Abstract
Concrete has been categorized as a key contributor of carbon emissions. This is mostly associated with the production of the ordinary portland cement. In response to this, the concrete and cement industries are pursuing a path to reduce their carbon emissions, generally in accordance with the climate change goals outlined in the Paris Agreement. These efforts have resulted in the development of multiple low-carbon concrete systems aimed to reduce the environmental impact of cement and concrete. However, these new low-carbon concrete systems may not always result in a more resilient structure, i.e., a more durable and longer-lasting structure. In fact, some research indicates that the service life of structures containing lower carbon producing alternative cementitious materials may be reduced based on their performance in corrosive conditions. However, reduced service life does not necessarily mean there are no carbon emission benefits from the new material. An objective assessment of the trade-off between improved sustainability (i.e., reduced carbon footprint) and reduced resilience (i.e., reduced service life) is needed. This paper presents a life-cycle assessment-system dynamics approach to evaluate the trade-off between more sustainable reinforced concrete solutions and the reduced resiliency of these systems over time. The objective of this paper is to assess the effect of improved sustainability and the potential reductions in service life as a result of corrosion deterioration for low-carbon concrete alternatives. This paper also aims to provide information to better understand how short-term sustainability decisions can influence the longer-term sustainability goals when designing and constructing with reinforced concrete.
Access provided by Autonomous University of Puebla. Download conference paper PDF
Similar content being viewed by others
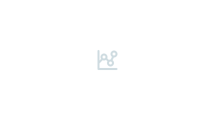
Keywords
- Sustainability
- Resiliency
- Reinforced Concrete
- Corrosion
- Alternative Cementitious Materials
- Service Life
- Life-Cycle Assessment
- System Dynamics
1 Introduction
1.1 Sustainability and Resiliency
Concrete has been categorized as a key contributor of carbon emissions. This is mostly associated with the production of the ordinary portland cement (OPC). The cement industry is considered to be a larger contributor accounting for approximately 8% of global carbon emissions [1]. In response to this, the concrete and cement industries are pursuing a path to reduce their carbon emissions, generally in accordance with the climate change goals outlined in the Paris Agreement [18]. These efforts have resulted in the development of multiple low-carbon concrete systems aimed to reduce the environmental impact of cement and concrete. However, these new low-carbon concrete systems may not always result in a more resilient structure, i.e., a more durable and longer-lasting structure. In fact, some research indicates that the service life (SL) of structures containing lower carbon producing alternative cementitious materials (ACMs) may be reduced based on their performance in corrosive conditions [3]. The use of purported low-carbon concrete systems could have unintended consequences. That is, the reduction in SL and resulting carbon emissions from the needed repairs or rebuilds could off-set the benefits of the lower initial carbon emissions. Even so, this should not strictly prevent the implementation of these systems. If significant reductions in initial carbon emissions can be realized when using low-carbon concrete systems, even with some reduction in service life (RSL) there could be value. A trade-off analysis is needed. This paper will assess the trade-off between sustainability and resiliency for these new low-carbon concrete systems through an innovative approach that combines the principles of system dynamics (SD), life-cycle assessment (LCA), and SL prediction into one model. This approach is herein referred to as the life-cycle assessment-system dynamics approach (LCA-SDA).
1.2 LCA-SDA
The LCA-SDA focuses on dynamically assessing the trade-off between sustainability and resiliency of new potentially low-carbon concrete systems that could exhibit a reduction in SL caused by chloride induced corrosion when used in reinforced concrete (RC) structures. Unlike static LCA, the approach presented herein uses a dynamic approach that allows for the implementation of discounting and inflation on carbon emissions. Static approaches do not discount or inflate carbon emissions [4]. It has been found that when alternatives have to be compared, discounting and inflation should be applied to better support decisions and generate more meaningful results [5]. Not discounting carbon emissions can underestimate the added-value of potentially low carbon-concrete alternatives when the social cost of carbon (SCC) is considered and there is a potential RSL. Thus, the LCA-SDA does allow for discounting of carbon emissions. Inflation, or the increase in SCC values over time, is also considered in the LCA-SDA model [6]. It is expected that the eventual implementation of this approach will allow design professionals, policy makers, and other stakeholders to better understand how their decisions regarding materials selection influence future carbon emissions and whether there is value in implementing these new solutions. Value here is defined as reduced carbon emissions from materials through the life cycle of the structure compared to a baseline (e.g., OPC).
