Abstract
Osteoporosis disproportionately affects older women, yet gender differences in human osteoblasts remain unexplored. Identifying mechanisms and biomarkers of osteoporosis will enable the development of preventative and therapeutic approaches. Transcriptome data of 187 osteoblast samples from men and women were compared. Differentially expressed genes (DEGs) were identified, and weighted gene co-expression network analysis (WGCNA) was used to discover co-expressed modules. Enrichment analysis was performed to annotate DEGs. Preservation analysis determined whether modules and pathways were similar between genders. Blood methylation, transcriptome data, mouse phenotype data, and drug treatment data were utilized to identify key osteoporosis genes. We identified 1460 DEGs enriched in immune response, neurogenesis, and GWAS osteoporosis-related genes. WGCNA uncovered 8 modules associated with immune response, development, collagen metabolism, mitochondrion, and amino acid synthesis. Preservation analysis indicated modules and pathways were generally similar between genders. Incorporating GWAS and mouse phenotype data revealed 9 key genes, including GMDS, SMOC2, SASH1, MMP2, AHCYL1, ARRDC2, IGHMBP2, ATP6V1A, and CTSK. These genes were differentially methylated in patient blood and differentiated high and low bone mineral density patients in pre- and postmenopausal women. Denosumab treatment in postmenopausal women down-regulated 6 key genes, up-regulated T cell proportions, and down-regulated fibroblast proportion. qRT-PCR was used to confirm the genes in postmenopausal women. We identified 9 key osteoporosis genes by comparing the transcriptome of osteoblasts in women and men. Our findings' clinical implications were confirmed by multi-omics data and qRT-PCR, and our study provides novel biomarkers and therapeutic targets for osteoporosis diagnosis and treatment.
Similar content being viewed by others
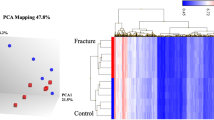
Avoid common mistakes on your manuscript.
Introduction
Osteoporosis is a debilitating disease that often leads to fractures, disability, and even mortality among the elderly population [1, 2]. While it affects both genders, postmenopausal women are particularly susceptible to osteoporosis, with a prevalence six times higher than that in men [2]. Notably, gender differences may contribute to the development of osteoporosis [3]. However, most osteoporosis studies focus on the disease in postmenopausal women, neglecting gender-specific differences in osteoblasts [4, 5].
Bone metabolism is complex and is influenced by a variety of molecules and cell types, including osteoblasts, osteoclasts, and immune cells [6,7,8]. The integrity of the human skeleton is maintained through a series of repeated bone remodeling processes, which involves the dynamic balance between bone formation mediated by osteoblasts and bone resorption mediated by osteoclasts [9]. The interplay among these factors adds to the complexity of the disease. Disorders of bone metabolism can lead to various bone-related diseases, including osteoporosis and osteoarthritis. However, the intricate mechanisms underlying osteoporosis remain largely unknown due to its complexity.
Emerging therapeutic avenues, such as T-cell therapy and anti-inflammatory treatments, offer promise in osteoporosis treatment [9]. Encouraging results have been observed in animal models with anti-inflammatory therapies [10]. Furthermore, the significance of RANKL, expressed in T cells and essential for osteoclastogenesis [6], has led to the development of denosumab. It is an anti-RANKL antibody drug approved by the U.S. Food and Drug Administration, which has shown efficacy in osteoporosis treatment [9]. Therefore, elucidating the mechanisms and biomarkers of osteoporosis may enable the development of novel therapeutic approaches.
Despite significant advances in biotechnology, such as next-generation and single-cell sequencing, a comprehensive understanding of bone-related data remains a challenge. Notably, the ongoing Genotype-Tissue Expression (GTEx) project (https://www.gtexportal.org/home/) has profiled over 50 human normal tissues but still lacks bone-related transcriptomic data [11, 12]. The Human Protein Atlas (HPA) program was launched in 2003 to map all human proteins within cells, tissues, and organs [13]. HPA has profiled over 44 human tissues but also lacks bone-related data.
In this study, we compared the transcriptome data of osteoblasts from women and men, and identified differential expression genes (DEGs). We performed a weighted gene co-expression network analysis (WGCNA) to discover co-expressed modules associated with different biological processes. To identify robust key osteoporosis genes, we intersected the DEGs with known GWAS data and mouse bone mineral density-related genes. We identified nine key genes, including GMDS, SMOC2, SASH1, MMP2, AHCYL1, ARRDC2, IGHMBP2, ATP6V1A, and CTSK. The clinical relevance of these key genes was confirmed through various multi-omics data and qRT-PCR. Furthermore, we also uncovered the potential effects of the anti-RANKL antibody, denosumab. We observed its impact on the down-regulation of six key genes, suggesting a potential modulation of critical pathways. Additionally, we found the up-regulation of T cell proportions and down-regulation of fibroblast proportion in bone biopsies, prompting further exploration of their roles in osteoporosis development. In sum, our study offers not only insights into gender disparities within osteoblast transcriptomes but also illuminates potential biomarkers and therapeutic targets for osteoporosis diagnosis and treatment.
Materials and Methods
Data Collection and Preparation
Human trabecular bone osteoblast dataset GSE15678 (Illumina humanRef-8 v2.0 expression beadchip) contains 187 samples from 95 unrelated donors [7]. The dataset was used for differential expression gene identification. Blood methylation dataset GSE163970 (Illumina HumanMethylation450 BeadChip) contains 492 samples from 232 Paget’s disease and 260 controls [14]. Blood monocytes transcriptome dataset GSE56815 (Affymetrix Human Genome U133A Array) contains 40 high (20 pre- and 20 postmenopausal) and 40 low hip bone mineral density (BMD) (20 pre- and 20 postmenopausal) subjects [15]. Diversity Outbred mice femoral transcriptome dataset GSE152708 (Illumina NextSeq 500) contains 192 femoral samples with kinds of bone traits data [16]. These datasets were used for the validation of key osteoporosis genes. Denosumab treatment dataset GSE141610 (Illumina HiSeq 2000) contains 30 bone biopsies isolated three months after treatment with a placebo (n = 15) or denosumab (n = 15) in post-menopausal women [5]. The dataset was used to infer potential drug mechanisms. All these datasets were downloaded from NCBI GEO.
