Abstract
In France, trihoalomethanes (THM) are regulated and regularly monitored at the water treatment plant and more recently in the drinking water system. THM concentrations at tap water depend on many factors like chlorine level, organic precursor’s concentrations, water temperature, residence time in the network, and presence of rechlorination stations. To predict concentrations in the water distribution system using data collected from treated water at the plant (i.e., the entrance of the distribution system), a first mathematical model was developed in 2009, from three sites supplied by surface water. Predicted concentrations produced with this model for five new sites didn’t match with observed concentrations. New efforts were then made in order to adapt this mathematical model to cover more types of water. Two formulations have been developed: a first model based on a minimum of variables and those easily available (from the French national SISE-Eaux database collecting all data from drinking water regulations) and a second model that includes more information about the reactivity of the organic matter with chlorine. The choice of variables and the general shape of the models were made by dividing the database into two random editions of the couples of data (75% of the data to build the models/25% to validate them). The validation of both models (simplified and complete model) was satisfactory, explaining respectively 87% and 88% of the variance, with a good capacity of generalization. The models developed herein can be used to assess THM concentrations at different points between the treated water at the plant and the consumer’s tap in a large range of French water systems supplied by surface waters.
Access provided by Autonomous University of Puebla. Download reference work entry PDF
Similar content being viewed by others
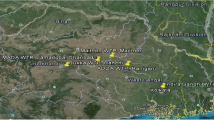
Keywords
- Drinking water
- Trihalomethanes
- Chlorine
- Chlorination byproducts
- Mathematical model
- Water distribution system
Introduction
Chlorination of drinking water is widely used around the world to prevent and the infectious risk conveyed by tap water. In France, its use dates from more than one century in several large cities. Since 2003, the French authorities have recommended to extend its use to all water systems regardless of the size of the population served. In 2007, more than 99% of produced drinking water were disinfected with chlorine (Davezac et al. 2008).
Because of its oxidizing properties, the chlorine reacts with water organic matter to form chlorination byproducts (SPC) . Nearly 600 SPC are identified to date (Richardson et al. 2007).
Trihalomethanes (THM) and haloacetic Acids (HAAs) account for between 20% and 30% of the total mass of the SPC produced generally (Weisel et al. 1999). Drinking water chlorination in France is mandatory under the national legislation, while regular inspections of recreational waters are also conducted regularly (Galey et al. 2015).
Water sampling is carried out at the outlet of the treatment stations having a chlorination step, and in network if the chlorine concentration in the distribution system exceeds 0.5 mg/L. The formation of SPC depends on the nature of the raw water, the treatments used to remove the organic matter and the disinfection strategy (injection points, applied doses, contact time).
The presence of SPC poses a public health problem due to associated health risks and the large size of exposed population. Epidemiological studies indicate anassociation between exposure to SPC , generally assessed by THM measurements as part of regulatory controls, and the occurrence of bladder cancer (Villanueva et al. 2007). An association between THM exposure and colorectal cancer is also doubtful (Rahman et al. 2010; Azhar et al. 2015). Suspected effects on reproduction and development, even if they are widely studied, are still controversial (Grellier et al. 2010; Lewis et al. 2011; Hwang and Jaakkola 2012; Levallois et al. 2012). Exposure estimation is generally the weak point of epidemiological studies.
THM formation evolves in the water distribution network . Several studies have showed an increase in THM concentrations by a factor of 2–6 between the treatment plant exit and periphery of the drinking water distribution system (Mouly et al. 2010).
A first regression model was constructed based on three production and distribution sites of drinking water in 2009 (Mouly et al. 2009, 2010) in order to predict THM concentrations in water systems from measured output data of treatment plants, with the aim of better estimating the population exposure. Data from five other production and distribution sites were used for external validation purposes.
The comparison of “2009” model predictions to the data measured on these five sites did not, however, allow to establish the validity of the model beyond the three sites considered for its construction.