2 LCA-SDA Model Development
The development of the model is divided into two main parts: the conceptual model development and the quantitative or LCA-SDA model development. The conceptual model is developed first. However, as the quantitative model is developed, the conceptual model is continuously updated; the process is iterative. Multiple versions of both models have been generated. Given that the models herein are in the initial stages of development, further development is anticipated. Thus, the model presented here should not be considered final and careful consideration should be taken when interpreting its results. The intent is to provide an initial approach for the readers to consider the impact of their decisions in terms of sustainability and resiliency. The model limitations and assumptions are presented later. This paper also addresses how future work will address these limitations and assumptions.
2.1 Conceptual Model Development
A conceptual model is developed to define the relationships and dependencies between the model components for the sustainability-resiliency trade-off system [7]. Overall, the conceptual model for the sustainability-resiliency trade-off system is made up of the sustainability (initial carbon), resiliency (in-service carbon), and design subsystems. The sustainability subsystem is then reduced into smaller sections: 1. The carbon from production section, and 2. The carbon from transportation section. The resiliency subsystem is also reduced into two sections: 1. The corrosion and SL section, and 2. The in-service carbon emissions section. Due to space constraints, refer to Moreno Rangel (2023) for more detailed information on this model [7].
2.2 Quantitative Model Development
A quantitative model is developed to quantify the multiple components of the sustainability-resiliency trade-off system presented in the conceptual model [7]. Again, due to space constraints, refer to Moreno Rangel (2023) for more detailed information on this model [7].
2.3 Data Collection
Data are a key element for the proper functioning of the model. The accuracy of the model outputs is directly related to the accuracy of the inputs coming from the data collection. The data collected for the model inputs comes from literature and from four main analyses: mixture proportioning, quantity take-off (QTO), LCA, and SL prediction.
2.3.1 Mixture Proportioning Data
Mixture proportioning data are a key component of the sustainability subsystem. Mixture proportioning data can be obtained by using a volumetric mixture proportioning method (e.g., ACI 211) [8] or from published literature. For the model herein, the mixture proportioning input data include the OPC proportion, the ACM proportion, the fine aggregate proportion, the coarse aggregate proportion, and the water proportion. The units of these terms are tonnes of constituent material per tonne of concrete. Mixture proportioning data must also include information on supplementary cementitious materials (SCMs), admixtures and other additives, if used in the mixture. These inputs are not considered in the current model. In the current model, ACMs are aluminate-based systems and are considered separately from SCMs such as fly ash, ground granulated blast-furnace slag (GGBFS), silica fume, and other SCMs.
2.3.2 Quantity Take-Off (QTO) Data
QTO data can be obtained using plans and specifications from the project. The amount of concrete on a project is a key input in quantifying how much carbon emissions will be emitted by the use of the materials in the project. The QTO data needed as inputs for this model include concrete and reinforcement required for the project (both in tonnes). Other materials, such as formwork (wood) can also be quantified and included but are currently not considered.
2.3.3 LCA Data
LCA data can be obtained from the output of LCA software or can be extracted from published literature. The LCA data to be inputted in this model needs to come from a cradle-to-gate LCA. For this model, a cradle-to-gate LCA will only consider the production process of the material and will not consider its transportation to any location outside of the production facility. The gate can be thought of as the gate of the production facility. The LCA data needs to be inputted as a carbon footprint unit weight (CFUW) for each material. CFUW refers to the amount of carbon that is emitted for every unit of the material being produced and has units of metric tons of carbon dioxide equivalent (MTCO2e) per unit of material (e.g., OPC in MTCO2e/tonne of OPC).Data from Sects. 2.3.1, 2.3.2, and 2.3.3 are used to calculate the initial carbon emissions, which is part of the sustainability subsystem within the sustainability-resiliency trade-off system.