Osteoporosis-related GWAS data was downloaded from GWAS Catalog using the following keywords, “osteoporosis,” “bone density,” “bone mineral content measurement,” and “bone mineral density” [17]. After redundancy removal, 2250 genes were kept for GWAS. International Mouse Phenotyping Consortium (IMPC) data were downloaded using the following keywords, “abnormal bone mineral density,” “decreased bone mineral density,” “increased bone mineral density,” “abnormal bone mineralization,” “decreased bone mineral content,” “increased bone mineral content,” and “abnormal bone mineral content” [18]. After redundancy removal, 730 genes were kept for IMPC. Gene function information was derived from the NCBI Gene database.
Transcriptome and Methylation Data Analysis
For dataset GSE15678, we used limma with the adjusted P value 0.05 as a threshold to screen as many genes as possible. Gene functional enrichment was performed by clusterProfiler and g:Profiler [19, 20]. Transcription factor motif enrichment was analyzed by the TRANSFC database [21], and only the top results were retained. For dataset GSE163970, we used the ChAMP package to analyze the M value matrix [22]. The adjusted P value for the differential methylation probe was set at 0.01. For dataset GSE141610, limma-voom was used to analyze the gene counts matrix, and the significance threshold for differential expression was set at adjusted P < 0.01 [23]. The proportion of immune cells was estimated by the TIMER tool [24].
Weighted Gene Co-expression Network Analysis for Differential Expression Genes
Weighted gene co-expression network analysis (WGCNA) was conducted according to the package manual in R4.0.3 [25]. The parameters were set as power = 14, minModuleSize = 30, network type = "signed," and deepSplit = 4. The module expression values were correlated with gender, where women were assigned a value of 1 and men a value of 0. The module and pathway preservation was calculated by the modulePreservation function in WGCNA with the following parameters, networkType = "signed," nPermutations = 100, parallelCalculation = T [26]. Preservation refers to the assessment of the similarity/conservation of modules or pathways across different datasets or conditions [26]. The preservation statistic was summarized by Zsummary value with the following thresholds: if Zsummary > 10 there is strong evidence that the module/pathway is preserved; if 2 < Zsummary < 10 there is weak to moderate evidence of preservation; if Zsummary < 2, there is no evidence that the module/pathway preserved. All the results visualization was carried out in R.
Quantitative Reverse Transcription Polymerase Chain Reaction (qRT-PCR)
Eight postmenopausal women with osteoporosis and 3 healthy women were enrolled. The femur (1 g) was collected from each patient during hip arthroplasty and stored in liquid nitrogen as soon as possible. For the qRT-PCR assay, after RNA extraction, 1 ug of RNA was used for reverse transcription to cDNA. A PCR experiment was conducted using the TB GreenTM Premix ExTaqTMII (Takara, Dalian, China) and analyzed with the real-time ABI 7500 FAST PCR system (Applied Biosystems, Foster City, USA). The primers used for the qRT-PCR were SLC35D2 (forward 5ʹ-TCCACTGGAGACCTGCAACA-3ʹ, reverse 5ʹ-GCTGTCGTCAGGGCTGAAT-3ʹ), C1D (forward 5ʹ-TTGGTCAAAATGGCAGGTGGAGAAATGAAT-3ʹ, reverse 5ʹ- TTAATGTTTACTTTTTCCTTTATTAGCAAC-3ʹ), and SMOC2 (forward 5ʹ-ATGACGACGGCACCTACAG-3ʹ, reverse 5ʹ-TCGCGTTGGGGTAACTTTTCA-3ʹ), SASH1 (forward 5ʹ-CGGTCCCAGATCGAAGAGTC-3ʹ, reverse 5ʹ-GTTCTTTCGGAAGTTCTGCCA-3ʹ), MMP2 (forward 5ʹ -TACAGGATCATTGGCTACACACC-3ʹ, reverse 5ʹ -GGTCACATCGCTCCAGACT-3ʹ), SPP1 (forward 5ʹ-ATAAGCGGAAAGCCAATGATGAGAG-3ʹ, reverse 5ʹ -TTTGGGGTCTACAACCAGCATATCT-3ʹ), KIF25 (forward 5ʹ -TGTTATGGCGTATGGACAGACG-3ʹ, reverse 5ʹ -TCAGCCACTCTAGGGATAATTCC-3ʹ), LAMA5 (forward 5ʹ -CCCACCGAGGACCTTTACTG-3ʹ, reverse 5ʹ-GGTGTGCCTTGTTGCTGTT-3ʹ), ARNT (forward 5ʹ-TAGTGCCCTGGCTCGAAAAC-3ʹ, reverse 5ʹ-GGTTCAAAACAGGAGTCACGG-3ʹ), FBXO38 (forward 5ʹ-TATGGCCTTCACCCTCGATAC-3ʹ, reverse 5ʹ-ACACCCACTAAGTTTGGGCAT-3ʹ), and AHCYL1 (forward 5ʹ-TCGCTCGATCTCACAGTCCT-3ʹ, reverse 5ʹ-TCCCGGCGTCCAAATTCTG-3ʹ). The PCR conditions were as follows: initial denaturation at 95 °C for 5 s, followed by 40 cycles of 95 °C for 30 s, 60 °C for 30 s, and a final extension step at 72 °C for 5 min. The data were analyzed using the relative quantification (ΔΔCq) method, with GAPDH expression levels used for normalization.
Statistical Analysis
The ROC curve was plotted in R with packages ROCR and pROC. Clustering and dimension reduction of DEGs were conducted by heatmap and plotMDS functions in R. The enrichment of osteoporosis-related genes in DEGs was tested by the hypergeometric distribution analysis. The correlation coefficient of variables with different units was calculated by the Spearman correlation analysis. The mean difference of cell proportions was evaluated by the two-tailed t-test.
