Abstract
In the late stage of oilfield development, it turns to be a new focus to search for residual oil in small or even micro structures. It is necessary to recognize and re-interpret the structure of developing areas with well logging and seismic data. In detailed seismic interpretation, horizon tracking is the most fundamental work. However, because of the inconsistency of energy field and multiple characteristics of non-marker beds, it is very difficult to track the cross-well part of horizons, which leads to great difference between the structural interpretation results and posterior well data. This paper proposes a method to minimize the error between the seismic interpretation result and the well data by forward modeling. Based on the conventional structural interpretation method, several main subfacies sand body models with known sedimentary background are established, which can summarize the principle of the seismic reflection variation caused by different lithology, litho-combination and thickness. Besides, cross-well sand body models are built to simulate seismic profiles in the study area. With the forward modeling as reference, detailed horizon tracking is accomplished. Thus, the error between the tracking result and well data is reduced, and it lays a good foundation for reservoir modeling, prediction and facies mapping in the next step.
Access provided by Autonomous University of Puebla. Download conference paper PDF
Similar content being viewed by others
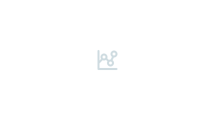
Keywords
1 Introduction
In detailed seismic interpretation, horizon tracking is a fundamental work. Although the theory is relatively simple, the heavy workload is sometimes easy to be ignored. The interpreted horizon will affect the accuracy of subsequent reservoir prediction and geological modeling. In the process of interpretation, due to the discontinuity of non-marker reflectors, tracking horizons becomes difficult to proceed, so interpreters need to take a variety of methods to improve the quality. Bondar (1992) presented image processing algorithms including the 2D median filtering of the instantaneous phase attribute. O’Malley and Kakadiaris (2004) presented a structure-enhancing adaptive filter guided by features derived from the Gradient Structure Tensor and employed this filter to reduce noise in seismic data and to assist in generating seed points for initializing an automatic horizon picking algorithm. Zinck et al. (2011) developed an algorithm to track a seismic horizon with a quasi-vertical discontinuity from the knowledge of the two points delimiting the horizon as well as the discontinuity location and jump. Aykroyd and Hamed (2014) proposed a statistical procedure aiming at smoothing out noise and using contextual information to identify coherent horizons for a rapid analysis. Figueiredo et al. (2015) proposed a clustering based methodology to map 3D horizons automatically. Goldner et al. (2015) proposed a tracking algorithm for 2D seismic horizons based on shortest paths in Directed Acyclic Graphs to balance global and local information of seismic data. Gogia et al. (2020) summarized the horizon tracking methods and categorized them into three groups: event correlation, artificial intelligence (AI) and flattening. Currently there are many studies of horizon tracking using deep learning method (e.g. Peters et al. 2019; Gupta et al. 2019; Koryagin et al. 2020; Tschannen et al. 2020). Bugge et al. (2019) presented a new automatic 3D method on data-driven horizon extraction from seismic images with nonlocal dynamic time warping and unwrapped instantaneous phase.
Forward modeling is by using geo-information to establish a geological model, and perform seismic simulation on the model to obtain an artificial seismic section, which can be used for comparison and verification with the real seismic section (e.g. Zhan 2017). In this forward modeling study the software Geoeast and SMI are mainly used. SMI provides the forward modeling function to obtain the synthetic profile, which is compared with the actual seismic profile to find out the principle of wavelet transform. Then the structural interpretation can be better instructed. In this study, forward modeling technology is used to establish the cross well sand body model. By comparing with the actual seismic profile and editing the model, the non-marker bed are calibrated. This method provides a reliable reference for interpretation, which is on one hand strictly consistent with well data, on the other hand fills the cross-well information gap.
2 Methods
2.1 Well Model
The forward modeling is established in the following steps: In the first step, the velocity model of the known sandstone and shale is given to SMI forward modeling module in depth domain. While inputting, in order to improve the accuracy, it needs to be established strictly according to the actual well litho-data; The second step is to assign corresponding layer velocity to the different geological bodies; In the third step, by taking numerous comparing tests, a zero-phase Rick wavelet with a dominant frequency of 35 Hz and a length of 100 ms is selected, which is similar to the seismic synthetic. 30% noise is input to simulate the underground situation.
