Abstract
The distributions of arbuscular mycorrhizal (AM) fungal communities are driven by climate, soil nutrients, and plant community composition. However, these distributions are estimated at the community level and AM fungal taxa will respond to selection pressure of global change at the species level. Thus, ecological niche models of individual AM fungal taxa may be an informative approach to predict AM fungal composition and function under future climates at the global scale. Here we present the first attempt to model AM fungal distributions with ecological niche models for the widespread AM fungal taxon Rhizophagus irregularis (formerly Glomus intraradices). We show that despite varying the definition of the operational taxonomic unit (OTU) for R. irregularis, the predicted distributions of this species complex are consistently affected by a positive association with soil moisture. The spatial extent of ecological niche models affected the predicted distribution of R. irregularis, with climatic drivers and resources affecting its distribution more strongly in the northern and southern hemispheres, respectively. Given that AM fungi are not dispersal limited and coexist at the landscape scale relevant for ecological niche model predictions, this widely distributed fungal clade provides a robust case study to apply hypothesis-driven distributional models to predict the biogeography of microorganisms.
Access provided by CONRICYT-eBooks. Download chapter PDF
Similar content being viewed by others
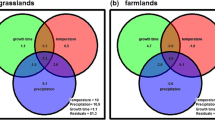
Keywords
- Arbuscular mycorrhizal fungi
- Glomeromycota
- Climate envelope modeling
- Macroecology
- Species distribution modeling
7.1 Introduction
Arbuscular mycorrhizal (AM) fungi are globally distributed, obligate, belowground symbionts that associate with up to 80% of all plant species (Smith and Read 2008; Kivlin et al. 2011; Öpik et al. 2013; Davison et al. 2015; Soudzilovskaia et al. 2015a). Typically, AM fungi improve host plant growth by providing soil nutrients (Smith and Read 2008), water (Augé 2001), and pathogen protection (Sikes et al. 2010). In doing so, they can influence C, N, and P dynamics within ecosystems, and—given their worldwide abundance—at the global scale as well (Mohan et al. 2014; Soudzilovskaia et al. 2015b). By considering the global distribution and functions of AM fungi, we may better predict large-scale C, N, and P cycling (Brzostek et al. 2014; Treseder 2016). Additionally, because AM fungal taxa vary in their effects on plant growth and nutrient uptake (van der Heijden et al. 1998a; Maherali and Klironomos 2007; Chagnon et al. 2013; Johnson et al. 2013), it is worthwhile considering the global biogeography of individual AM taxa.
AM fungi can be particularly sensitive to global change, because their function (Treseder 2004; Johnson et al. 2010; Kivlin et al. 2013) and community composition (Yang et al. 2013a) are affected by environmental conditions. For example, AM fungal taxa differ in their responses to climate (Kivlin et al. 2011; Davison et al. 2015), soil nutrients (Xiang et al. 2014), and plant community composition (Öpik et al. 2010). Thus, human activities that alter these conditions could, in turn, change the distribution of AM fungal taxa.
Given the importance of environmental variables in determining the distribution of AM fungal species, ecological niche models may provide robust predictions of distributions of individual AM fungal taxa. Ecological niche models, or species distribution models, use underlying variation in environmental conditions and known species occurrences to predict which unexplored areas may contain optimal habitat for a focal taxon (Phillips et al. 2006). These models are commonly used to determine potential habitat for plant and animal species (Peterson et al. 2002), define cryptic species (Raxworthy et al. 2007), predict invasion success (Peterson 2003), and model the spread of crop pests (Venette et al. 2010). However, ecological niche models do not incorporate dispersal limitation or competition, which may result in narrower realized distributions of taxa than predicted by these models (reviewed by Sinclair et al. 2010). Because there is limited evidence of short-term dispersal limitation for AM fungi (Davison et al. 2015) and competition among AM fungal taxa occurs at very small spatial scales (Maherali and Klironomos 2012), ecological niche models have the potential to accurately predict large-scale species distribution of these taxa, perhaps even better than current models for macroorganisms (Pearson and Dawson 2003). Indeed, ecological niche models have successfully modeled the niche for fungal pathogens (e.g., Baptista-Rosas et al. 2007; Reed et al. 2008); yet they have not been applied to mutualistic fungal taxa.