Results
1460 Differential Expression Genes were Identified in Women Compared to Men
We analyzed the GSE15678 human osteoblast dataset and found 1460 genes with differential expression (DEGs) in women compared to men. Women and men osteoblast clusters are similar, but they do not form two distinct clusters (Fig. 1A). To visualize the samples based on DEGs, we used Multidimensional Scaling (MDS) which showed most of the samples clustered into two regions (Fig. 1B). Enrichment analysis indicated DEGs were associated with immune response and neurogenesis genes (Fig. 1C). Neurogenesis genes such as CTHRC1, SERPINF1, and CDK5RAP2 were down-regulated, while LAMA5, SPP1, and EPB41L3 were up-regulated in women osteoblasts. Sex-specific DEGs were observed, including Y-linked genes RPS4Y1, CYORF15A, NLGN4Y, EIF1AY, and JARID1D, which are significantly down-regulated in women osteoblast. In contrast, some X chromosome genes, such as HDHD1A, UTX, RPS4X, GEMIN8, and EIF2S3, are up-regulated in women (Fig. 1D). Furthermore, we found that some autosomal genes showed top changes in expression, with CTHRC1, PTGDS, AKR1C3, CTSK, and PHLDA1 down-regulated and HLA-DRA, RNASE1, MYH11, ACTG2, and LYZ up-regulated (Fig. 1D).
The differential expression of genes in women's osteoblast compared to men's in the GSE15678 dataset. A The heatmap highlights the different expression patterns of osteoblasts in women (red) and men (green). Rows represent genes and columns represent samples. B The Multidimensional Scaling (MDS) plot shows the two-dimensional space with green and red dots representing men and women, respectively. C The plot depicts the results of the functional enrichment analysis of DEGs by clusterProfiler. D The barplot displays the top 5 up-regulated and down-regulated autosomal genes and the top 5 up-regulated X-linked and down-regulated Y-linked genes in osteoblasts
Differential Genes were Enriched with Osteoporosis-Related Genes
We collected data from the NHGRI-EBI GWAS Catalog, which is a curated collection of all human genome-wide association studies, and from IMPC, a database of gene-knockout mouse phenotypes. We compared our DEGs and osteoporosis-related genes in GWAS Catalog and IMPC and found significant gene overlap between DEGs and GWAS Catalog (P < 0.01, Fig. 2A). Of the 166 overlapping genes, ossification and collagen catabolism-related genes were enriched (Fig. 2B). The nine genes shared among all three lists were GMDS, SMOC2, and SASH1 (up-regulated), MMP2, AHCYL1, ARRDC2, IGHMBP2, ATP6V1A, and CTSK (down-regulated), which were identified as key osteoporosis genes using the TRANSFC database (Fig. 2C). These genes were found to be enriched with motifs targeted by transcription factors LEF1 and ETS2, both of which were important in bone formation and mass [27, 28].
Differential genes were enriched with osteoporosis-related genes. A The intersection of three gene lists, DEG, GWAS, and IMPC. B Functional enrichment analysis of the 166 DEGs that overlapped with GWAS or IMPC gene lists using clusterProfiler. C The 166 genes were enriched with the target motif of two transcription factors, LEF1 and ETS2
Gene Co-expression Network Analysis Identified 8 Functional Modules
We used WGCNA to identify co-expressed modules for the key osteoporosis genes. The network construction parameter β was set as 14, which resulted in a scale-free network (Fig. 3A–C). We identified eight gene co-expression modules (Fig. 3D), which showed different expression patterns (Fig. 3E). We then performed module preservation analysis to determine the similarity of modules between men and women. We observed a general similarity between them (Fig. 3F). Functional analysis indicates that these modules are associated with different functions (Fig. 3G). For example, M1 is significantly associated with immune response and vesicle, and M2 is associated with development and collagen metabolism. We also analyzed the correlation of these modules with sex and found that modules M2 and M3 are down-regulated in women, while the other 6 modules, including M1, M4, M5, M6, M7, and M8, were up-regulated in women (Fig. 3H).
Gene co-expression network analysis of the 1460 differential genes identified 8 functional modules in osteoblast in dataset GSE15678. A The plot shows the network construction parameter β set at 14, resulting in a scale-free network. B The plot shows the regression R2 between log10(k) and log10(p(k)), which reached 0.94 with β set as 14, indicating a good fit. C The network mean connectivity stabilized when β was set as 14. D The dendrogram shows the identification of co-expression modules. The color bar shows the assignment of genes to different modules. E The plot illustrates the clustering of the 8 identified modules. F The dot plot shows the module preservation analysis using men as a reference, and the Zsummary statistic indicates the degree of module preservation. Zsummary > 10 indicates the module is highly preserved; 2 < Zsummary < 10 indicates weak to moderate evidence of preservation; Zsummary < 2, indicates no preservation. (G) Functional enrichment analysis of the module genes by g:Profiler. H The heatmap shows the relationship between module expression and gender by correlation analysis
Pathway Preservation Analysis Indicates the General Similarity Between Men's and Women's Osteoblast Transcriptome
We used the WGCNA modulePreservation function to analyze the similarity of pathways between men and women for the DEGs, which were distributed across different pathways. We selected eight KEGG pathways, including phagosome, osteoclast differentiation, fructose and mannose metabolism, lysosome, GnRH signaling pathway, cysteine and methionine metabolism, glycosaminoglycan biosynthesis -heparan sulfate/heparin, and mTOR signaling pathway, and analyzed only DEGs within these pathways. We employed the preservation statistic Zsummary (Z) as the primary measurement for the similarity/preservation between two pathways. Z > 10 indicates strong preservation, whereas 2 < Z < 10 indicates weak-moderate preservation and Z < 2 indicates no preservation. Our analysis revealed varying levels of pathway similarity between genders (Fig. 4A–K). Notably, pathways such as osteoclast differentiation, lysosome, and phagosome demonstrated significant similarity (Fig. 4A, J). Conversely, the GnRH signaling pathway, cysteine and methionine metabolism, glycosaminoglycan biosynthesis—heparan sulfate/heparin, and mTOR signaling pathways indicated no preservation. Gene co-expression network plots were used to further visualize the differences between these pathways for the individual genes (Fig. S1). For example, in the phagosome pathway, the gene ATP6V0E2 displayed weaker and fewer connections with other genes in women than in men (Fig. S1A). Similarly, in the osteoclast differentiation pathway, the gene SIRPA had fewer connections with other genes (Fig. S1B), while in the GnRH signaling pathway, MMP14 displayed a weaker connection with ADCY3 (Fig. S1B).