2.3.4 SL Prediction Data
SL prediction data for the systems being analyzed can be obtained from literature or from SL software such as Life-365 [9]. The end of SL used in this model is assumed to occur as a result of chloride-induced corrosion. Other mechanisms of deterioration can be included but are not part of the model herein. The SL input data for the model herein includes time to corrosion (years) and time from corrosion initiation to initial repair (years). SL is the sum of these two inputs. The model uses these inputs to determine when repairs and rebuilds will be needed. In that regard, SL data are used to determine the in-service carbon emissions and is part of the resiliency subsystem within the sustainability-resiliency trade-off system.
2.3.5 Other Data
Other data needed for the model include transportation, repair, rebuild, SCC, and discount rate. Transportation data includes resource proximity (distance from plant to site), number of round trips (from plant to site), and fuel economy. Only the transport of concrete was considered in this study, but the inputs can be adjusted to include transportation for other materials. Transportation data forms part of the sustainability subsystem.Input repair data includes percent of SL increased per repair (how much SL is obtained per repair as a percentage of the initial SL), repairs allowed before rebuild (how many times can the structure be repaired before needing to be rebuilt), and percent area to repair. The percent area to repair for this model is assumed to be equivalent to the percent of initial carbon emissions produced by each repair. For example, if 5% of the structure is repaired, 5% of the initial carbon emissions will be produced by that repair. This assumption is included within the model and cannot be changed unless the model is modified. Future versions of the model will allow the carbon emissions emitted per repair to be an input selected by the user. Repair data are part of the resiliency subsystem.
The SCC data (in $/MTCO2e) are critical inputs for this model. The present SCC (present refers to 2023 in this model) and the future SCC at three different social damage discount rates (SDRs), 2.5%, 3%, and 5%, are used. The SCC and SDR data are obtained from the Interagency Working Group on Social Cost of Greenhouse Gases from the United States Government (USIWG) [6]. To obtain present SCC values for 2023 and future SCC values at each time of repair or rebuild, equations were fitted to the data provided in Table ES-1 of the USIWG document [6] for each SDR. The following equations are used to determine the
where Δt is the difference in years between 2020 and the future year for which the Future SCC ($/MTCO2e) is being obtained. The incorporation of these equations is key for the determining the SCC in future years. Note that the USIWG only provides data from 2020 to 2050 [6], so for any year after 2050, the values are extrapolated.
The SCC values from Eqs. 1 through 3 are then used to obtain the future value (FV) of the social cost of in-service carbon emissions (FVSCICE), which is defined as the product of the carbon emissions at the year of repair or rebuild (FV of in-service carbon emissions) and the SCC at that same year and at the selected SDR. The FVSCICE is then discounted to the present value (PV) of the social cost of in-service carbon emissions (PVSCICE) using Eq. (4).
where EDR is the emissions discount rate (%), n is the years from initial construction to the repair or rebuild, FVSCICE is the FV of the social cost of in-service carbon emissions ($), and PVSCICE is the PV of the social cost of in-service carbon emissions ($). Given that the SCC increases over time per Eqs. 1 through 3, the in-service carbon emissions are inflated when multiplied by the SCC. Thus, both discounting, per Eq. (4), and inflation, per Eqs. 1 through 3, of in-service carbon emissions are considered in this model.Note that there are two different types of discount rates in this model. Discount rates published by the USIWG [6] are herein referred to as SDRs and are used by the USIWG to obtain present SCC values by discounting the cost of future social damages caused by carbon emissions. Discount rates used in Eq. (4) to obtain the PV of the future social cost of in-service carbon emissions coming from repairs or rebuilds are herein referred to as EDRs. Higher SDRs for discounting cost of future social damages caused by carbon emissions (as used by the USIWG) would result in lower present SCCs and in turn would promote the generation of carbon emissions now rather than in the future. Higher EDRs for discounting cost of carbon emissions would result in lower PV for future carbon emissions that in turn would promote the generation of carbon emissions in the future rather than today. EDRs can be selected based on the financial situation of the user (e.g., minimum attractive rate of return) or in accordance with the published SDRs. Careful consideration should be taken when recommending discount rates as these rates have significant implications on carbon emissions. Figure 1 shows how the discounting process works within this model.In the current model, performing the analysis with a 3% discount rate (DR) means both that the SCC data came from a 3% SDR and that emissions are being discounted in the LCA-SDA model from a future value to a PV using Eq. (4) at a 3% EDR. These do not necessarily need to be the same. The SCC data could come from a 5% SDR and the emissions could be discounted at a 4% EDR. However, the current model and case study use the same value for both the SDR and the EDR as it is assumed that jurisdictions will also use the same value. Thus, throughout the paper, discount rates will be referred to as DRs (DR = SDR = EDR), unless a distinction between SDRs and EDRs needs to be made. The current model does not have an option to input SDR and EDR separately; it only allows for the input of DR. In future versions, these will be separate inputs.