Once taxon-specific distributions are understood, they could then be leveraged to predict AM fungal functions across large spatial scales in cases where functions are well understood. For example, traits of AM fungi that are influential in nutrient acquisition, such as intra- and extraradical colonization rates, are phylogenetically conserved (Powell et al. 2009; Maherali and Klironomos 2012). AM fungi also exhibit generalizable and well-characterized diversity-productivity relationships (van der Heijden et al. 1998b). Thus it is relatively straightforward to link taxon distributions to well-known trait distributions for this clade. Because AM fungi are so well studied, this system provides an excellent case for linking microbial composition to ecosystem function (Treseder 2016), allowing inference of ecosystem process rates from simple community-based metrics.
7.2 Importance of Species Level Models of AM Fungal Distribution
Despite the promise that AM fungal community composition is indicative of function, we currently lack predictive models of AM fungal distribution under current or future climates. Instead, the factors affecting AM fungal composition are measured via community-wide metrics based on observational data of composition and underlying environmental conditions.
However, the community level is not the correct scale of inference to predict how AM fungi will respond to global change. Community-wide metrics, such as Bray-Curtis distance of beta-diversity among sites, are biased in their interpretation because they often favor the most abundant or widespread taxa while marginalizing the effects of rare AM fungi (Wolda 1981; Plotkin and Muller-Landau 2002). Instead, understanding the factors that affect the distribution of individual AM fungi will ultimately yield the most predictive models of AM fungal distributions, because the capability of AM fungi to disperse, adapt, or acclimate to environmental change is controlled by selection at the species level (Vellend 2010). For example, spore size varies among AM fungal taxa, which could limit the short-term dispersal ability of some large-spored species (e.g., Gigaspora gigantea), while other taxa (e.g., Archaeospora schenckii) may be less affected (Kivlin et al. 2014). In addition, local adaptation of AM fungal taxa to both soil nutrient concentrations (Johnson et al. 2010; Rúa et al. 2016) and climate (Antunes et al. 2010) suggests that AM fungal taxa may differentially respond to these drivers as well. Evidence of AM fungal acclimation is rare but can occur in response to temperature (Heinemeyer et al. 2006; Hawkes et al. 2008). Creating ecological niche models at the species level does not preclude community-level inference; once the distributions of individual fungal taxa are understood, these can be aggregated to infer potential community composition in the absence of competition at any given site (Thuiller et al. 2015). Because ecological niche models predict composition in the absence of biotic interactions, comparing models to actual communities can also help to infer the role of biotic interactions in community assembly (Wisz et al. 2013; Calabrese et al. 2014).
7.3 Testing Niche Modeling in a Common AM Fungal Taxon
Here we apply ecological niche modeling techniques to the most abundant and widespread AM fungus, Rhizophagus irregularis (formerly Glomus intraradices), to illustrate when this technique is useful for predicting where this microbial species occurs and to determine potential drawbacks of this technique. We use a presence-only modeling approach whereby environmental conditions at locations of known species occurrences are compared to environmental conditions at “background” locations (Phillips et al. 2006). We ran three models to predict R. irregularis distribution: (1) a full model including all (i.e., climate and resource) variables, (2) a climate model including Bioclim variables and soil moisture, and (3) a resource model including soil resources and plant net primary productivity. We expected that R. irregularis distributions would be affected by both climate and soil resources given current understanding of the factors affecting AM fungi at the global scale. Despite a long tradition of determining ecological niches of plants and animals (Grinnell 1917; Elton 1927), to our knowledge, this is the first attempt to predict AM fungal niches at the global scale.
7.3.1 Species Definitions
AM fungal species in current databases (e.g., MAARJAM) are typically defined as sharing at least 97% of DNA bases in conserved 18S ribosomal subunit genes (Öpik et al. 2010). However, the most appropriate species definition of AM fungi is currently being debated (see Davison et al. 2015; Bruns and Taylor 2016; Öpik et al. 2016). Virtual taxa in the MAARJAM database may represent species complexes that more closely resemble family-level resolution in plant and animal clades (Bruns and Taylor 2016). This feature may be particularly relevant for R. irregularis, which is one of the most genetically diverse AM fungal morphospecies (Börstler et al. 2008). Therefore, we examined how varying the OTU definition based on sharing 95, 97, 99, or 99.5% of bases in the 18S gene affected the predicted niche of R. irregularis. We expected that genetic resolution could change the importance of individual drivers of R. irregularis distributions, but overall interpretation of the importance of climatic vs. resource drivers would not vary.