KEGG pathway-based analysis of the preservation between women and men in dataset GSE15678. A–C Three preservation statistics for all eight pathways are presented. Z > 10 indicates strong preservation, whereas 2 < Z < 10 indicates weak-moderate preservation and Z < 2 indicates no preservation. The dot labels are extracted from the first word for each pathway name. D–K The kME correlation for women and men in the 8 pathways, including phagosome, osteoclast differentiation, fructose, and mannose metabolism, lysosome, GnRH signaling pathway, cysteine, and methionine metabolism, glycosaminoglycan biosynthesis—heparan sulfate/heparin, and mTOR signaling pathway
Blood Methylation and Transcriptome Data Analysis Identified Key Osteoporosis Genes
The findings of a previous study suggest that blood has the potential to reflect the bone methylome and encompass sites linked to bone regulation [29]. We analyzed blood methylation data and identified 451 differentially methylated positions (DMPs) among our DEGs with adjusted P < 0.05, corresponding to 62 genes (Fig. 5A). Some top genes, such as RNF39 and KIF25, were identified by several probes (Fig. 5B). The CpG sites were distributed across different genomic regions, with the highest proportion in the gene body and CpG island (Fig. 5C). Functional analysis revealed enrichment of two bone abnormality-related terms in the Human Phenotype Ontology (HPO) database, including spondylolysis and delayed closure of the anterior fontanela (Fig. 5D), with genes included in HPO such as CTSK, FLNB, MMP2, RUNX2, and SMC3. Eight of the 9 key osteoporosis genes were among the 62 genes, with SASH1, SMOC2, and GMDS having the highest number of detected probes (19, 14, and 10, respectively) among the 9 key osteoporosis genes (Fig. 5E, F).
Blood methylation is changed in osteoporosis patients in dataset GSE163970. A The heatmap of the top 94 significantly changed CpG sites. B Histogram for the top 70 genes enriched by significant CpGs. The y-axis represents the number of detected methylated probes. C The distribution of methylation sites in different genome regions. D Functional enrichment analysis of the 62 genes by g:Profiler. E, F The distribution of methylation proportion of genes SMOC2 and GMDS in different genome regions
In the analysis of blood monocyte transcriptomes of pre- and postmenopausal women with low or high bone mineral density, we examined whether the 9 key genes could separate high and low BMD. Our results showed that only ATP6V1A had a moderate performance in separating subjects with high and low BMD in the two cohorts (Fig. 6A). Therefore, we conducted a transcriptome-wide analysis to identify other genes. In premenopausal women, ACAP2, FBXO38, and ARNT were the top genes with high performance (AUC > 0.85). In postmenopausal women, SLC35D2, C1D, ATP5PD, CTSS, and PFDN4 were identified as the top genes with high performance (AUC > 0.9). CTSS is an overlapping gene between the top-performance genes in both cohorts and is included in our DEG list. However, its separating performance differs in the two cohorts (Fig. 6A).
Clinical relevance of the key osteoporosis genes. A Blood monocyte genes in pre- and postmenopausal women can separate subjects with high and low bone mineral density, as shown in ROC curves for ATP6V1A and CTSS in dataset GSE56815. B Validation of key genes in Diversity Outbred (DO) mice identified the significant correlations of SMOC2, MMP2, ATP6V1A, and GMDS with bone traits in dataset GSE152708. C The cell proportion of CD4+, CD8+, and fibroblast significantly changed after denosumab treatment in human bone biopsies in dataset GSE141610
Confirm the Key Genes in Diversity Outbred (DO) Mice and Denosumab Treatment
In the Diversity Outbred (DO) mouse model [16], we found that both SMOC2 and MMP2 were positively associated with trabecular separation and negatively associated with trabecular number, while SMOC2 was up-regulated in osteoblasts isolated from female human donors. ATP6V1A and GMDS were positively associated with osteoblasts number, while ATP6V1A was down-regulated in women osteoblasts (Fig. 6B). In a human dataset of needle bone biopsies from the posterior iliac crest [5], we discovered that 6 of the key genes were up-regulated after denosumab treatment, including AHCYL1, ATP6V1A, CTSK, GMDS, IGHMBP2, and SASH1. Additionally, we identified that the proportion of CD4+ and CD8+ T cells increased, and that of fibroblast decreased after treatment in bone biopsies (Fig. 6C).
Validation of DEGs
To verify the above results, gene expression levels of DEGs were detected using qRT-PCR in femur samples from both postmenopausal osteoporotic patients and healthy subjects (Fig. 7). The results indicated that the up-regulation of SMOC2, SASH1, SPP1, and LAMA5 in femur was consistent with the results of DEGs, while the expression SLC35D2 and C1D was consistent with the results of postmenopausal women. Therefore, the independent data validated the credibility of our result.
Confirmation of DEG results via quantitative PCR was performed. Based on the overlapping genes present in the three gene lists, 11 DEGs (SLC35D2, C1D, SMOC2, SASH1, MMP2, SPP1, KIF25, LAMA5, ARNT, FBXO38, and AHCYL1) were selected for further qPCR validation. Femurs were collected from both postmenopausal osteoporosis patients (n = 8) and postmenopausal healthy subjects (n = 3). **P < 0.01, *P < 0.05
Discussion
Gender disparities in osteoporosis have been widely recognized, with a higher prevalence of osteoporosis and risk of fracture in women than in men [30]. While the current research has recognized gender differences in osteoporosis to a certain degree, deeper exploration into this topic could yield valuable new perspectives [31]. Our study for the first time analyzed the differences in gene expression in men and women osteoblasts, which play a major role in bone formation. We identified 1460 DEGs in osteoblasts and found they are enriched with known GWAS genes of bone density and osteoporosis. By incorporating all three gene lists, we identified 9 potential key genes associated with osteoporosis. Using WGCNA, we discovered functional modules associated with sex, and pathway-level analysis suggested that although similar, the individual gene behaviors are different in men and women. The clinical implications of these key genes were confirmed in blood methylation, transcriptome, DO mice, and drug treatment.