2.2 Litho-Combination Model
The theory normally applied while interpreting thin layers is the “tuning principle”, that is, within the tuning thickness range, the thickness of a single sand layer is basically linear with the amplitude of wavelet. This principle can be used to quantitatively interpret thin layers based on the amplitude (e.g. Lu 1993). According to the research by Yuan et al. (1996), the thickness of sandstone is in the range of 0 ~ λ/4 (λ is the wavelength of seismic wavelet), and the amplitude increases with the scaling up of sandstone, and reaches the maximum at λ/4 (around 15 m).
In this study, four different 2D litho combination models of sandstone and shale in different thickness are established and forward simulated to seismic profile. This helps to find out the principle of wiggle transforming in the real seismic section. Then a well tie geological model is established according to the well litho data and geological principle. By comparing the simulated result and the actual profile, the model is corrected until the result is similar to the real one, so reasonable horizon top can be better calibrated.
3 Data Set
Location (after Zhao et al. 2012) and stratigraphic information (the target member is in bold highlighted) of SC oilfield.
The SC oilfield is located in the eastern fault zone of the Songliao Basin (see Fig. 1) and has a fractured ultra-low permeability sandstone reservoir. Block H lies in the central part of the SC oilfield. The current target layer is the third member of the Lower Cretaceous Denglouku Formation, which is diagnosed mainly as the delta front deposition. There are 4 members in Denglouku Formation. As the lower two members in this region were not deposited during uplift period, only the upper two members remains, the Deng-3 Member and the Deng-4 Member. The Deng-3 Member is the main oil reservoir, and the sand/shale ratio is between 50% and 81%. Deng-3 Member is further divided into 3 sandstone groups and 17 beds. In Block H, the reservoir has a shallow burial depth, poor physical properties, and vertically few oil layers. However, stable distribution of main reservoirs and clear oil-water interface are both advantages for exploration and production. At present, many drilled wells are known to have encountered oil layers and produce industrial oil flows. The comprehensive application of structural interpretation, reservoir inversion, sedimentary microfacies and conventional geological research results, is combined with well-seismic modeling technology for the optimization of horizontal well design. At this stage, the detailed seismic interpretation of the fault block, in which Well H661 is located, is carried out to lay a solid basement for reservoir inversion and modeling. Taking four vertical wells as standard wells, the establishment of well tie section is shown in Fig. 2.
The target horizon of this study is the sandstone reservoirs of D21–D23. According to well data, the oil-water interface is below the D23 layer of Well H6611. The target horizons of the other three wells are above the oil-water interface.
4 Results and Discussions
From the well lithology data, it can be seen that the target horizon is mainly interbedded sand-shale with a thickness of about 10 m. As horizontal wells will be drilled to raise the oil production in Block H, it is necessary to interpret the tops of the D21–D23 layers in the fault block to provide a credible reference. Forward modeling of different well models is performed (see Fig. 3), and the specific model velocity used in this study is compiled in Table 1. The results indicate that the target horizon in different well-log traces doesn’t have a uniformed characteristic.
The thickness of sandstone in the target layers is mostly less than 15 m. In accordance with the forward modeling of a thin-layered single sand body with varying thickness (see Fig. 4), only when the layer thickness reaches 3 m can an obvious reflector appear. So in the sand-shale interbedded model, the thinner a single layer of sandstone is, the lower is the amplitude.
The reservoir in the study area involves four lithology combination modes: thick shale with thick sandstone (model 1), thin sandstone with thin shale interbedded (below 4 m, model 2), thick shale with thin sandstone (model 3), and thick sandstone with thin shale (model 4). Corresponding forward simulations were carried out for different combination modes (see Fig. 5).
It can be seen from the simulation results that in model 1, the interface waveform at top and bottom of sand are strong peak and through. When the thin sandstone and shale are interbedded (model 2), it shows continuous weak amplitude, and the position of the interface is difficult to determine. The situation of model 4 is similar to model 2, which shows weak amplitude. Model 3 has obvious strong amplitudes at the top interface of sandstone.