7.3.2 Spatial Resolution
Sampling effort of AM fungi to date is biased in favor of northern hemisphere locations (Kivlin et al. 2011). Consequently, global niche models may be biased to highlight only the predictive drivers of AM fungal distribution in northern latitudes. For example, because of glaciation, soil nutrients are more limiting in equatorial ecosystems (Vitousek and Howarth 1991), whereas more extreme climates are a greater constraint at temperate and boreal latitudes. These environmental drivers have been hypothesized to control the distributions of many taxa (MacArthur 1972). There is some evidence that soil resources and climate affect distribution of plant (Condit et al. 2013) and animal (Parmesan et al. 2000) species. However, a synthetic comparison of the relative importance of these drivers on species distributions at the global scale has not been conducted. Thus, we predicted that niches of R. irregularis in North America and Eurasia would be most affected by climate, whereas soil resources would drive niches in South America and Africa.
7.3.3 Data Acquisition
DNA sequences of the 18S gene of R. irregularis were collected from published studies in the GenBank database through December 17, 2015. Sequences were aligned with the MAFFT aligner (Katoh et al. 2002) using PASTA (Mirarab et al. 2015). Sequences were then separated into operational taxonomic units (OTUs) with either 95, 97, 99, or 99.5% sequence similarity using the mothur farthest neighbor algorithm in QIIME (Caporaso et al. 2010). This created two 95% OTUs, four 97% OTUs, three 99% OTUs, and one 99.5% OTU with at least ten occurrences in the dataset (Table 7.1). A representative sequence of each OTU was queried against the MAARJAM database to confirm identity to R. irregularis (VTX00114).
For each entry, we collected the latitude and longitude of the sample from GenBank. Locations were used to infer environmental characteristics including both climate and resource variables. Climate information was based on raster layers obtained from Bioclim (Hijmans et al. 2005), which included mean diurnal temperature range, isothermality, maximum temperature in the warmest month, minimum temperature in the coldest month, mean temperature in the wettest quarter, mean temperature in the warmest quarter, mean annual precipitation, precipitation in the wettest month, precipitation in the driest month, precipitation seasonality, precipitation of the warmest quarter, and precipitation of the coldest quarter; we further included soil moisture derived solely from climate variables (Willmott et al. 1985). Resource-related parameters were net primary productivity (NPP) (Foley et al. 1996), soil carbon (C), soil pH (IGBP-DIS), soil percent clay (Hengl et al. 2014), and soil phosphorus (P) (Yang et al. 2013b). Because Bioclim variables are highly correlated, we retained only the nonredundant variables (excluding mean annual temperature, temperature seasonality, temperature annual range, mean temperature in the driest quarter, mean temperature of the coldest quarter, precipitation of the wettest quarter, and precipitation of driest quarter) (Ricklefs and He 2016). Resolution of all raster layers was standardized to 10 arc min.
To understand the spatial variability of R. irregularis niches across continents, separate models were constructed on the full dataset of R. irregularis occurrences in Africa, Eurasia, North America, and South America, as these were the only geographic areas with over ten occurrences.
For the entire dataset and each OTU and continent, we created three main models: (1) a model that included all of the environmental (climate and soil) variables (hereafter full model), (2) a model with only nonredundant Bioclim variables (listed above; Ricklefs and He 2016) and soil moisture (hereafter climate-only model), and (3) a model with all other soil and resource variables (NPP, soil C, soil pH, percent soil clay, and soil P; hereafter resource-only model). By comparing the output of these models, we determined the relative influence of climate and resources on R. irregularis distributions across genetic and spatial scales.
7.3.4 Ecological Niche Model Parameters
We built ecological niche models using the MaxEnt algorithm (Phillips et al. 2006) and we used the ENMeval v 0.2.0 R package (Muscarella et al. 2014) to “tune” model parameters to balance fit and predictive ability. We used a two-stage process of model selection to first determine the optimal model complexity for each of the three main models described above and then to identify which of the three main models best described occurrence patterns for R. irregularis. Specifically, in the first stage, we separately evaluated a range of candidate models across a range of complexity by allowing for different possible combinations of feature classes (i.e., linear, quadratic, hinge, threshold, and product) and regularization multiplier values (Merow et al. 2013). We used k-fold cross validation to evaluate model performance for each combination of parameters. For this, we partitioned occurrence records and background points into testing and training bins using the “checkerboard2” method in ENMeval (using default settings for aggregation factors). We used variable importance metrics generated by MaxEnt to determine the relative explanatory power of each predictor variable in our models. Performance was assessed with AUC (Hanley and McNeil 1982), OR10 (Fielding and Bell 1997), and AICc (Burnham and Anderson 2004). In each case, the best fit model (full, climate-only, or resource-only) was chosen using AICc. All model runs, raster manipulations, and distribution visualizations were performed using the dismo v. 1–0.15 (Hijmans and Elith 2012) and ENMeval v. 0.2.0 (Muscarella et al. 2014) packages in R v. 3.2.4 (R Development Core Team 2009).
