prognostic factor for PFS (HR 2.2 p = 0.024). Although there was a trend in univariate analysis, SLR (>0.77) and TLG (>258) were not considered as statistically independent prognostic factors (adjusted p values = 0.18 and 0.23, respectively).
At the time of analysis, median OS was 28.5 (95%CI 13.4–43.8) and 29 patients died. High TMTV (adjusted p value = 0.042) and high BLR (adjusted p value = 0.048) were significantly associated with poor OS in univariate analysis (Table 2). In multivariate analysis, high TMTV and high BLR remained independent statistically significant prognostic factors for OS (HR 2.5, p = 0.022 and HR 2.3, p = 0.041, respectively) (Table 2). As with PFS, and despite a trend in univariate analysis, SLR and TLG were not considered as statistically independent prognostic factors for OS (adjusted p values = 0.24 and 0.29, respectively). Thus, in summary, high TMTV and high BLR correlate with shortened OS and PFS in univariate models and both correlate significantly with OS in a multivariate model while only TMTV correlates with PFS in a multivariate model.
Prognostic metabolic score
We developed a prognostic score combining the TMTV and BLR, which are both independent factors for OS in multivariate analysis (Fig. 2). The population was stratified in three risk categories: low-risk if TMTV≤25 cm3 and BLR ≤ 0.79 (n = 19, 34%), intermediate-risk group if TMTV>25cm3 or BLR > 0.79 (n = 18, 33%) and high-risk if TMTV>25cm3 and BLR > 0.79 (n = 18, 33%) (Fig. 2). Median OS was 52.4 months (95%CI 38.9–65.8) for the low-risk group, 36.7 months (95%CI 25.7–47.7) for the intermediate-risk group, and 13.9 months (95%CI 9.2–18.8) for high-risk group (p = 0.001).
PET biomarkers associated with immune-response
The predictive value of imaging biomarkers for BOR status was evaluated to differentiate the group of patients with ‘objective response’ (33 patients including 19 CR and 14 PR) from patients with ‘no objective response’ (22 patients including 7 SD and 15 PD). Pretreatment metabolic tumor burden imaging biomarkers such as TMTV (p = 0.033) and TLG (p = 0.026) were significantly lower in patients with objective response than otherwise (Table S2, Fig. S6). Other biomarkers gathered from tumor and hematopoietic tissue glycolytic activities were not significantly different between the two groups (p > 0.05).
Forty patients achieved disease control (19 with CR, 14 with PR and 7 with SD) and 15 patients did not (PD). Metabolic tumor burden imaging biomarkers were most predictive of disease control status (Table S2). TMTV and TLG were significantly lower in patients with disease control than progression (p = 0.003 and 0.001, respectively). Other biomarkers gathered from tumor and hematopoietic tissue glycolytic activities were not significantly different between the two groups (p > 0.05).
Correlation of PET biomarkers with transcriptomics
To evaluate the cross-talk between bone marrow derived myeloid cells and the tumor immune micro-environment, we determined whether there were any correlations between bone marrow metabolism and immune cell phenotype within the tumor immune micro-environment using NanoString genomic analysis. We compared results from NanoString analysis using previous gene clusters defined based on single cell RNAseq analysis of metastatic melanoma tumors (Fig. 3). Out of 11 clusters, increased bone marrow metabolism was significantly associated with an upregulation of genes in three clusters associated with dendritic cells phenotype, regulatory T cells phenotype, as well as memory T cells phenotype as demonstrated by Spearman’s rho correlation coefficient > 0.5 (p < 0.05). Figure 3 shows that certain immune processes were not altered and can be used as controls.
Spearman’s rho correlation coefficient between PET biomarkers and Transcriptomics analysis
Out of 11 clusters, increased bone marrow metabolism was significantly associated with an upregulation of genes in three clusters associated with dendritic cells phenotype (IL3RA, THBO), regulatory T cells phenotype (FOXP3, CD4), as well as memory T cells phenotype (LTB)
Discussion
Pre-treatment metabolic tumor burden (TMTV) and hematopoietic tissue metabolism, in particular bone marrow to liver glucose metabolism ratio (BLR), on 18F-FDG PET, have significant and independent value in prognosticating OS and PFS in metastatic melanoma patients treated with anti-PD1. These imaging metrics provide a promising new way to predict anti-PD1 efficacy and offer a novel method for better stratifying and selecting the metastatic melanoma patient candidates who would most benefit from additional therapies beyond anti-PD1. On a wider perspective, clinicians need to be aware that BLR can be appraised visually using a maximum intensity projection on standard of care 18F-FDG PET. Beyond anti-PD-1 treatments, BLR could find broader applications since we have demonstrated an association with OS as well as systemic immunosuppression, and hematopoietic cells are promising targets for a broad range of therapies.