The transcriptome of human cell types has been extensively studied, however, the human osteoblast is rarely analyzed. Our study used traditional differential gene expression analysis to identify DEGs and identified several sex-related genes, indicating that the transcriptome data is valid and our DEGs workflow is correct. Sex-specific differences, including increased transcription of RPS4Y1, have been proven to be associated with osteogenic differentiation in human adult neural crest-derived stem cells [32]. DEGs were enriched with immune response and neurogenesis genes. The associations between immune imbalance, neurogenesis, and osteoporosis have been established [9, 33]. For example, neurogenesis genes CTHRC1 can positively regulate osteogenic differentiation in rat alveolar bone, and CDK5RAP2 variation can promote osteoporosis in a large cohort of postmenopausal women [34, 35]. For the first time in women's osteoblasts, we identified autosome genes related to bone diseases, despite some of these genes being previously well-studied. For example, CTHRC1 was found to be down-regulated in women's osteoblasts, while it was known to increase bone mass as a positive regulator of osteoblastic bone formation in CTHRC1 transgenic mice [36]. Similarly, CTSK, whose knockout or inhibition could promote tooth alveolar bone regeneration in CTSK knockout mice [37], was found to be down-regulated in women osteoblasts. In the plasma samples of postmenopausal women, the expression of protein lysozyme C (LYZ) was negatively related to BMD [4], while it was down-regulated in women. IGHMBP2 mutation will compromise several critical parameters of bone quantity and architecture in IGHMBP2 mutation mice [38]. We found that IGHMBP2 was down-regulated in women. MMP2, which is associated with a human genetic disorder characterized by subcutaneous nodules, arthropathy, and focal osteolysis [39], was also found to be down-regulated in women. Our study also identified other significant genes, such as AHCYL1, ARRDC2, GMDS, and SASH1, as potential novel genes associated with bone biology that have not previously been reported to be involved in bone. Overall, these results highlight the potential molecular differences in osteoblasts that may contribute to the susceptibility of women to osteoporosis.
To gain a confident list of key osteoporosis-related genes, we incorporated several datasets, including GWAS Catalog and IMPC. Our identified genes were enriched with functions such as cell differentiation, collagen metabolism, and ossification, which are vital processes in bone homeostasis. Additionally, the DEGs we identified were enriched with known osteoporosis genes from the GWAS database. This suggests that sex differences in osteoblasts may contribute to osteoporosis susceptibility in women. The common genes of the three gene lists were also found to be enriched with targets of transcription factors LEF1 and ETS2, which are known to play critical roles in bone development and osteogenesis [40, 41]. The expression of LEF1 was detected in primary cranial osteoblasts. In female mice lacking LEF1, a decrease in the number of osteoblasts and a reduction in trabecular bone mass were observed. However, these defects were not observed in male mice lacking LEF1 [40].
To further understand the complex polygenic nature of osteoporosis, we used WGCNA to analyze gene expression patterns in men's and women's osteoblasts. Functional enrichment analysis revealed that collagen metabolism genes were down-regulated in women, despite its important roles in bone resorption and formation [42]. As each module contains many genes, pathway-level analysis was performed, which was mainly based on the pathways that involve the 9 key genes. Most of the pathways have been reported to be associated with bone resorption. This includes vital processes like osteoclast differentiation, which plays a pivotal role in osteolytic bone destruction [40]. At the pathway level, we found that SIRPA has more connections with other genes in women, which cannot be revealed by module-level analysis such as WGCNA. SIRPA is an osteoclast-associated marker and is involved in MMP-9 secretion, which is vital in endochondral ossification and bone formation [43, 44].
For complex diseases like osteoporosis, gene expression is just one aspect of the system, and many factors may contribute to its development, such as gene interactions, gene modifications, cell interactions, and cell proportions. The methylation status of genes in the blood can serve as a potential indicator of systemic factors that influence gene expression across multiple tissues, including osteoblasts [29]. To validate our DEGs, we used blood methylation and transcriptome data and identified that 62 methylated genes overlapped with DEGs and were associated with bone malfunctions, indicating the potential of epigenetics in studying osteoporosis. We also identified ATP6V1A and CTSS as potential biomarkers for osteoporosis in our study. We, for the first time, found that ATP6V1A could moderately differentiate subjects with high and low BMD in both pre- and postmenopausal women. Only one study reported the differentially methylated ATP6V1A in postmenopausal women [45]. However, as the current data only contains 40 samples, future studies with larger cohorts should be carried out to confirm it. We also found that CTSS could differentiate patients but with different performances in the two cohorts, indicating possible additional different mechanisms of osteoporosis in pre- and postmenopausal women. In our DEGs, CTSK was down-regulated, while CTSS was up-regulated. Cathepsin K and S (i.e., CTSK, CTSS) are two isoforms of cysteine proteases with different functions, whose roles in bone resorption have been well-established. Osteoclast-specific CTSK deletion stimulates S1P-dependent bone formation [46]. CTSS deficiency alters the balance between adipocyte and osteoblast differentiation [47]. Our analysis provides potential biomarkers for osteoporosis, which could help doctors to treat osteoporosis patients more effectively.
To validate the identified key genes, we used DO mice and denosumab treatment datasets. We found that SMOC2 had the highest correlation with trabecular number. However, its role is still controversial as research has shown that SMOC2 knockdown inhibited osteoblastogenesis while SMOC2 overexpression inhibited osteogenic differentiation and extracellular matrix mineralization [48, 49]. For denosumab treatment in osteoporosis, we first discovered that the immune cells such as T cells are increased after treatment, while fibroblast is decreased. Only recently, the role of T cells in osteoporosis is discussed [2]. Fibroblast is the main source of FGF2 which plays a critical role in the modulation of the cartilage collagen network [50]. Our data indicate the potential pharmacological mechanism of denosumab.