7.4 Model Output
At all levels of genetic resolution, both climatic and resource variables influenced the distributions of R. irregularis at the global scale (Table 7.1 and Fig. 7.1). However, the influence of climate was stronger in most cases. For all data points and each 95% OTU, soil moisture was the strongest predictor for R. irregularis occurrence, with higher probability of occurrence in wetter soils. When OTUs were delineated at 97% sequence similarity, a positive correlation with soil moisture was still the main predictive variable for one out of the four OTUs, but negative associations with precipitation seasonality and isothermality, as well as a peak at intermediate NPP, also explained some variation in occurrence of three out of the four OTUs. At 99% sequence similarity, a positive association with soil moisture and negative association with precipitation seasonality explained the variation of both two tested OTUs. The 99.5% OTU distribution was best explained by a negative correlation with diurnal temperature range.
The drivers of potential distribution of R. irregularis varied across continents. Potential distribution in Eurasia and North America was driven by climate—positive effects of precipitation seasonality and peaking at intermediate minimum temperatures in the coldest month, respectively (Fig. 7.2). In contrast, the niche of R. irregularis in South America was controlled by a positive association with soil C, whereas the niche in Africa was driven by both climate (negative association with mean temperature in the warmest quarter) and resources (positive association with soil P).
Overall, based on our 15 final AICc-selected AM fungal ecological niche models, 87% had high AUC scores (i.e., AUC > 0.80), indicating accurate discrimination of AM fungal presence from background points. Omission rates were also fairly low (mean OR10 = 0.25), indicating that models were generally not overfit. The AICc-selected models based on different species resolution tended to have better performance than the spatial models, likely because of the higher overall sample size (e.g., species resolution models had an average AUC of 0.90 versus 0.79 for the spatial extent models). In particular, some of spatial models had high omission rates (e.g., 0.42 and 0.50 for South America and Africa, respectively), suggesting overfitting. In contrast, the average omission rate for OTU models was 0.21.
As we hypothesized, the genetic resolution of species definition for the R. irregularis species complex affected the relative importance of factors affecting ecological niche models. However, the most important drivers in every case were climatic, with soil moisture dominating the distribution of 55% of OTUs. Therefore, despite the current debate about the “true” definition of AM fungal species, current databases of virtual taxa still provide relevant information about the importance of climatic versus resource-related drivers of AM fungal distributions.
The spatial scale of the ecological niche models affected AM fungal distribution much more than genetic resolution. As expected, ecological niche models constructed in mostly temperate and boreal latitudes reflected the influence of climate on AM fungal distribution, whereas those from mostly tropical regions highlighted the influence of soil resources. The congruence of these models with previous modeling attempts for plants and animals suggests that tropical nutrient limitation and temperate climatic variability may also affect mycorrhizal life forms. This is also consistent with community-level mycorrhizal fungal patterns (e.g., Tedersoo et al. 2014). However, we have only examined a single complex AM fungal taxon; additional work will be needed to generalize these patterns.
7.5 Limitations of Ecological Niche Models
Despite the promise of ecological niche models to infer the factors affecting microbial distribution, they do not capture several dynamic aspects that may influence microbial biogeography. For example, dispersal is not explicitly represented in ecological niche models (Soberón 2007). If Glomeromycota dispersal indeed is not limiting (Davison et al. 2015), this constraint may not be meaningful. However dispersal of AM fungi remains poorly understood. In addition, for obligate plant symbionts, such as the AM fungi modeled here, host distribution and association preference are not taken into account. While AM fungi are mostly host species generalists (Öpik et al. 2013), variation in function among AM fungal hosts (Rúa et al. 2016) may affect both fungal and host fitness, with implications for AM fungal niches. These models also assume that species are at equilibrium in the environment (Yackulic et al. 2015), which may not be true since suitable habitat space fluctuates regularly for reasons as varied as seasonality, disturbance, plant succession, and global change. There was also a substantial sampling bias of both AM fungal composition and underlying environmental layers toward northern hemisphere locations that may skew the interpretation of our models. For example, only 33 of the 147 occurrences of R. irregularis in the current dataset were in South America or Africa. The models based on these records suffered from overfitting, and further work will be required to generate robust estimates of species ecological niches, particularly in these areas. As appreciation of this sampling bias is realized, more geographically explicit sampling schemes can only improve the resolution of global ecological niche models for microorganisms. Finally, by their nature, MaxEnt models only model occurrence records and do not take into account true absences. It is currently difficult to assess true absences of microbial species due to low sequencing effort and primer bias, but as sequencing methodology and depth improve, future distribution modeling may benefit from presence and absence data.