As an extension of our previously published pretreatment CT-scan score [5], we built a metabolic score, combining the TMTV and BLR to stratify the population in three different prognostic groups. Low-risk patients (low TMTV and low BLR) encompassed 34% of the population with median OS of 52 months. This promising and innovative score provides proof of concept that should be validated in a larger, independent and prospective cohort.
Our study has demonstrated that pretreatment TMTV has value in predicting whether patients treated with anti-PD1 will respond to therapy and whether they will die of melanoma. Patients with high metabolic tumor burden (TMTV>25cm3) exhibited a poorer prognosis with shorter median PFS and OS than those with low tumor burden. Additionally, pretreatment metabolic tumor burden (TMTV and TLG) was predictive of anti-PD1 mAbs efficacy since it was higher in immune-refractory patients than in immune-responsive patients. These results generalize to ICI the prognostic and predictive value of metabolic tumor which was demonstrated in other melanoma treatment strategies [26,27,28] such as surgery, chemotherapy, and targeted therapy.
The measurement of hematopoietic tissue proposed in this work was a useful tool to predict patient outcome. High bone marrow glucose metabolism (BLR) and spleen glucose metabolism (SLR) were both associated with poor survival. However, due to the strong correlation between bone marrow and spleen glucose metabolism, only bone marrow glucose metabolism significantly and independently correlated with outcome in a multivariate analysis. We therefore confirmed in an independent cohort that melanoma patients treated with ICI with increased spleen glucose metabolism (SLR > 0.77 vs. SLR > 0.82) have poorer outcome [29]. Nonetheless, in our cohort, we did not find an association with the risk of progression [29] and we suggested that the predictive value of bone marrow glucose metabolism might outperform spleen glucose metabolism. Strikingly, bone marrow metabolism was associated with the tumor immune transcriptome. The transcriptomics analysis, performed on tumor tissues, has demonstrated that increased bone marrow metabolism was associated with an upregulation of genes associated with dendritic cells, regulatory T cells activity, as well as memory T cells phenotypes. While current research strategies in oncology are deciphering tumor ‘microradiomics’ phenotype using complex imaging biomarkers measured at a millimetric voxel level, we propose an alternative ‘macroradiomics’ approach using biomarkers deciphering global characteristics such as total tumor burden and hematopoietic tissue metabolism [30].
The finding that hematopoietic tissue metabolism correlates with negative clinical outcomes can be explained based on multiple clinical and pre-clinical studies showing that bone marrow derived cells play key roles in tumor progression, most notably in neo-vascularization and priming of the metastatic niche [31, 32]. There is indeed a strong rational suggesting that there is a cross talk between tumor immune cells, tumor cells, and bone marrow [33]. Furthermore, tumor growth in melanoma seems to play a critical role in reprogramming the host immune system by regulating hematopoiesis, which might be associated with the expansion of immunosuppressive cells such as tumor associated macrophages (TAMs), regulatory T cells (Tregs) and Myeloid-Derived Suppressor Cells (MDSCs) [34]. Bone marrow hematopoietic tissues tend to be dominated by granulocyte progenitors/precursors and are mainly stimulated by cytokines and growth factors. Some patients with advanced melanoma, before any treatment or hematopoietic growth factors, present with an increased hematopoietic metabolic state on FDG PET similar to that observed in patients treated with colony granulocyte and granulocyte-macrophage colony stimulating factors (G–CSF and GM-CSF).