With the results above, for the thin interbedded and thick sandstone with thin shale, the layer interface is not clear, and cannot be tracked accurately. Therefore, the model can be simplified by incorporating the thin layer of shale into the sandstone as a whole model. The simplified well model is shown in Fig. 6 (target layers are in red colored).
The study area is inside a fault block and has no reflection effects by faults. However, the thickness of the target layer is small, so the wavelet has interlayer interference, which leads to the appearance of some complex waves. The horizontal regularity of the reflector is inconsistent. Therefore, the non-marker bed is difficult to track. With the conventional interpretation, the forward simulation model is applied to reconfirm the position of horizons.
First, the well groups in the study area are preprocessed. AC, Den, and GR curves of each well are excluded from abnormal values, and caliper correction and multi-well standardization are performed. Then each well is synthesized with the Rick wavelet (35 Hz, 100 ms). The result of forward modeling is close to the result of the synthetics, and the cross-well seismic profile can be analyzed. The bifurcation and fusion of the reflectors are common phenomena in the process of horizon tracking (Fig. 7).
Based on the lithology data, D11, D21, and D31 are respectively the top surfaces of the I, II, and III groups of the third member of Denglouku Formation. D11 and D31 contain thick shale layers. No matter in the result of forward modeling or in the actual seismic profile, the reflectors are obvious, and the distribution range is wide, which can be tracked as a marker bed. Afterwards, the tracked D11 and D31 horizon can be considered as a boundary to constrain the tracking of target layers.
After analyzing the lithology and tops information, a well-tie profile model is established in accordance with the simulated well model. The forward modeling results are shown in Fig. 8. Using the reference of forward modeling, the regular pattern of waveform can be analyzed, which is in good agreement with the waveform at the marked parts in Fig. 7. The consequence is conducive to determine the position of the layer interface.
The red sand body in Fig. 8 represents the whole sandstone body of Group II. By multiple comparison and modification, the forward modeling result is close to the actual seismic profile. The shale layer at the top of D21 at well H6611 is thick, and the reflection interface of sand body is obvious, which is consistent with the well synthetic. The horizon of D21 top surface was tracked principally along the peak. When peak amplitude becomes weak, or complex wave appears, the position of layers’ interface in forward model is referred to, and manual calibration is carried out. After D21 horizon interpretation is completed, D22 and D23 can be limited to 3/4 λ by taking the usage of bottom interface D31. Since the thickness of the three layers is close, DII group can be divided equally in three parts. That is to say, D22 is mainly calibrated as the ± zero phase, and D23 is mainly calibrated as the trough. Then a regional velocity field is established by well T_D (Time-Depth) curve interpolation, and time to depth conversion is carried out. Verification is done by using top data of other directional wells in the area. Structural errors can be seen in Table 2, and the interpreted horizon in depth domain is then calipered by well top data.
The results indicate that the error of interpretation with help of forward modeling is lower than that of conventional non-model reference, especially at the position where multiplicity occurs in the cross-well part. In this way, horizon D22 and D23 are interpreted.
5 Conclusions
By forward modeling, interpreters can better understand the reflection characteristics of various lithologic combinations on seismic profiles, and provide a reference for horizon tracking. The use of seismic forward modeling can more accurately describe the stratum interface, and further improve the accuracy of the structure interpretation of the cross-well part. But at the same time, the ambiguity of seismic interpretation also makes it incapable of fully determining the geological body, and forward modeling will increase the workload of the interpreters. Therefore, this method should be used only on specific conditions like deviation calculation for horizontal well designing or detailed interpretation of local structures.