7.6 The Future of Ecological Niche Models of AM Fungi
Ultimately, AM fungal ecological niche models should be combined with similar models of their plant hosts. If both AM fungi and their hosts are affected by climate, and dispersal limitation does not limit migration, we can project future ranges based on our current understanding of climate change projections (with caveats as mentioned above). Attempts to predict biogeographical ranges are common for plants at large scales (Bakkenes et al. 2002), but only two localized studies (Pellissier et al. 2013; Bueno de Mesquita et al. 2015) have incorporated fungal symbionts that may hinder or ameliorate plant environmental stress tolerance and only under current environmental conditions. In addition, understanding not only the distribution but also the demographic rates of symbiotic fungi across environmental gradients will aid in determining the future distributions of these species (Merow et al. 2014). For example, if current ecological niche models indicate that soil moisture is the most influential variable for current AM fungal distribution, but temperature is more influential on AM fungal spore production and fitness (Schenck and Smith 1982; Zhang et al. 2016), then future AM fungal populations may not track current drivers of biogeography. Integrating performance-based metrics of microbial population dynamics into spatially explicit ecological niche models will be necessary to capture these processes.
Nevertheless, current datasets across broad spatial scales and taxonomic levels are ushering in a new age of microbial biogeography. By comparing distribution patterns of individual AM fungal taxa, we can predict simple macroecological patterns for these, for example, range size. Furthermore, because computationally stacking distribution patterns of individual AM fungal taxa can predict their diversity and community composition, these models can also be used to elucidate community-level patterns, such as latitudinal gradients in diversity or species turnover across environmental gradients. The macroecological hypotheses generated from ecological niche modeling techniques can then be tested with molecular surveys, allowing for a predictive microbial biogeography framework.
References
Antunes PM, Koch AM, Morton JB, Rillig MC, Klironomos JN (2010) Evidence for functional divergence in arbuscular mycorrhizal fungi from contrasting climatic origins. New Phytol 189:507–514
Augé RM (2001) Water relations, drought and vesicular-arbuscular mycorrhizal symbiosis. Mycorrhiza 11:3–42
Bakkenes M, Alkemade JRM, Ihle F, Leemans R, Latour JB (2002) Assessing effects of forecasted climate change on the diversity and distribution of European higher plants for 2050. Glob Change Biol 8:390–407
Baptista-Rosas RC, Hinojosa A, Riquelme M (2007) Ecological niche modeling of Coccidioides spp. in Western North American deserts. Ann N Y Acad Sci 1111:35–46
Börstler B, Raab PA, Thiéry O, Morton JB, Redecker D (2008) Genetic diversity of the arbuscular mycorrhizal fungus Glomus intraradices as determined by mitochondrial large subunit rRNA gene sequences is considerably higher than previously expected. New Phytol 180:452–465
Bruns TD, Taylor JW (2016) Comment on “global assessment of arbuscular mycorrhizal fungus diversity reveals very low endemism”. Science 351:826
Brzostek ER, Fisher JB, Phillips RP (2014) Modeling the carbon cost of plant nitrogen acquisition: mycorrhizal trade-offs and multipath resistance uptake improve predictions of retranslocation. J Geophys Res-Biogeo 119:1684–1697
Bueno de Mesquita CP, King AJ, Schmidt SK, Farrer EC, Suding KN (2015) Incorporating biotic factors in species distribution modeling: are interactions with soil microbes important? Ecography 39:970–980
Burnham KP, Anderson DR (2004) Multimodel inference: understanding AIC and BIC in model selection. Sociol Methods Res 33:261–304
Calabrese JM, Certain G, Kraan C, Dormann CF (2014) Stacking species distribution models and adjusting bias by linking them to macroecological models. Glob Ecol Biogeogr 23:99–112