The aim of the study is therefore to propose two variants of a new regression modelbased on the analysis of all the data (data from the three sites used for the establishment of the “2009” model and the five sites used for its external validation), in order to have a new model with a wider range of application.
A “complete model” using all the variables provided by the operators of the different sites was constructed, as well as a “simplified model” retaining a minimal subset of variables, reduced to those that are indispensable, or easily accessible and routinely produced.
Materials and Methods
Study Sites
Eight sites were used for model construction and validation. All these sites are fed by surface or retaining water, and comprise a complete treatment process with a filtration step on activated carbon or two-layer filtration, and an ozonization step.
There is no prechlorination step in the treatment process. Final disinfection by chlorine is carried out at the exit of the treatment plant before the distribution of the water in the network. The data come from various sampling campaigns of analyzes carried out in different seasons.
During each campaign, a sample was systematically carried out at the outlet of the treatment plant, downstream of the chlorination step at the treatment plant, and one to several samples were taken in different points of the distribution network , before or after a possible re-chlorination step.
As a result, the complete data used are distributed as follows for the different sites (Table 1):
Depending on the study site, several sampling points were chosen along the drinking water system . At each study site, sampling points included one point before the chlorination step, one point at the treated water at the plant (i.e., at the entrance to the drinking water network: reference point 0) and several points along the drinking water network with different residence times (Fig. 1).
Variation Range of the Studied Parameters
Table 2 presents the description of water quality variables and operating variables, which may influence the formation of THM. The incorporation of these variables in the “simplified” and “complete” models is given in the table.
The concentration is expressed in molar concentration (μmol.L−1) because the distribution of individual THM (chloroform, dichlorobromomethane, chlorodibromomethane, bromoform) is different depending on site and because the molar mass is different for each THM. The use of all data in the same model requires translation of the concentration into molar concentration.
Modelization
The method used to adjust the two models is based on the random division of datain two subsamples. The first, called the training sample, is made up of 75% of the available data and it’s used to build the model. The second, called test or validation sample, consists of the remaining 25% of the data and it’s used to measure the generalization capacity of the model by comparing its predictions to the observed values.
Explanatory variable is introduced as polynomial functions of 1–3 degrees in order to take into account the possible nonlinearity of the relationship between the levels of THM present in the network and the explanatory variables. Different regression models were then tested with the variables by introducing possible interactions. These models were assessed by considering:
-
1.
R2: the coefficient of determination which determines the contribution of the tested variables in the explanation of variability of the response; RMSE: the residual mean standard error which corresponds to the error making on prediction
-
2.
Assessment of the fit quality of the model by analyzing the graphic distribution of residues
-
3.
Prediction capacity of the model on data not used for its construction
(validation sample), evaluated on the basis of:
-
(a)
RMSE: root mean square error
-
(b)
Relative error N25: which represents the percentage of predictions with a relative error less than 25%
-
(c)
Relative error related to uncertainty N5unc: which represents the percentage of predictions with a relative error less than 5% when uncertainty on explanatory variables is taking into account
-
(a)
Higher values of N25% and N5unc mean that the model has a great prediction and generalization capacity.
The stability of the two models selected was verified by cross-validation on eight subsamples made randomly from the starting data sample. The work was done with software R (V2.14.2).
Results
Simplified Model
The search for a simplified model aims to have a predictive tool, using a minimal subset of easily accessible explanatory variables (present in the SISE-EAUX French database).
After exploring the relationship between THMi (THM concentration in the distribution network) and the available explanatory variables, the form of the simplified model is a polynomial form, of 1–3 degrees according to the variables, with a term of interaction between network rechlorination and water temperature (Table 3).
The fitting quality and predictive performance of this model are as follows:
Construction on the training sample (N = 197) | ||
R2 = 87.15% | RMSE = 0.0484 | p < 2.2e − 16 |
Validation on the test sample (N = 65) | ||
RMSE = 0.0625 | N25 = 67.7% | N5unc = 81.5% |
The simplified model adjusts well the observed data. Indeed, the histogram and the Q-Q plot of the residues show that the distribution of the residues is close to a normal distribution. Moreover, the residual values do not exhibit any particular tendency (Fig. 2).