2.4 Model Simulation and Outputs
The model is simulated over time within Insight Maker (SD software) to provide the user the ability to dynamically change the inputs and observe their effects on the behaviour of the system. Simulating systems over time is the basis of SD models [10]. The default simulation settings include years as the unit of time, simulation time steps of 1 year, and the Euler’s integration method as the simulation analysis algorithm. This means that outputs and other values within the model are calculated and plotted using the Euler’s method at 1-year intervals. The unit of time, time step, and algorithm can be adjusted depending on the needs of the project or system. The main two outputs of the model include the PV of carbon emissions generated by the system over time and the PV of the total carbon emissions generated by the system. The outputs generated from the model are dynamically plotted within the software to allow for a preliminary visual analysis and data check. For further or more advanced data processing, the user has the option to download the CSV files of the data generated by the model. Section 3 will provide an example case study application for the LCA-SDA model developed.
3 LCA-SDA Model Application – Example Case Study
The model presented in this paper was applied for the analysis of an example case study in which two different bridge deck systems were compared over time at different reductions in SL for the low-carbon concrete alternative, and at different discount rates. An OPC concrete bridge deck system is selected as the base case scenario and an ACM, specifically a calcium sulfoaluminate cement (CSA) bridge deck system, is chosen to be the low-carbon concrete alternative. Both systems were analyzed over a period of 55 years that included a maximum of 4 repairs for the OPC concrete structure. The design SL for the base case scenario (OPC system) was selected to be 31 years to assess the short-term impacts of the sustainability-resiliency trade-off. The SL of the CSA system varied in this assessment and included SL reductions from the OPC system of 0%, 10%, 20%, 30%, 40%, and 50%. Three DRs = were considered in this assessment: 2.5%, 3%, and 5%.The main two goals of this example case study are to demonstrate how the model is applied and to assess the sustainability-resiliency trade-off between the two systems. Figure 2 provides a flowchart summarizing the general process followed for the application of the LCA-SDA model. Section 3.1 provides information on what data are collected or selected to be included as inputs. Section 3.2 covers the simulation settings used and the model outputs, or results obtained from the sustainability-resiliency trade-off assessment.
3.1 Model Inputs
3.1.1 Mixture Proportioning Inputs
The mixture proportioning data used as inputs for this analysis are taken from the technical paper published by Cook and Murray [11]. Table 1 provides a summary of the mixture proportion quantities for the OPC and CSA systems. Cook and Murray roughly matched the proportions of the CSA system to those of the OPC system and used a different water to cement ratio (w/c) for each system to achieve a similar flexural and compressive strength. A 0.40 w/c was used for the OPC system, and a 0.48 w/c was used for the CSA system. A higher w/c was used for the CSA system to ensure complete hydration [11].
3.1.2 QTO Inputs
3.1.3 LCA Inputs
The LCA data collected for this analysis are obtained from various published sources which focused on LCA of concrete systems. Table 3 provides a summary of the CFUW for each product or process along with the sources from which the data are obtained.
3.1.4 SL Prediction Inputs
SL predictions are obtained using Life-365 v2.2.3 [9]. Table 4 shows the parameters that were selected within Life-365 for the calculation of the SL of the OPC system. An aggressive environment was purposely selected within Life-365 to evaluate the short-term effects of the sustainability-resiliency trade-off.
Based on the Life-365 output, the SL of the OPC system is 31 years with 25 years for corrosion initiation and 6 years for propagation. Six different SLs were considered for the CSA system: 31 years, 27.9 years, 24.8 years, 21.7 years, 18.6 years, and 15.5 years, respectively.