Caporaso JG, Kuczynski J, Stombaugh J, Bittinger K, Bushman FD, Costello EK, Fierer N, Pena AG, Goodrich JK, Gordon JI et al (2010) QIIME allows analysis of high-throughput community sequencing data. Nat Methods 7:335–336
Chagnon PL, Bradley RL, Maherali H, Klironomos JN (2013) A trait-based framework to understand life history of mycorrhizal fungi. Trends Plant Sci 18:484–491
Condit R, Engelbrecht BMJ, Pino D, Pérez R, Turner BL (2013) Species distributions in response to individual soil nutrients and seasonal drought across a community of tropical trees. Proc Natl Acad Sci U S A 110:5064–5068
Davison J, Moora M, Öpik M, Adholeya A, Ainsaar L, Bâ A et al (2015) Global assessment of arbuscular mycorrhizal fungus diversity reveals very low endemism. Science 349:970–973
Elton CS (1927) Animal ecology. The Macmillan, New York
Fielding AH, Bell JF (1997) A review of methods for the assessment of prediction errors in conservation presence/absence models. Environ Conserv 24:38–49
Foley JA, Prentice IC, Ramankutty N, Levis S, Pollard D, Sitch S et al (1996) An integrated biosphere model of land surface processes, terrestrial carbon balance, and vegetation dynamics. Global Biogeochem Cycles 10:603–628
Grinnell J (1917) The niche-relationships of the California thrasher. Auk 34:427–433
Hanley JA, McNeil BJ (1982) The meaning and use of the area under the receiver operating characteristic (ROC) curve. Radiology 143:29–36
Hawkes CV, Hartley IP, Ineson P, Fitter AH (2008) Soil temperature affects carbon allocation within arbuscular mycorrhizal networks and carbon transport from plant to fungus. Glob Change Biol 14:1181–1190
Heinemeyer A, Ineson P, Ostle N, Fitter A (2006) Respiration of the external mycelium in the arbuscular mycorrhizal symbiosis shows strong dependence on recent photosynthates and acclimation to temperature. New Phytol 171:159–170
Hengl T, de Jesus JM, MacMillan RA, Batjes NH, Heuvelink GBM, Ribeiro E et al (2014) SoilGrids1km? Global soil information based on automated mapping. PLoS One 9:e105992
Hijmans RJ, Elith J (2012) Species distribution modeling with R. http://cran.r-project.org/web/packages/dismo/vignettes/dm.pdf
Hijmans RJ, Cameron SE, Parra JL, Jones PG, Jarvis A (2005) Very high resolution interpolated climate surfaces for global land areas. Int J Climatol 25:1965–1978
Johnson NC, Wilson GWT, Bowker MA, Wilson JA, Miller RM (2010) Resource limitation is a driver of local adaptation in mycorrhizal symbioses. Proc Natl Acad Sci U S A 107:2093–2098
Johnson NC, Angelard C, Sanders IR, Kiers ET (2013) Predicting community and ecosystem outcomes of mycorrhizal responses to global change. Ecol Lett 16:140–153
Katoh K, Misawa K, Kuma KI, Miyata T (2002) MAFFT: a novel method for rapid multiple sequence alignment based on fast Fourier transform. Nucleic Acids Res 30:3059–3066
Kivlin SN, Hawkes CV, Treseder KK (2011) Global diversity and distribution of arbuscular mycorrhizal fungi. Soil Biol Biochem 43:2294–2303
Kivlin SN, Emery SM, Rudgers JA (2013) Fungal symbionts alter plant responses to global change. Am J Bot 100:1445–1457
Kivlin SN, Winston GC, Goulden ML, Treseder KK (2014) Environmental filtering affects soil fungal community composition more than dispersal limitation at regional scales. Fungal Ecol 12:14–25
MacArthur RH (1972) Geographical ecology: patterns in the distribution of species. Princeton University Press, Princeton
Maherali H, Klironomos JN (2007) Influence of phylogeny on fungal community assembly and ecosystem functioning. Science 316:1746–1748
Maherali H, Klironomos JN (2012) Phylogenetic and trait-based assembly of arbuscular mycorrhizal fungal communities. PLoS One 7:e36695
Merow C, Smith MJ, Silander JA (2013) A practical guide to MaxEnt for modeling species’ distributions: what it does, and why inputs and settings matter. Ecography 36:1058–1069
Merow C, Latimer AM, Wilson AM, McMahon SM, Rebelo AG, Silander JA (2014) On using integral projection models to generate demographically driven predictions of species’ distributions: development and validation using sparse data. Ecography 37:1167–1183