Good predictive performances were also observed for the vast majority of the predictions of the validation sample. Predicted THM values were close to the observed ones (Fig. 3 – N25 close to 70% and N5unc greater than 80%).
The four observed atypical concentrations between 0.23 and 0.37 μmole.L−1, for which the simplified model predicts a value around 0.1 μmole.L–1, belong to the same site (site 7). They were all measured in the spring during the same campaign. The four sampling points are different, but have a double chlorination in the network and a residence time RT probably underestimated.
The form of the relationships observed between levels of THMi present in the network and each explanatory variable of the simplified model allows to assess the coherence of the relations with the mechanisms involved (Fig. 4).
A growing relationship is observed between the formation of THMi in the network and THM0 (THM concentration at the plant outlet), Cl20 (residual chlorine leaving the plant), RTi (residence time of water at the sample point i), and Temp0 (water temperature) when no rechlorination is used in the network. These results are in line with expectations.
The bell shape of the relationship with temperature in the presence of network rechlorination is more difficult to apprehend.
The relationship observed for the higher TOC0 (organic carbon of the distributed water greater than 3.5 mg.L−1) or high pH (pH > 8.3) have no explanation. The campaigns associated with these conditions are limited in number and concern only a few sites.
Complete Model
After exploring the relationship between THMi and the available explanatory variables, theform of the “complete model” was a polynomial form, of 1–3 degrees according to the variable, with a term of interaction between network rechlorination and water temperature (Table 4). The complete model uses the UV absorbance (at 254 nm) of water, as well as the variable R which define the chlorine consumption rate at the plant.
The fitting quality and predictive performance of this model are as follows (Figs. 5 and 6):
Construction on the training sample (N = 197) | ||
R2 = 88.45% | RMSE = 0.0467 | p < 2.2e-16 |
Validation on the test sample (N = 65) | ||
RMSE = 0.0563 | N25 = 67.7% | N5unc = 86.1% |
The forms of relations between THM concentrations present in the network and the explanatory variables used in the complete model are similar to those observed for the simplified model (not shown).
Conclusion
The model built in 2009 (Mouly et al. 2009) using data from three production and water distribution sites have not been validated on the new data collected from other sites. The quality of the water produced by the three initial sites was fairly similar, with THM concentration ranging from 10 to nearly 90 μg/L.
A new modeling was then undertaken, using data from eight sites: the three sites used for the construction of the 2009 model, and the five new sites. All these sites are fed by surface water and include a complete water treatment process with ozonation and filtration steps.
Two models were then built. The first is called “simplified.” It was built based on variables usually available from the sanitary control French basis and other indispensable variables as hydraulic residence time of water in the distribution network.
The second model is called “complete.” It is constructed from all the available variables. Compared to the “Simplified” model, it includes variables that better characterize the reactivity of organic matter to chlorine as UV absorbance and the rate of chlorine consumption in the plant.
The performances of these two models are very similar, with a slight improvement when moving from the simplified model to the complete model (increase of R2 from 87.15% to 88.45% and N5unc increase from 81.5% to 86.1%).
The field of application of these models seems to cover surface water and French conditions water treatments, for a wide range of THM concentration levels at the outlet of the treatment plant (between 1.3 and 68 μg/L).
The overall validity of the “simplified” and “complete” models leads us to propose their use to estimate THM content in a distribution network.
Many difficulties were met during this work in collecting entry data especially for hydraulic residence time data. Several sites initially proposed to contribute to the modeling work were not selected due to lack of exact data on relevant variables.
The use of these two models to predict a THM level at a point of a water distribution network is possible and easy to do under Excel®, providing data availability of explanatory variables. These models can be used to determine levels of THM concentrations at different points of the same network, and help identify the most critical areas, close to the regulatory standard for example.