We observed a correlation between bone marrow and splenic metabolism supporting the hypothesis of a pathophysiologic mechanism and common systemic inflammatory state/milieu. The spleen is also a recently identified unique extramedullary reservoir of myeloid cells, which can play a significant role in the inflammatory response that follows acute injury [20]. Chronic tumor-associated inflammation initiates drastic MDSCs expansion from bone marrow and spleen [35]. As an example, MDSCs represent less than 3% of splenocytes in healthy mice compared to nearly 20% in tumor-bearing mice. Further studies could explore the correlation between bone marrow and spleen glycolytic activities features on FDG-PET, TAN and TAM density within the tumor and serum levels of circulating MDSCs and G-CSF expressed by tumor cells.
In addition to potentially helping guide patient categorization and treatment, FDG-PET may also offer insight into the biology and pathophysiology of advanced melanoma and its susceptibility to ICI treatment thanks to a growing number of non-invasive immune-diagnostic approaches [36]. Indeed, the link between cancer, inflammation and immunosuppression is well-recognized [37], as well as tumor-host immune interactions in melanoma [38, 39]. Accumulation of inflammatory factors leads to immunosuppression which is associated with cancer progression. Prolonged exposure to pro-inflammatory cytokines and hematopoietic stimulating factors may potentially result in the induction of C-reactive protein synthesis, which is considered a prognostic marker in advanced melanoma [40]. Because only a fraction of patients experience long-term benefit of ICI, additional investigations, including imaging, are needed to explore immune suppressive features that may be occurring in the tumor microenvironment and lymphoid or hematopoietic organs [41].
Surprisingly, in our cohort, pretreatment tumor glucose metabolism, appraised using SUVmax, did not serve as a prognostic biomarker. In daily clinical routine, the SUVmax is used as a reference for subsequent response evaluation, such as in the PERCIST criteria, and is considered as a surrogate of tumor aggressiveness. Higher SUVmax is associated with tumor cell proliferation and poor prognosis [42]. Our results contrast with recent studies on non-small cell lung cancer and gastric cancer, which have revealed a relationship between tumor SUVmax and the expression of tumor-related immunity markers, suggesting a potential role for 18F-FDG PET in characterizing the tumor microenvironment and selecting patient candidates for ICI [43]. Similarly, the heterogeneity index of SUV (HISUV), which is a marker that is simple to measure, describing one aspect of global intra-tumor heterogeneity [16], was not a prognostic biomarker; however, more advanced radiomics approaches using texture analysis could be investigated.
On a wider perspective, other pilot studies showed that the outcome of metastatic melanoma patients treated with anti-CTLA-4 ICI could be predicted with 18F-FDG PET imaging biomarkers deciphering the characteristics of non-melanoma-involved organs. First, a low physiologic colonic FDG uptake that is a surrogate of the gut microbiota was associated with higher clinical benefit through a T cell-mediated immune response [44]. Second, the behavior of benign lymphoid tissues revealed a relationship between the appearance of mediastinal and hilar lymph nodes (“sarcoid-like” lymphadenopathy) and response to treatment, unlike splenic uptake/appearance, which was not significantly different between responders and refractory patients [18].
The main limitations of this study include its retrospective design. The cohort was heterogeneous, perhaps including patients with inherently different prognostic factors independent of metabolic tumor burden and FDG uptake in the BM. We also acquired images on two different PET devices which could influence the measurement of PET features. However, the estimation of spleen and bone marrow uptakes was assessed by standardizing values with liver background, allowing for the harmonization of PET features and potential generalizability of our model to different centers. Finally, we could not consider immune RECIST (iRECIST) as an endpoint for PFS since it would have introduced a chronological bias. The RECIST working group developed iRECIST (i.e., a modified version of RECIST version 1.1) criteria in 2017 while our data was gathered from January 2012 to September 2017 [45]. Patients allocated earlier to anti-PD1 treatment are therefore expected to have a lower rate of pseudoprogression due to a lower rate of evaluation by CT-scans beyond progression than participants who are recruited more recently. However, this did not alter our primary endpoint (OS) and recent studies demonstrated an excellent agreement between RECIST and iRECIST [46].