References
Aykroyd, R.G., Hamed, F.M.O.: Horizon detection in seismic data: an application of linked feature detection from multiple time series. Adv. Stat. 2014, 1–10 (2014)
Bondar, I.: Seismic horizon detection using image processing algorithms. Geophys. Prospect. 40, 785–800 (1992)
Bugge, A.J., Lie, J.E., Evensen, A.K., et al.: Automatic extraction of dislocated horizons from 3D seismic data using nonlocal trace matching. Geophysics 84(6), IM77–1M86 (2019)
Figueiredo, A.M., Silva, F.B., Silva, P.M., et al.: A clustering-based approach to map 3D seismic horizons. In: 14th International Congress of the Brazilian Geophysical Society, Rio de Janeiro, Brazil (2015)
Gogia, R., Singh, R., Gupta, H., et al.: Tracking 3D seismic horizons with a new hybrid tracking algorithm. Interpretation 8(4), 1–7 (2020)
Goldner, E.L., Vasconcelos, C.N., Silva, P.M., et al.: A shortest path algorithm for 2D seismic horizon tracking. In: SAC, Salamanca, Spain (2015)
Gupta, H., Pradhan, S., Gogia, R., et al.: Deep learning based automatic horizon identification from seismic data. In: SPE Annual Technical Conference and Exhibition, Calgary, Alberta, Canada (2019)
Koryagin, A., Mylzenova, D., Khudorozhkov, R., Tsimfer, S.: Seismic horizon detection with neural networks. Computer Vision and Pattern Recognition (2020)
Lu, J.: Principles of Seismic Exploration, vol. 2. University of Petroleum Press, Dongying (1993)
O’Malley, S.M., Kakadiaris, I.A.: Towards robust structure-based enhancement and horizon picking in 3-D seismic data. In: Proceedings of the 2004 IEEE Computer Society Conference on Computer Vision and Pattern Recognition, CVPR (2004)
Peters, B., Haber, E., Granek, J.: Neural networks for geophysicists and their application to seismic data interpretation. Lead. Edge 38, 534–540 (2019)
Tschannen, V., Delescluse, M., Ettrich, M., et al.: Extracting horizon surfaces from 3D seismic data using deep learning. Geophysics 85(3), N17–N26 (2020)
Yuan, Z., et al.: Research and application of forward modeling in time domain and frequency domain of thin interbed seismic reflection. Petrol. Geophys. Explor. 35(3), 14–20 (1996)
Zhan, X.: Forward modeling and seismic identification of igneous rocks in an area of Oriente Basin, Ecuador. Petrochem. Technol. 24(3), 162 (2017)
Zhao, R., Cheng, J., Zhang, K.: CO2 plume evolution and pressure buildup of large-scale CO2 injection into saline aquifers in Sanzhao Depression, Songliao Basin, China. Transp. Porous Media 95, 407–424 (2012)
Zinck, G., Donias, M., Guillon, S., et al.: Discontinuous seismic horizon tracking based on a Poisson equation with incremental Dirichlet boundary conditions. In: 18th IEEE International Conference on Image Processing, pp. 3446–3449 (2011)
Acknowledgments
The idea applying forward modeling to structural interpretation came from my colleague Mr. Zhang Hongmou, to whom I would like to express my gratitude. I also owe a special debt of gratitude to Mr. Professor Andreas Weller and Mr. Dr. Zhang Zeyu, who offered me valuable suggestions during my study. And I should finally express my gratitude to my families, who supported me when I came across difficulties.
Author information
Authors and Affiliations
Corresponding author
Editor information
Editors and Affiliations
Rights and permissions
Copyright information
© 2022 The Author(s), under exclusive license to Springer Nature Singapore Pte Ltd.
About this paper
Cite this paper
Zhao, Mx., Wu, Y., Yang, W., Zhang, Hm. (2022). Applied Forward Modeling in the Seismic Interpretation of Non-marker Beds in Delta Front. In: Lin, J. (eds) Proceedings of the International Field Exploration and Development Conference 2021. IFEDC 2021. Springer Series in Geomechanics and Geoengineering. Springer, Singapore. https://doi.org/10.1007/978-981-19-2149-0_303
Download citation
DOI: https://doi.org/10.1007/978-981-19-2149-0_303
Published:
Publisher Name: Springer, Singapore
Print ISBN: 978-981-19-2148-3
Online ISBN: 978-981-19-2149-0
eBook Packages: Earth and Environmental ScienceEarth and Environmental Science (R0)