3.1.5 Other Inputs
Other required inputs for this case study included transportation, repair, rebuild, and SCC. Transportation data for this analysis are only considered for the transport of concrete and are assumed to be the same for both OPC and CSA systems. Future analyses will consider the transportation of all resources. The fuel economy input was obtained from the Alternative Fuels Data Center [24]. Repair and rebuild input data are set by the user based on experience.
3.2 Model Simulation and Outputs (Results)
The model is simulated using the default Insight Maker simulation settings. This means that the model was run at 1-year time steps using the Euler’s method as the simulation algorithm.
The output data from each simulation were initially visually analyzed within Insight Maker and then exported as a CSV file into a spreadsheet for further analysis.
Figure 3(a) presents information regarding the PV of carbon emissions at a 3% DR for the two systems as obtained directly from the model output before correcting for unused carbon emissions resulting from the remaining SL of the rebuilds or repairs at the end of the 55-year analysis period. Unused carbon emissions are associated with the remaining SL of the structure beyond the analysis period.Careful consideration should be taken when analysing the results shown in Fig. 3(a) as they consider all carbon beyond the analysis period. Making decisions based on Fig. 3(a) can underestimate the potential benefits of low-carbon concrete alternatives that exhibit reduced service lives when the carbon emissions are not corrected for unused carbon emissions. When alternatives with unequal lives are compared and an alternative’s life is longer than the analysis period, there is some remaining value at the end of the analysis period and adjustments to the analysis must be made accordingly [26]. The remaining value in this analysis is in terms of invested carbon emissions that have not been used (referred herein as unused carbon emissions) instead of invested money that has not been used as it is typical in a life-cycle cost and economic analysis.
For example, the CSA concrete option with 20% reduction in SL (24.8 years) will have an initial construction, 4 repairs, and will have to be rebuilt at 44.6 years (compared to the 55 years for the OPC option, which is the analysis period). For this case, the proportion of SL for the rebuild up to year 55 is used as a factor to subtract the unused carbon emissions. For the 20% SL reduction case, 10.4 years of the rebuild are used up to the end of the analysis period and therefore only https://doi.org/10.4/24.8 (41.9%) of the carbon emissions coming from the rebuild should be included in the analysis. Unused carbon emissions coming from a repair can also subtracted. Figure 3(b) shows the comparison of PV of carbon emissions for CSA and OPC after correcting for unused carbon emissions. CSA 20% RSL was corrected for unused carbon emissions from a rebuild and CSA 40% RSL was corrected for unused carbon emissions from a repair. By comparing Figs. 3(a) and 3(b) for these two scenarios, it can be noted that the effect of correcting for unused carbon emissions from a rebuild is more significant than the effect of correcting for unused carbon emissions from a repair. No correction was needed for the CSA 0% RSL and the OPC Baseline as the service life of the fourth repair ends at the end of the 55-year analysis period for both.
The influence of the discount rate on the PV of carbon emissions is shown in Fig. 4. Note that the values for Fig. 4 are not corrected for unused carbon emissions as the figure only shows the OPC option. It can be seen in Fig. 4 that as the discount rate increases, the PV of carbon emissions decreases.
It is important to note that the PV is sensitive to the DR. Different DRs will result in different outcomes. These DR values are generally defined by governmental administrations and organizations. Different administrations use different discount rates to obtain the SCC data. In fact, different organizations within the same administration can use different DR values. The definition of DR values seems to be politicized and fluid.
The example case study presented herein assesses two different systems (OPC and CSA) at a 2.5, 3, and 5% DR. A DR of 3% is the value currently being used by the current administration in the US [6, 25]. Figure 5 shows the trade-off between sustainability and resiliency for a CSA system in terms of the change in PV of total carbon emissions (CCE) from that of the OPC systemas a function of the percent RSL from that of the OPC system. Note that as the DR increases, the percent RSL at the breakeven point increases. The breakeven point is the intersection of the 0% CCE line and the CSA curve at the selected DR. In Fig. 5, these breakeven points occur at percent RSLs of approximately 22%, 23%, and 28% for the 2.5%, 3.0%, and 5% DR values respectively. It should be noted that the data used to generate Fig. 5 has been corrected for unused carbon emissions.