The two models were not validated on waters and treatment processes other than thoseused for their construction. It would be interesting to have other datasets of new sites, in particular with underground water, in order to verify their ability to be generalized.
References
Azhar S, Sumayya S, Majid M, Mirza MH, Haider AK (2015) Multipathways human health risk assessment of trihalomethane exposure through drinking water. Ecotoxicol Environ Saf 116:129–136
Davezac H, Grandguillot G, Robin A, Saout C (2008) L’eau potable en France 2005–2006, p 63
Galey C, Zeghnoun A, Boudouch O, Beaudeau P, Rosin C (2015) Modélisation de la formation des trihalométhanes dans les réseaux de distribution d’eau destinés à la consommation humaine en France. Tech Sci Méthodes 6(1):20–31
Grellier J, Bennett J, Patelarou E, Smith RB, Toledano MB, Rushton L et al (2010) Exposure to disinfection by-products, fetal growth, and prematurity: a systematic review and meta-analysis. Epidemiology 21(3):300–313
Hwang BF, Jaakkola JJK (2012) Risk of stillbirth in the relation to water disinfection by-products: a population-based case-control study in Taiwan. PLoS One 7(3):e33949
Levallois P, Gingras S, Marcoux S, Legay C, Catto C, Rodriguez M et al (2012) Maternal exposure to drinking-water chlorination by-products and small-for-gestational-age neonates. Epidemiology 23(2):267–276
Lewis C, Hoggatt KJ, Ritz B (2011) The impact of different causal models on estimated effects of disinfection by-products on preterm birth. Environ Res 111(3):371–376
Mouly D, Joulin E, Rosin C, Beaudeau P, Zeghnoun A, Olszewski OA et al (2009) Les sous-produits de chloration dans l’eau destinée à la consommation humaine en France. Campagnes d’analyses dans quatre systèmes de distribution d’eau et modélisation de l’évolution des trihalométhanes. Institut de veille sanitaire, Saint-Maurice, p 73
Mouly D, Joulin E, Rosin C, Beaudeau P, Zeghnoun A, Olszewski OA et al (2010) Variations in trihalomethane levels in three French water distribution systems and the development of a predictive model. Water Res 44(18):5168–5179
Rahman MB, Driscoll T, Cowie C, Armstrong BK (2010) Disinfection by-products in drinking water and colorectal cancer: a meta-analysis. Int J Epidemiol 39:733–745
Richardson SD, Plewa MJ, Wagner ED, Schoeny R, Demarini DM (2007) Occurrence, genotoxicity, and carcinogenicity of regulated and emerging disinfection by-products in drinking water: a review and roadmap for research. Mutat Res 636(1-3):178–242
Villanueva CM, Cantor KP, Grimalt JO, Malats N, Silverman D, Tardon A et al (2007) Bladder cancer and exposure to water disinfection by-products through ingestion, bathing, showering, and swimming in pools. Am J Epidemiol 165(2):148–156
Weisel CP, Kim H, Haltmeier P, Klotz JB (1999) Exposure estimates to disinfection by-products of chlorinated drinking water. Environ Health Perspect 107(2):103–110
Author information
Authors and Affiliations
Corresponding author
Editor information
Editors and Affiliations
Section Editor information
Rights and permissions
Copyright information
© 2019 Springer Nature Switzerland AG
About this entry
Cite this entry
Boudouch, O., Galey, C., Rosin, C., Zeghnoun, A. (2019). Modelization of Trihalomethanes Formation in Drinking Water Distribution Systems in France. In: Hussain, C. (eds) Handbook of Environmental Materials Management. Springer, Cham. https://doi.org/10.1007/978-3-319-73645-7_155
Download citation
DOI: https://doi.org/10.1007/978-3-319-73645-7_155
Published:
Publisher Name: Springer, Cham
Print ISBN: 978-3-319-73644-0
Online ISBN: 978-3-319-73645-7
eBook Packages: Chemistry and Materials ScienceReference Module Physical and Materials ScienceReference Module Chemistry, Materials and Physics