Mirarab S, Nguyen N, Guo S, Wang L-S, Kim J, Warnow T (2015) PASTA: ultra-large multiple sequence alignment for nucleotide and amino-acid sequences. J Comput Biol 22:377–386
Mohan JE, Cowden CC, Baas P, Dawadi A, Frankson PT, Helmick K et al (2014) Mycorrhizal fungi mediation of terrestrial ecosystem responses to global change: mini-review. Fungal Ecol 10:3–19
Muscarella R, Galante PJ, Soley-Guardia M, Boria RA, Kass JM, Uriarte M et al (2014) ENMeval: an R package for conducting spatially independent evaluations and estimating optimal model complexity for Maxent ecological niche models. Methods Ecol Evol 5:1198–1205
Öpik M, Vanatoa A, Vanatoa E, Moora M, Davison J, Kalwij JM et al (2010) The online database MaarjAM reveals global and ecosystemic distribution patterns in arbuscular mycorrhizal fungi (Glomeromycota). New Phytol 188:233–241
Öpik M, Zobel M, Cantero J, Davison J, Facelli J, Hiiesalu I et al (2013) Global sampling of plant roots expands the described molecular diversity of arbuscular mycorrhizal fungi. Mycorrhiza 23:411–430
Öpik M, Davison J, Moora M, Partel M, Zobel M (2016) Response to comment on “global assessment of arbuscular mycorrhizal fungus diversity reveals very low endemism”. Science 351:826
Parmesan C, Root T, Willig M (2000) Impacts of extreme weather and climate on terrestrial biota. B Am Meteorol Soc 81:443–450
Pearson RG, Dawson TP (2003) Predicting the impacts of climate change on the distribution of species: are bioclimate envelope models useful? Glob Ecol Biogeogr 12:361–371
Pellissier L, Pinto-Figueroa E, Niculita-Hirzel H, Moora M, Villard L, Goudet J et al (2013) Plant species distributions along environmental gradients: do belowground interactions with fungi matter? Front Plant Sci 4:1–9
Peterson AT (2003) Predicting the geography of species’ invasions via ecological niche modeling. Q Rev Biol 78:419–433
Peterson AT, Ortega-Huerta MA, Bartley J, Sanchez-Cordero V, Soberon J, Buddemeier RH et al (2002) Future projections for Mexican faunas under global climate change scenarios. Nature 416:626–629
Phillips SJ, Anderson RP, Schapire RE (2006) Maximum entropy modeling of species geographic distributions. Ecol Model 190:231–259
Plotkin JB, Muller-Landau HC (2002) Sampling the species composition of a landscape. Ecology 83:3344–3356
Powell JR, Parrent JL, Hart MM, Klironomos JN, Rillig MC, Maherali H (2009) Phylogenetic trait conservatism and the evolution of functional trade-offs in arbuscular mycorrhizal fungi. Proc R Soc B 276:4237–4245
R Development Core Team (2009) R: a language and environment for statistical computing. R Foundation for Statistical Computing, Vienna
Raxworthy CJ, Ingram CM, Rabibisoa N, Pearson RG (2007) Applications of ecological niche modeling for species delimitation: a review and empirical evaluation using day geckos (Phelsuma) from Madagascar. Syst Biol 56:907–923
Reed KD, Meece JK, Archer JR, Peterson AT (2008) Ecologic niche modeling of Blastomyces dermatitidis in Wisconsin. PLoS One 3:e2034
Ricklefs RE, He F (2016) Region effects influence local tree species diversity. Proc Natl Acad Sci U S A 113:674–679
Rúa MA, Antoninka A, Antunes PM, Chaudhary VB, Gehring C, Lamit LJ et al (2016) Home-field advantage? evidence of local adaptation among plants, soil, and arbuscular mycorrhizal fungi through meta-analysis. BMC Evol Biol 16:122
Schenck NC, Smith GS (1982) Responses of six species of vesicular-arbuscular mycorrhizal fungi and their effects on soybean at four soil temperatures. New Phytol 92:193–201
Sikes BA, Powell JR, Rillig MC (2010) Deciphering the relative contributions of multiple functions within plant-microbe symbioses. Ecology 91:1591–1597
Sinclair SJ, White MD, Newell GR (2010) How useful are species distribution models for managing biodiversity under future climates? Ecol Soc 15:8
Smith SE, Read DJ (2008) Mycorrhizal symbiosis, 3rd edn. Academic, New York