Figure 5 shows that at a 3% DR, there is no value in implementing the low-carbon concrete alternative if that alternative (in this case, the CSA) reduces the SL by 23% (23% RSL) or more (i.e., the breakeven point). Thus, the material should not reduce the SL by more than 23% as this would result in an increase in carbon emissions over the analysis period. Lower RSLs result in higher carbon savings. Up to this point we have identified a potential low-carbon concrete material and quantified its CCE for several potential RSLs. As we know the potential low-carbon material that will be used, we can now assess the actual SL of the low-carbon concrete system and determine if there is value, i.e., overall reduced carbon emissions.
If it is assumed that the RSL for CSA is 10% from the OPC system, it can be seen from Fig. 5 that the CCE is approximately -15%. The CCE and the RSL, which can together be referred to as the sustainability-resiliency trade-off, would be -15% CCE: 10% RSL. The negative sign on the CCE of this sustainability-resiliency trade-off indicates a reduction in carbon emissions and that the implementation of the low-carbon concrete alternative (CSA) is value adding. However, the RSL of 10% has only been assumed.
The actual RSL of the low-carbon concrete alternative (CSA system) can be determined using SL prediction tools such as Life-365 [9] or SL prediction models published in the literature. Furthering the earlier example, SL prediction results reported by Dev Vasudevan and Trejo [3] can be used. Dev Vasudevan and Trejo used Life-365 to predict the SL of both OPC and CSA systems and reported that CSA systems could reduce the SL by approximately 42% (42% RSL) when compared to OPC systems [3]. In this case, based on Fig. 5, there would be a + 28% CCE: 42% RSL. This indicates that a 42% RSL results in a + 28% change in carbon emissions; the positive sign on the CCE indicates an increase in carbon emissions and that the implementation of the potential low-carbon concrete alternative (CSA) is non-value adding.
A clarification and disclaimer are needed here. Dev Vasudevan and Trejo provided results for 0.42 and 0.485 w/c for the OPC system and w/c values of 0.35, 0.45, and 0.55 for the CSA system. The mixture proportion used in the example case study presented in this paper uses a w/c of 0.40 for the OPC system and a w/c of 0.48 for the CSA system. The 42% RSL for this work was determined by comparing the SL of the 0.42 w/c OPC system with the average SL of the 0.45 w/c and 0.55 w/c CSA systems from the Dev Vasudevan and Trejo results [3]. It should also be noted that Life365 was used by the authors to determine SL and the Life365 program was developed for systems containing OPC [9]; the applicability to CSA systems is unknown.
It has been shown that the model developed herein can quantify the sustainability-resiliency trade-off for materials that, when manufactured, are purported to be “low-carbon” concrete alternatives or “sustainable” due to their reduced carbon emissions during their production. However, if the new materials result in a reduced life, the trade-off analysis between the sustainability and resiliency developed herein should be performed.
Assumptions and potential limitations of the work are addressed next.
4 Model Assumptions and Limitations
The current model is considered a starting point for the assessment of the sustainability-resiliency trade-off as it still contains multiple assumptions and limitations that need to be tested and/or corrected. The assumptions for the model and case study include the following:
Assumption 1:
All data for the LCA considers only the carbon emissions coming from the production of the materials. This assumes the construction methods for both systems are the same.
Assumption 2:
Carbon emissions produced per repair are a percentage of the initial carbon emissions equivalent to the area to be repaired; for example, if 5% of the bridge deck area is repaired, 5% of the initial carbon emissions will be emitted. Carbon emissions from rebuilds are assumed to be the same as the initial carbon emissions.
Assumption 3:
The propagation phase for the time to first repair is 6 years for all alternatives.
Assumption 4:
Five percent of the deck area will be repaired after the end of the SL and the end of each consecutive repair, and each repair will increase the SL by 20% of the initial SL.
The limitations of the current model and case study include the following:
Limitation 1:
The current model and case study only consider the impact of carbon emissions coming from the production of the materials, the transportation of concrete, and repairs or rebuilds as a function of the initial materials production and concrete transportation emissions. It does not include emissions coming from the construction process, waste disposal, recycling, or the transportation of materials, other than concrete, to the site or concrete plant.