Our study provides new perspective on the immunological discussion on melanoma and offers a springboard to exciting, new research opportunities for the future. Based on our results, a potential approach for novel therapies going forward may be to block immune cells in the inflammatory microenvironment, such as MDSCs [47]. This approach may possibly be used to potentiate immune checkpoint inhibitors [33]. Our innovative methodology might furthermore be an interesting tool to evaluate medullary and extra-medullary hematopoiesis triggering tumor-induced immune suppression. For instance, pre-immunotherapy 18F-FDG PET that explores BM and metabolic tumor burden might be a relevant assay to predict response to MDSCs-blockade therapies, in combination with ICI [48]. In the near future, we plan to explore immune changes in hematopoietic organs induced by ICI therapy, using 18F-FDG PET imaging.
As a conclusion, since tumor biopsy-directed clinical trials suggest that a single pretreatment biomarker cannot reliably predict outcome of melanoma patients treated with anti-Programmed Death-1 antibody (anti-PD1), we used 18F-FDG PET biomarkers to improve pretreatment risk stratification. Metabolic tumor burden (TMTV) and hematopoietic tissue metabolism, in particular bone marrow to liver glucose metabolism ratio (BLR), had significant and independent value in prognosticating survival. These imaging metrics provide a promising new way to predict anti-PD1 efficacy and offer a novel method for stratifying and selecting the metastatic melanoma patient candidates who would most benefit from anti-PD1. Low-risk patients (low TMTV and low BLR) encompassed 34% of the population with median overall survival of 52 months. Additionally, increased BLR was associated with transcriptomic profiles including regulatory T cell markers. TMTV and BLR could provide clinicians an early indication of treatment success or failure using standard of care 18F-FDG PET scans.
Abbreviations
- 18F-FDG:
-
18fluor-fluoro-deoxy-glucose
- AJCC:
-
American joint committee on cancer
- BLR:
-
Bone marrow-to-liver maximum standard uptake value ratio
- BM:
-
Bone marrow
- BOR:
-
Best overall response
- CD(4/8):
-
Cluster of differentiation
- CI:
-
Confidence interval
- CR:
-
Complete response
- CT:
-
Computed tomography
- FOXP3:
-
Forkhead box P3
- G-CSF:
-
Granulocyte-colony stimulating factor
- GM-CSF:
-
Granulocyte-macrophage colony stimulating factor.
- HISUV:
-
Heterogeneity index standard uptake value-based
- HR:
-
Hazard ratio
- ICI:
-
Immune checkpoint inhibitor
- IgG:
-
Immunoglobulin G
- IL3RA:
-
Interleukin 3 receptor subunit alpha
- iRECIST:
-
Immune response evaluation criteria in solid tumors
- LDH:
-
Lactate dehydrogenase
- LSO:
-
Lu2SiO5:Ce (lutetium, orthosilicate, cerium)
- LYSO:
-
Lu1.8Y.2SiO5:Ce (lutetium, yttrium, orthosilicate, cerium)
- MDSC:
-
Myeloid-derived suppressor cell
- MRI:
-
Magnetic resonance imaging
- OS:
-
Overall survival
- PET:
-
Positron emission tomography
- PD:
-
Progression disease
- PD-1:
-
Programmed cell death-1
- PERCIST:
-
Positron emission tomography evaluation response criteria in solid tumors
- PFS:
-
Progression-free survival
- PR:
-
Partial response
- RECIST 1.1:
-
Response evaluation criteria in solid tumors version 1.1
- RNA:
-
Ribonucleic acid
- SD:
-
Stable disease
- SLR:
-
Spleen-to-liver maximum standard uptake value ratio
- SUVmax:
-
Maximum standard uptake value
- SUVmean:
-
Mean standard uptake value
- TAM:
-
Tumor-associated macrophage
- TAN:
-
Tumor-associated neutrophil
- TLG:
-
Total lesion glycolysis
- TMTV:
-
Total metabolic tumor volume
- Tregs:
-
Regulatory T cells
- VOI:
-
Volume of interest
References
Robert C, Long GV, Brady B, Dutriaux C, Maio M, Mortier L, et al. Nivolumab in previously untreated melanoma without BRAF mutation. N Engl J Med. 2015;372:320–30.
Robert C, Schachter J, Long GV, Arance A, Grob JJ, Mortier L, et al. Pembrolizumab versus ipilimumab in advanced melanoma. N Engl J Med. 2015;372:2521–32.
Ribas A, Hamid O, Daud A, Hodi FS, Wolchok JD, Kefford R, et al. Association of Pembrolizumab with tumor response and survival among patients with advanced melanoma. JAMA. 2016;315:1600–9.