One limitation of our analysis pertains to the validation datasets, where bone biopsies could encompass various cell types, including osteoblasts, osteoclasts, and bone lining cells. This diversity complicates the direct attribution of gene expression changes to a specific cellular source. As a reference indicates, osteocytes dominate the bone matrix as the most abundant cell type, constituting 90–95% of all bone cells, while osteoblasts and osteoclasts account for the remaining proportion (4–6% and 1–2%, respectively) [51]. We found that only two of the nine genes were more abundantly expressed in osteoclasts than in osteoblasts, including CTSK and ATP6V1A in datasets GSE31156 and GSE190615, which is consistent with BioGPS database showing higher expression in mouse osteoclasts [52]. Another limitation is that mouse BMD-related genes might be sex-specific. The sex-agnostic analysis of mice may miss some sex-specific genes. It is important to note that the differentially expressed genes identified in this analysis only represent a subset of those altered between the study groups. The genes reported here should be considered as strong candidate biomarkers, rather than an exhaustive list of all osteoporosis genes. Further explorations using more comprehensive analytical approaches may help uncover additional genes underlying the observed phenotypes.
In summary, our study used multi-omics data to identify key genes associated with osteoporosis, focusing on gender differences in osteoblast transcriptomes. Through module and pathway-level analyses, we identified differences in gene expression patterns between men and women. Additionally, we confirmed the clinical relevance of these key genes using blood methylation, transcriptome, and Diversity Outbred mice data. Our analysis of the denosumab treatment transcriptome also revealed its potential mechanism of action. Our findings provide promising biomarkers for osteoporosis diagnosis and treatment, shedding light on the complex mechanisms and gender differences underlying the disease.
Data Availability
The data analyzed in the study is available through the NCBI GEO database at https://www.ncbi.nlm.nih.gov/geo/.
References
Chen CY, Rao SS, Tan YJ, Luo MJ, Hu XK, Yin H, Huang J, Hu Y, Luo ZW, Liu ZZ, Wang ZX, Cao J, Liu YW, Li HM, Chen Y, Du W, Liu JH, Zhang Y, Chen TH, Liu HM, Wu B, Yue T, Wang YY, Xia K, Lei PF, Tang SY, Xie H (2019) Extracellular vesicles from human urine-derived stem cells prevent osteoporosis by transferring CTHRC1 and OPG. Bone Res 7:18
Srivastava RK, Dar HY, Mishra PK (2018) Immunoporosis: immunology of osteoporosis-role of T cells. Front Immunol 9:657
Kanis JA, Pitt FA (1992) Epidemiology of osteoporosis. Bone 13(Suppl 1):S7-15
Huang D, Wang Y, Lv J, Yan Y, Hu Y, Liu C, Zhang F, Wang J, Hao D (2020) Proteomic profiling analysis of postmenopausal osteoporosis and osteopenia identifies potential proteins associated with low bone mineral density. PeerJ 8:e9009
Weivoda MM, Chew CK, Monroe DG, Farr JN, Atkinson EJ, Geske JR, Eckhardt B, Thicke B, Ruan M, Tweed AJ, McCready LK, Rizza RA, Matveyenko A, Kassem M, Andersen TL, Vella A, Drake MT, Clarke BL, Oursler MJ, Khosla S (2020) Identification of osteoclast-osteoblast coupling factors in humans reveals links between bone and energy metabolism. Nat Commun 11:87
Zhao W, Liu Y, Cahill CM, Yang W, Rogers JT, Huang X (2009) The role of T cells in osteoporosis, an update. Int J Clin Exp Pathol 2:544–552
Laxman N, Rubin CJ, Mallmin H, Nilsson O, Pastinen T, Grundberg E, Kindmark A (2015) Global miRNA expression and correlation with mRNA levels in primary human bone cells. RNA 21:1433–1443
Li H, Wang C, Jin Y, Cai Y, Sun H, Liu M (2022) The integrative analysis of competitive endogenous RNA regulatory networks in osteoporosis. Sci Rep 12:9549
Zhang W, Dang K, Huai Y, Qian A (2020) Osteoimmunology: the regulatory roles of T lymphocytes in osteoporosis. Front Endocrinol (Lausanne) 11:465
Dar HY, Singh A, Shukla P, Anupam R, Mondal RK, Mishra PK, Srivastava RK (2018) High dietary salt intake correlates with modulated Th17-Treg cell balance resulting in enhanced bone loss and impaired bone-microarchitecture in male mice. Sci Rep 8:2503
Al-Barghouthi BM, Rosenow WT, Du KP, Heo J, Maynard R, Mesner L, Calabrese G, Nakasone A, Senwar B, Gerstenfeld L, Larner J, Ferguson V, Ackert-Bicknell C, Morgan E, Brautigan D, Farber CR (2022) Transcriptome-wide association study and eQTL colocalization identify potentially causal genes responsible for human bone mineral density GWAS associations. Elife 11:e77285
Consortium GT (2013) The genotype-tissue expression (GTEx) project. Nat Genet 45:580–585
Ponten F, Schwenk JM, Asplund A, Edqvist PH (2011) The Human Protein Atlas as a proteomic resource for biomarker discovery. J Intern Med 270:428–446
Diboun I, Wani S, Ralston SH, Albagha OM (2021) Epigenetic analysis of Paget’s disease of bone identifies differentially methylated loci that predict disease status. Elife 10:e65715
Zhou Y, Gao Y, Xu C, Shen H, Tian Q, Deng HW (2018) A novel approach for correction of crosstalk effects in pathway analysis and its application in osteoporosis research. Sci Rep 8:668