Limitation 2:
The current model does not include inputs for analyzing the effects of admixtures, SCMs, and other concrete constituent materials on the sustainability-resiliency trade-off. It also does not include inputs for other construction materials such as wood for formwork, curing compounds, etc.
Limitation 3:
The current model can perform assessments up to a 100-year analysis period and the correction for unused carbon emissions coming from the remaining SL of rebuilds or repairs needs to be performed separately.
Limitation 4:
The current model only allows for the input of DR, which does not allow for SDR and EDR to be inputted separately (i.e., EDR = SDR = DR).Future work on further developing the model and its application on real case studies is on-going and is expected to reduce the number of assumptions and to address most of the limitations presented herein.
5 Conclusions and Future Work
The LCA-SDA model developed can objectively assess the trade-off between purported sustainability and resiliency of RC subjected to corrosive conditions. The model output provides designers with an objective assessment of overall carbon footprint and potential value of using such materials. Just because a new material exhibits a lower carbon footprint during manufacturing does not necessarily mean that the constructed system will exhibit a lower carbon footprint. The resulting SL of implementing the new material is critical. The incorporation of SD allows for the modeling of the dynamic interaction between sustainability and resiliency over time through the concept of in-service carbon emissions resulting from repairs or rebuilds paired with the discounting process. This dynamic model accounts for the limitations of static models that do not discount or inflate carbon emissions. This work indicates that the model is sensitive to the selected DR, and this can influence the outcome; in general, DR values are determined by governmental agencies and administrations. The results from this model can be used to supplement other analyses, such as life-cycle cost analysis (LCCA). Note that this is the first version of the model and results presented herein demonstrate the applicability of the LCA-SDA model for assessing the sustainability-resiliency trade-off. Future work will focus on further developing this model and addressing the limitations and assumptions presented in this study. Its application on real case studies and a sensitivity analysis on its inputs is also expected in future work.
References
Sharma, A., Basavaraj, A.S., Chaunsali, P., Gettu, R.: Calcium sulfoaluminate cement manufacturing in India— prospects and prognosis of environmental impacts. ACI Mater. J. 120(1), 17–28 (2023)
IPCC. Summary for Policymakers. In Global Warming of 1.5°C: IPCC Special Report on Impacts of Global Warming of 1.5°C above Pre-industrial Levels in Context of Strengthening Response to Climate Change, Sustainable Development, and Efforts to Eradicate Poverty (pp. 1–24). Cambridge: Cambridge University Press (2022)
Dev Vasudevan, G., Trejo, D.: Assessing the critical chloride threshold of conventional reinforcement embedded in alternate cementitious systems. Construct. Build. Mater., 346 (2022)
Müller, L.J., Kätelhön, A., Bachmann, M., Zimmermann, A., Sternberg, A., Bardow, A.: A guideline for life cycle assessment of carbon capture and utilization. Front. Energy Res., 8 (2020)
Lueddeckens, S., Saling, P., Guenther, E.: Discounting and life cycle assessment: a distorting measure in assessments, a reasonable instrument for decisions. Int. J. Environ. Sci. Technol. 19(4), 2961–2972 (2021)
Interagency Working Group on Social Cost of Greenhouse Gases, United States Government. Technical Support Document: Social Cost of Carbon, Methane, and Nitrous Oxide Interim Estimates under Executive Order 13990. The White House (2021)
Moreno Rangel, E.D.: (thesis) Assessing the Trade-Off Between Sustainability and Resiliency of Reinforced Concrete Alternatives in Corrosive Conditions: An LCA-System Dynamics Approach. Oregon State University (2023)