Tan AC, Emmett L, Lo S, Liu V, Kapoor R, Carlino MS, et al. FDG-PET response and outcome from anti-PD-1 therapy in metastatic melanoma. Ann Oncol. 2018;29:2115–20.
Dercle L, Ammari S, Champiat S, Massard C, Ferté C, Taihi L, et al. Rapid and objective CT scan prognostic scoring identifies metastatic patients with long-term clinical benefit on anti-PD-1/−L1 therapy. Eur J Cancer. 2016;65:33–42.
Qin B-D, Jiao X-D, Zang Y-S. Tumor mutation burden to tumor burden ratio and prediction of clinical benefit of anti-PD-1/PD-L1 immunotherapy. Med Hypotheses. 2018;116:111–3.
Dercle L, Seban R-D, Lazarovici J, Schwartz LH, Houot R, Ammari S, et al. 18F-FDG PET and CT scans detect new imaging patterns of response and progression in patients with Hodgkin lymphoma treated by anti-programmed death 1 immune checkpoint inhibitor. J Nucl Med. 2018;59:15–24.
Dercle L, Ammari S, Seban R-D, Schwartz LH, Houot R, Labaied N, et al. Kinetics and nadir of responses to immune checkpoint blockade by anti-PD1 in patients with classical Hodgkin lymphoma. Eur J Cancer. 2018;91:136–44.
Joseph RW, Elassaiss-Schaap J, Kefford RF, Hwu W-J, Wolchok JD, Joshua AM, et al. Baseline tumor size is an independent prognostic factor for overall survival in patients with melanoma treated with Pembrolizumab. Clin Cancer Res. 2018;24(20):4960–7.
Robert C, Ribas A, Hamid O, Daud A, Wolchok JD, Joshua AM, et al. Durable complete response after discontinuation of Pembrolizumab in patients with metastatic melanoma. J Clin Oncol. 2018;36:1668–74.
Son SH, Kang SM, Jeong SY, Lee S-W, Lee S-J, Lee J, et al. Prognostic value of volumetric parameters measured by pretreatment 18F FDG PET/CT in patients with cutaneous malignant melanoma. Clin Nucl Med. 2016;41:e266–73.
Tomita M, Yasui H, Higashikawa K, Nakajima K, Takakura H, Shiga T, et al. Anti PD-1 treatment increases [18F]FDG uptake by cancer cells in a mouse B16F10 melanoma model. EJNMMI Res. 2018;8:82.
Hanahan D, Weinberg RA. Hallmarks of cancer: the next generation. Cell. 2011;144:646–74.
Angelova M, Mlecnik B, Vasaturo A, Bindea G, Fredriksen T, Lafontaine L, et al. Evolution of metastases in space and time under immune selection. Cell. 2018;175:751–765.e16.
Gerlinger M, Rowan AJ, Horswell S, Math M, Larkin J, Endesfelder D, et al. Intratumor heterogeneity and branched evolution revealed by multiregion sequencing. N Engl J Med. 2012;366:883–92.
Salamon J, Derlin T, Bannas P, Busch JD, Herrmann J, Bockhorn M, et al. Evaluation of intratumoural heterogeneity on 18F-FDG PET/CT for characterization of peripheral nerve sheath tumours in neurofibromatosis type 1. Eur J Nucl Med Mol Imaging. 2013;40:685–92.
Dercle L, Hartl D, Rozenblum-Beddok L, Mokrane F-Z, Seban R-D, Yeh R, et al. Diagnostic and prognostic value of 18F-FDG PET, CT, and MRI in perineural spread of head and neck malignancies. Eur Radiol. 2018;28:1761–70.
Sachpekidis C, Larribère L, Kopp-Schneider A, Hassel JC, Dimitrakopoulou-Strauss A. Can benign lymphoid tissue changes in 18F-FDG PET/CT predict response to immunotherapy in metastatic melanoma? Cancer Immunol Immunother. 2019;68(2):297–303.
Zhao E, Xu H, Wang L, Kryczek I, Wu K, Hu Y, et al. Bone marrow and the control of immunity. Cell Mol Immunol. 2012;9:11–9.