Al-Barghouthi BM, Mesner LD, Calabrese GM, Brooks D, Tommasini SM, Bouxsein ML, Horowitz MC, Rosen CJ, Nguyen K, Haddox S, Farber EA, Onengut-Gumuscu S, Pomp D, Farber CR (2021) Systems genetics in diversity outbred mice inform BMD GWAS and identify determinants of bone strength. Nat Commun 12:3408
MacArthur J, Bowler E, Cerezo M, Gil L, Hall P, Hastings E, Junkins H, McMahon A, Milano A, Morales J, Pendlington ZM, Welter D, Burdett T, Hindorff L, Flicek P, Cunningham F, Parkinson H (2017) The new NHGRI-EBI Catalog of published genome-wide association studies (GWAS Catalog). Nucleic Acids Res 45:D896–D901
Meehan TF, Conte N, West DB, Jacobsen JO, Mason J, Warren J, Chen CK, Tudose I, Relac M, Matthews P, Karp N, Santos L, Fiegel T, Ring N, Westerberg H, Greenaway S, Sneddon D, Morgan H, Codner GF, Stewart ME, Brown J, Horner N, International Mouse Phenotyping C, Haendel M, Washington N, Mungall CJ, Reynolds CL, Gallegos J, Gailus-Durner V, Sorg T, Pavlovic G, Bower LR, Moore M, Morse I, Gao X, Tocchini-Valentini GP, Obata Y, Cho SY, Seong JK, Seavitt J, Beaudet AL, Dickinson ME, Herault Y, Wurst W, de Angelis MH, Lloyd KCK, Flenniken AM, Nutter LMJ, Newbigging S, McKerlie C, Justice MJ, Murray SA, Svenson KL, Braun RE, White JK, Bradley A, Flicek P, Wells S, Skarnes WC, Adams DJ, Parkinson H, Mallon AM, Brown SDM, Smedley D (2017) Disease model discovery from 3,328 gene knockouts by The International Mouse Phenotyping Consortium. Nat Genet 49:1231–1238
Wu T, Hu E, Xu S, Chen M, Guo P, Dai Z, Feng T, Zhou L, Tang W, Zhan L, Fu X, Liu S, Bo X, Yu G (2021) clusterProfiler 4.0: a universal enrichment tool for interpreting omics data. Innovation (Camb) 2:100141
Raudvere U, Kolberg L, Kuzmin I, Arak T, Adler P, Peterson H, Vilo J (2019) g:Profiler: a web server for functional enrichment analysis and conversions of gene lists (2019 update). Nucleic Acids Res 47:W191–W198
Wingender E, Dietze P, Karas H, Knuppel R (1996) TRANSFAC: a database on transcription factors and their DNA binding sites. Nucleic Acids Res 24:238–241
Tian Y, Morris TJ, Webster AP, Yang Z, Beck S, Feber A, Teschendorff AE (2017) ChAMP: updated methylation analysis pipeline for Illumina BeadChips. Bioinformatics 33:3982–3984
Law CW, Chen Y, Shi W, Smyth GK (2014) voom: Precision weights unlock linear model analysis tools for RNA-seq read counts. Genome Biol 15:R29
Li T, Fu J, Zeng Z, Cohen D, Li J, Chen Q, Li B, Liu XS (2020) TIMER2.0 for analysis of tumor-infiltrating immune cells. Nucleic Acids Res 48:W509–W514
Langfelder P, Horvath S (2008) WGCNA: an R package for weighted correlation network analysis. BMC Bioinformatics 9:559
Langfelder P, Luo R, Oldham MC, Horvath S (2011) Is my network module preserved and reproducible? PLoS Comput Biol 7:e1001057
Raouf A, Seth A (2000) Ets transcription factors and targets in osteogenesis. Oncogene 19:6455–6463
Noh T, Gabet Y, Cogan J, Shi Y, Tank A, Sasaki T, Criswell B, Dixon A, Lee C, Tam J, Kohler T, Segev E, Kockeritz L, Woodgett J, Muller R, Chai Y, Smith E, Bab I, Frenkel B (2009) Lef1 haploinsufficient mice display a low turnover and low bone mass phenotype in a gender- and age-specific manner. PLoS ONE 4:e5438
Ebrahimi P, Luthman H, McGuigan FE, Akesson KE (2021) Epigenome-wide cross-tissue correlation of human bone and blood DNA methylation - can blood be used as a surrogate for bone? Epigenetics 16:92–105
Khosla S, Melton LJ 3rd, Riggs BL (1999) Osteoporosis: gender differences and similarities. Lupus 8:393–396
Karasik D, Ferrari SL (2008) Contribution of gender-specific genetic factors to osteoporosis risk. Ann Hum Genet 72:696–714
Merten M, Greiner JFW, Niemann T, Grosse Venhaus M, Kronenberg D, Stange R, Wahnert D, Kaltschmidt C, Vordemvenne T, Kaltschmidt B (2022) Human sex matters: Y-linked lysine demethylase 5D drives accelerated male craniofacial osteogenic differentiation. Cells 11:823
Minoia A, Dalle Carbonare L, Schwamborn JC, Bolognin S, Valenti MT (2022) Bone tissue and the nervous system: what do they have in common? Cells 12:51
Wang C, Gu W, Sun B, Zhang Y, Ji Y, Xu X, Wen Y (2017) CTHRC1 promotes osteogenic differentiation of periodontal ligament stem cells by regulating TAZ. J Mol Histol 48:311–319
Lee SH, Kang MI, Ahn SH, Lim KH, Lee GE, Shin ES, Lee JE, Kim BJ, Cho EH, Kim SW, Kim TH, Kim HJ, Yoon KH, Lee WC, Kim GS, Koh JM, Kim SY (2014) Common and rare variants in the exons and regulatory regions of osteoporosis-related genes improve osteoporotic fracture risk prediction. J Clin Endocrinol Metab 99:E2400-2411
Kimura H, Kwan KM, Zhang Z, Deng JM, Darnay BG, Behringer RR, Nakamura T, de Crombrugghe B, Akiyama H (2008) Cthrc1 is a positive regulator of osteoblastic bone formation. PLoS ONE 3:e3174
Zhang W, Dong Z, Li D, Li B, Liu Y, Zheng X, Liu H, Zhou H, Hu K, Xue Y (2021) Cathepsin K deficiency promotes alveolar bone regeneration by promoting jaw bone marrow mesenchymal stem cells proliferation and differentiation via glycolysis pathway. Cell Prolif 54:e13058
Lee BJ, Cox GA, Maddatu TP, Judex S, Rubin CT (2009) Devastation of bone tissue in the appendicular skeleton parallels the progression of neuromuscular disease. J Musculoskelet Neuronal Interact 9:215–224
Inoue K, Mikuni-Takagaki Y, Oikawa K, Itoh T, Inada M, Noguchi T, Park JS, Onodera T, Krane SM, Noda M, Itohara S (2006) A crucial role for matrix metalloproteinase 2 in osteocytic canalicular formation and bone metabolism. J Biol Chem 281:33814–33824