Soberón J (2007) Grinnellian and Eltonian niches and geographic distributions of species. Ecol Lett 10:1115–1123
Soudzilovskaia NA, Douma JC, Akhmetzhanova AA, van Bodegom PM, Cornwell WK, Moens EJ et al (2015a) Global patterns of plant root colonization intensity by mycorrhizal fungi explained by climate and soil chemistry. Glob Ecol Biogeogr 24:371–382
Soudzilovskaia NA, van der Heijden MGA, Cornelissen JHC, Makarov MI, Onipchenko VG, Maslov MN et al (2015b) Quantitative assessment of the differential impacts of arbuscular and ectomycorrhiza on soil carbon cycling. New Phytol 208:280–293
Tedersoo L, Bahram M, Põlme S, Kõljalg U, Yorou NS, Wijesundera R et al (2014) Global diversity and geography of soil fungi. Science 346
Thuiller W, Pollock LJ, Gueguen M, Münkemüller T (2015) From species distributions to meta-communities. Ecol Lett 18:1321–1328
Treseder KK (2004) A meta-analysis of mycorrhizal responses to nitrogen, phosphorus, and atmospheric CO2 in field studies. New Phytol 164:347–355
Treseder KK (2016) Model behavior of arbuscular mycorrhizal fungi: predicting soil carbon dynamics under climate change. Botany 94:417–423
van der Heijden MGA, Boller T, Wiemken A, Sanders IR (1998a) Different arbuscular mycorrhizal fungal species are potential determinants of plant community structure. Ecology 79:2082–2091
van der Heijden MGA, Klironomos JN, Ursic M, Moutoglis P, Streitwolf-Engel R, Boller T et al (1998b) Mycorrhizal fungal diversity determines plant biodiversity, ecosystem variability and productivity. Nature 396:69–72
Vellend M (2010) Conceptual synthesis in community ecology. Q Rev Biol 85:183–206
Venette RC, Kriticos DJ, Magarey RD, Koch FH, Baker RHA, Worner SP et al (2010) Pest risk maps for invasive alien species: a roadmap for improvement. Bioscience 60:349–362
Vitousek P, Howarth R (1991) Nitrogen limitation on land and in the sea: how can it occur? Biogeochemistry 13:87–115
Willmott CJ, Rowe CM, Mintz Y (1985) Climatology of the terrestrial seasonal water cycle. J Climatol 5:589–606
Wisz MS, Pottier J, Kissling WD, Pellissier L, Lenoir J, Damgaard CF et al (2013) The role of biotic interactions in shaping distributions and realised assemblages of species: implications for species distribution modelling. Biol Rev 88:15–30
Wolda H (1981) Similarity indices, sample size and diversity. Oecologia 50:296–302
Xiang D, Verbruggen E, Hu Y, Veresoglou SD, Rillig MC, Zhou W et al (2014) Land use influences arbuscular mycorrhizal fungal communities in the farming–pastoral ecotone of northern China. New Phytol 204:968–978
Yackulic CB, Nichols JD, Reid J, Der R (2015) To predict the niche, model colonization and extinction. Ecology 96:16–23
Yang W, Zheng Y, Gao C, He X, Ding Q, Kim Y et al (2013a) The arbuscular mycorrhizal fungal community response to warming and grazing differs between soil and roots on the Qinghai-Tibetan plateau. PLoS One 8:e76447
Yang X, Post WM, Thornton PE, Jain A (2013b) The distribution of soil phosphorus for global biogeochemical modeling. Biogeosciences 10:2525–2537
Zhang T, Yang X, Guo R, Guo J (2016) Response of AM fungi spore population to elevated temperature and nitrogen addition and their influence on the plant community composition and productivity. Sci Rep 6:24749
Author information
Authors and Affiliations
Corresponding author
Editor information
Editors and Affiliations
Rights and permissions
Copyright information
© 2017 Springer International Publishing AG
About this chapter
Cite this chapter
Kivlin, S.N., Muscarella, R., Hawkes, C.V., Treseder, K.K. (2017). The Predictive Power of Ecological Niche Modeling for Global Arbuscular Mycorrhizal Fungal Biogeography. In: Tedersoo, L. (eds) Biogeography of Mycorrhizal Symbiosis. Ecological Studies, vol 230. Springer, Cham. https://doi.org/10.1007/978-3-319-56363-3_7
Download citation
DOI: https://doi.org/10.1007/978-3-319-56363-3_7
Published:
Publisher Name: Springer, Cham
Print ISBN: 978-3-319-56362-6
Online ISBN: 978-3-319-56363-3
eBook Packages: Biomedical and Life SciencesBiomedical and Life Sciences (R0)