Jordan KR, Kapoor P, Spongberg E, Tobin RP, Gao D, Borges VF, et al. Immunosuppressive myeloid-derived suppressor cells are increased in splenocytes from cancer patients. Cancer Immunol Immunother. 2017;66:503–13.
Zilio S, Serafini P. Neutrophils and granulocytic MDSC: the Janus god of cancer immunotherapy. Vaccines (Basel). 2016. https://doi.org/10.3390/vaccines4030031.
Cortez-Retamozo V, Etzrodt M, Newton A, Rauch PJ, Chudnovskiy A, Berger C, et al. Origins of tumor-associated macrophages and neutrophils. Proc Natl Acad Sci USA. 2012;109:2491–6.
Seban R-D, Robert C, Dercle L, Yeh R, Dunant A, Reuze S, et al. Increased bone marrow SUVmax on 18F-FDG PET is associated with higher pelvic treatment failure in patients with cervical cancer treated by chemoradiotherapy and brachytherapy. Oncoimmunology. 2019;8:e1574197.
Nam H-Y, Kim S-J, Kim I-J, Kim B-S, Pak K, Kim K. The clinical implication and prediction of diffuse splenic FDG uptake during cancer surveillance. Clin Nucl Med. 2010;35:759–63.
Sade-Feldman M, Yizhak K, Bjorgaard SL, Ray JP, de Boer CG, Jenkins RW, et al. Defining T cell states associated with response to checkpoint immunotherapy in melanoma. Cell. 2018;175:998–1013.e20.
Chung HW, Lee KY, Kim HJ, Kim WS, So Y. FDG PET/CT metabolic tumor volume and total lesion glycolysis predict prognosis in patients with advanced lung adenocarcinoma. J Cancer Res Clin Oncol. 2014;140:89–98.
Lee JW, Kang CM, Choi HJ, Lee WJ, Song SY, Lee J-H, et al. Prognostic value of metabolic tumor volume and total lesion glycolysis on preoperative 18F-FDG PET/CT in patients with pancreatic cancer. J Nucl Med. 2014;55:898–904.
Kang S, Ahn B-C, Hong CM, Song B-I, Lee HJ, Jeong SY, et al. Can (18)F-FDG PET/CT predict recurrence in patients with cutaneous malignant melanoma? Nuklearmedizin. 2011;50:116–21.
Wong ANM, Callahan J, Beresford J, Herschtal A, Fullerton S, Milne D, et al. Spleen to liver ratio (SLR): novel PET imaging biomarker for prediction of overall survival after ipilimumab and anti-PD1 in patients with metastatic melanoma. JCO. 2016;34:9523.
Sun R, Limkin EJ, Vakalopoulou M, Dercle L, Champiat S, Han SR, et al. A radiomics approach to assess tumour-infiltrating CD8 cells and response to anti-PD-1 or anti-PD-L1 immunotherapy: an imaging biomarker, retrospective multicohort study. Lancet Oncol. 2018;19:1180–91.
Kaplan RN, Riba RD, Zacharoulis S, Bramley AH, Vincent L, Costa C, et al. VEGFR1-positive haematopoietic bone marrow progenitors initiate the pre-metastatic niche. Nature. 2005;438:820–7.
Hiratsuka S, Nakamura K, Iwai S, Murakami M, Itoh T, Kijima H, et al. MMP9 induction by vascular endothelial growth factor receptor-1 is involved in lung-specific metastasis. Cancer Cell. 2002;2:289–300.
Morrison SJ, Scadden DT. The bone marrow niche for haematopoietic stem cells. Nature. 2014;505:327–34.
Kamran N, Li Y, Sierra M, Alghamri MS, Kadiyala P, Appelman HD, et al. Melanoma induced immunosuppression is mediated by hematopoietic dysregulation. Oncoimmunology. 2018;7:e1408750.
Gabrilovich DI, Nagaraj S. Myeloid-derived suppressor cells as regulators of the immune system. Nat Rev Immunol. 2009;9:162–74.
Bauckneht M, Piva R, Sambuceti G, Grossi F, Morbelli S. Evaluation of response to immune checkpoint inhibitors: is there a role for positron emission tomography? World J Radiol. 2017;9:27–33.
Munn LL. Cancer and inflammation. Wiley Interdiscip Rev Syst Biol Med. 2017;9(2):e1370.