Li Z, Xu Z, Duan C, Liu W, Sun J, Han B (2018) Role of TCF/LEF transcription factors in bone development and osteogenesis. Int J Med Sci 15:1415–1422
Wanschitz F, Stein E, Sutter W, Kneidinger D, Smolik K, Watzinger F, Turhani D (2007) Expression patterns of Ets2 protein correlate with bone-specific proteins in cell-seeded three-dimensional bone constructs. Cells Tissues Organs 186:213–220
Eriksen EF, Charles P, Melsen F, Mosekilde L, Risteli L, Risteli J (1993) Serum markers of type I collagen formation and degradation in metabolic bone disease: correlation with bone histomorphometry. J Bone Miner Res 8:127–132
Makieva S, Dubicke A, Rinaldi SF, Fransson E, Ekman-Ordeberg G, Norman JE (2017) The preterm cervix reveals a transcriptomic signature in the presence of premature prelabor rupture of membranes. Am J Obstet Gynecol 216:602.e1-602.e21
Ortega N, Wang K, Ferrara N, Werb Z, Vu TH (2010) Complementary interplay between matrix metalloproteinase-9, vascular endothelial growth factor and osteoclast function drives endochondral bone formation. Dis Model Mech 3:224–235
Yumi Noronha N, da Silva Rodrigues G, Harumi Yonehara Noma I, Fernanda Cunha Brandao C, Pereira Rodrigues K, Colello Bruno A, Sae-Lee C, Moriguchi Watanabe L, Augusta de Souza Pinhel M, Mello Schineider I, Luciano de Almeida M, Barbosa Junior F, Araujo Morais D, Tavares de Sousa Junior W, Plosch T, Roberto Bueno Junior C, Barbosa Nonino C (2022) 14-weeks combined exercise epigenetically modulated 118 genes of menopausal women with prediabetes. Front Endocrinol (Lausanne) 13:895489
Lotinun S, Kiviranta R, Matsubara T, Alzate JA, Neff L, Luth A, Koskivirta I, Kleuser B, Vacher J, Vuorio E, Horne WC, Baron R (2013) Osteoclast-specific cathepsin K deletion stimulates S1P-dependent bone formation. J Clin Invest 123:666–681
Rauner M, Foger-Samwald U, Kurz MF, Brunner-Kubath C, Schamall D, Kapfenberger A, Varga P, Kudlacek S, Wutzl A, Hoger H, Zysset PK, Shi GP, Hofbauer LC, Sipos W, Pietschmann P (2014) Cathepsin S controls adipocytic and osteoblastic differentiation, bone turnover, and bone microarchitecture. Bone 64:281–287
Takahata Y, Hagino H, Kimura A, Urushizaki M, Kobayashi S, Wakamori K, Fujiwara C, Nakamura E, Yu K, Kiyonari H, Bando K, Murakami T, Komori T, Hata K, Nishimura R (2021) Smoc1 and Smoc2 regulate bone formation as downstream molecules of Runx2. Commun Biol 4:1199
Peeters T, Monteagudo S, Tylzanowski P, Luyten FP, Lories R, Cailotto F (2018) SMOC2 inhibits calcification of osteoprogenitor and endothelial cells. PLoS ONE 13:e0198104
Jenniskens YM, Koevoet W, de Bart AC, Weinans H, Jahr H, Verhaar JA, DeGroot J, van Osch GJ (2006) Biochemical and functional modulation of the cartilage collagen network by IGF1, TGFbeta2 and FGF2. Osteoarthritis Cartilage 14:1136–1146
Schaffler MB, Kennedy OD (2012) Osteocyte signaling in bone. Curr Osteoporos Rep 10:118–125
Wu C, Orozco C, Boyer J, Leglise M, Goodale J, Batalov S, Hodge CL, Haase J, Janes J, Huss JW 3rd, Su AI (2009) BioGPS: an extensible and customizable portal for querying and organizing gene annotation resources. Genome Biol 10:R130
Funding
The authors declare that no funds, grants, or other support were received during the preparation of this manuscript.
Author information
Authors and Affiliations
Contributions
All authors contributed to the study conception and design. Material preparation, data collection, and analysis were performed by Dongfeng Chen, Ying Li, and Qiang Wang. The first draft of the manuscript was written by Dongfeng Chen, Ying Li, and Peng Zhan and all authors commented on previous versions of the manuscript. All authors read and approved the final manuscript.
Corresponding author
Ethics declarations
Conflict of interest
Dongfeng Chen, Ying Li, Qiang Wang, and Peng Zhan declare that they have no conflict of interest.
Ethics Approval
This study was approved by the Ethics Committee of Clinical Research of Longyan First Hospital Affiliated to Fujian Medical University. The trials were conducted in compliance with the International Code of Medical Ethics of the World Medical Association, and all participants provided written informed consent.
Human and Animal Rights and Informed Consent
The study was performed according to the Declaration of Helsinki and was approved by the Ethics Committee of Clinical Research of Longyan First Hospital. This article does not contain any studies with animals.
Additional information
Publisher's Note
Springer Nature remains neutral with regard to jurisdictional claims in published maps and institutional affiliations.
Supplementary Information
Below is the link to the electronic supplementary material.
Rights and permissions
Springer Nature or its licensor (e.g. a society or other partner) holds exclusive rights to this article under a publishing agreement with the author(s) or other rightsholder(s); author self-archiving of the accepted manuscript version of this article is solely governed by the terms of such publishing agreement and applicable law.
About this article
Cite this article
Chen, D., Li, Y., Wang, Q. et al. Identification of Key Osteoporosis Genes Through Comparative Analysis of Men's and Women's Osteoblast Transcriptomes. Calcif Tissue Int 113, 618–629 (2023). https://doi.org/10.1007/s00223-023-01147-3
Received:
Accepted:
Published:
Issue Date:
DOI: https://doi.org/10.1007/s00223-023-01147-3