Margolin K. Introduction to the role of the immune system in melanoma. Hematol Oncol Clin North Am. 2014;28:537–58.
Maru GB, Gandhi K, Ramchandani A, Kumar G. The role of inflammation in skin cancer. Adv Exp Med Biol. 2014;816:437–69.
Jiang H, Gebhardt C, Umansky L, Beckhove P, Schulze TJ, Utikal J, et al. Elevated chronic inflammatory factors and myeloid-derived suppressor cells indicate poor prognosis in advanced melanoma patients. Int J Cancer. 2015;136:2352–60.
Gebhardt C, Sevko A, Jiang H, Lichtenberger R, Reith M, Tarnanidis K, et al. Myeloid cells and related chronic inflammatory factors as novel predictive markers in melanoma treatment with Ipilimumab. Clin Cancer Res. 2015;21:5453–9.
Bastiaannet E, Hoekstra OS, de Jong JR, Brouwers AH, Suurmeijer AJH, Hoekstra HJ. Prognostic value of the standardized uptake value for (18)F-fluorodeoxyglucose in patients with stage IIIB melanoma. Eur J Nucl Med Mol Imaging. 2012;39:1592–8.
Lopci E, Toschi L, Grizzi F, Rahal D, Olivari L, Castino GF, et al. Correlation of metabolic information on FDG-PET with tissue expression of immune markers in patients with non-small cell lung cancer (NSCLC) who are candidates for upfront surgery. Eur J Nucl Med Mol Imaging. 2016;43:1954–61.
Boursi B, Werner TJ, Gholami S, Margalit O, Baruch E, Markel G, et al. Physiologic colonic fluorine-18-fluorodeoxyglucose uptake may predict response to immunotherapy in patients with metastatic melanoma. Melanoma Res. 2018.
Seymour L, Bogaerts J, Perrone A, Ford R, Schwartz LH, Mandrekar S, et al. iRECIST: guidelines for response criteria for use in trials testing immunotherapeutics. Lancet Oncol. 2017;18:e143–52.
Houdek Š, Büchler T, Kindlová E. Comparison of RECIST 1.1 and iRECIST for response evaluation in solid Tumours. Klin Onkol. 2017;30:32–9.
Shen M, Wang J, Yu W, Zhang C, Liu M, Wang K, et al. A novel MDSC-induced PD-1-PD-L1+ B-cell subset in breast tumor microenvironment possesses immuno-suppressive properties. Oncoimmunology. 2018;7:e1413520.
Liu Y, Wei G, Cheng WA, Dong Z, Sun H, Lee VY, et al. Targeting myeloid-derived suppressor cells for cancer immunotherapy. Cancer Immunol Immunother. 2018;67(8):1181–95.
Acknowledgements
Patients Recruitment (CM, JCS, LHS, YS, CR). Clinical Data Collection (SA, AMP, FZM, GR, LB, LD). Imaging Data Collection (RDS, JSN, RY, LD). Transcriptomics Data Cost (AM, YS). Transcriptomics Data Collection (AM, RG, AB, YS, LD). Data analysis (RDS, LD). Manuscript writing (RDS, LD). Manuscript editing (RDS, JSN, YS, LD). Manuscript final approval (all authors). L Dercle work is funded by a grant from Fondation Philanthropia, Geneva, Switzerland and the Fondation Nuovo-Soldati.
Author information
Authors and Affiliations
Corresponding author
Ethics declarations
Competing interests
The authors have declared no competing interest.
Additional information
Publisher’s note
Springer Nature remains neutral with regard to jurisdictional claims in published maps and institutional affiliations.
This article is part of the Topical Collection on Oncology–General.
Electronic supplementary material
ESM 1
(DOCX 344 kb)
Rights and permissions
About this article
Cite this article
Seban, RD., Nemer, J.S., Marabelle, A. et al. Prognostic and theranostic 18F-FDG PET biomarkers for anti-PD1 immunotherapy in metastatic melanoma: association with outcome and transcriptomics. Eur J Nucl Med Mol Imaging 46, 2298–2310 (2019). https://doi.org/10.1007/s00259-019-04411-7
Received:
Accepted:
Published:
Issue Date:
DOI: https://doi.org/10.1007/s00259-019-04411-7