ACI Committee 211: ACI PRC-211.1–22: Selecting Proportions for Normal-Density and High Density-Concrete – Guide (pp. 1–38). American Concrete Institute (2022)
Life-365 Consortium III. Life-365 v2.2.3 User’s Manual (pp. 1–87), The Silica Fume Association (2013)
System dynamics. Insight Maker (n.d.) (2023). https://insightmaker.com/docs/systemdynamics
Cook, G.W., Murray, C.D.: Behavior of reinforced concrete made with belitic calcium sulfoaluminate cement at early ages. ACI Mater. J., 117(1) (2020)
Zib, L., Byrne, D.M., Marston, L.T., Chini, C.M.: Operational carbon footprint of the U.S. water and wastewater sector’s energy consumption. J. Clean. Prod., 321 (2021)
Kelechi, S.E., Adamu, M., Mohammed, A., Obianyo, I.I., Ibrahim, Y.E., Alanazi, H.: Equivalent CO2 emission and cost analysis of green self-compacting rubberized concrete. Sustainability 14(1), 137–151 (2021)
Jiménez, L.F., Domínguez, J.A., Vega-Azamar, R.E.: Carbon footprint of recycled aggregate concrete. Adv. Civil Eng. 2018, 1–6 (2018)
Fayomi, G.U., Mini, S.E., Fayomi, O.S., Ayoola, A.A.: Perspectives on environmental CO2 emission and energy factor in cement industry. IOP Conf. Ser.: Earth Environ. Sci. 331(1) (2019)
Naqi, A., Jang, J.: Recent progress in green cement technology utilizing low-carbon emission fuels and raw materials: a review. Sustainability 11(2), 537–555 (2019)
Nie, S., et al.: Analysis of theoretical carbon dioxide emissions from cement production: methodology and application. J. Clean. Prod., 334 (2022)
Tao, Y., Rahul, A.V., Mohan, M.K., De Schutter, G., Van Tittelboom, K.: Recent progress and technical challenges in using calcium sulfoaluminate (CSA) cement. Cement and Concrete Composites, 137 (2023)
Alapati, P., Kurtis, K.E.: Carbonation in alternative cementitious materials: Implications on durability and mechanical properties. In: Sixth International Conference on Durability of Concrete Structures. University of Leeds, Leeds, West Yorkshire, United Kingdom (2018)
Chaunsali, P., Mondal, P.: Influence of calcium sulfoaluminate (CSA) cement content on expansion and hydration behavior of various ordinary Portland cement-CSA blends. J. Am. Ceram. Soc. 98(8), 2617–2624 (2015)
Re-Steel Supply Co, Inc. Corporate. EPD of Re-Steel’s carbon-steel and low-alloy uncoated rebar products. ASTM Int., 1–17 (2021)
Marceau, M.L., Nisbet, M.A., VanGeem, M.G.: Life cycle inventory of Portland cement concrete, SN3011. Portland Cement Assoc., 1–121 (2007)
U.S. Environmental Protection Agency. Emission factors for greenhouse gas inventories (2023). https://www.epa.gov/system/files/documents/2023-03/ghg_emission_factors_hub.pdf
Average Fuel Economy by Major Vehicle Category. alternative fuels data center: maps and data (2023). https://afdc.energy.gov/data
Prest, B.C., Pizer, W.A., Newell, R.G.: Improving discounting in the social cost of carbon. Working Toward New Soc. Cost Carbon 208, 12–15 (2021)
Fabrycky, W.J., Blanchard, B.S.: Life-Cycle Cost and Economic analysis. Prentice Hall, Saddle River (1991)
Acknowledgments
The authors would like to acknowledge the many great contributions of Professor Ravindra Gettu to the RILEM and the concrete industry and would also like to thank the organizers of the 2023 RILEM Week/ISSSI and organizers of the Ravindra Gettu Honorary Session for all of their work in making these events possible. Professor Gettu has made outstanding and significant contributions to the science, engineering, and technology of cementitious systems.
Author information
Authors and Affiliations
Corresponding author
Editor information
Editors and Affiliations
Rights and permissions
Copyright information
© 2024 The Author(s), under exclusive license to Springer Nature Switzerland AG
About this paper
Cite this paper
Moreno Rangel, E.D., Trejo, D. (2024). Assessing the Trade-Off Between Sustainability and Resiliency of Reinforced Concrete in Corrosive Conditions: An LCA-System Dynamics Approach. In: Banthia, N., Soleimani-Dashtaki, S., Mindess, S. (eds) Smart & Sustainable Infrastructure: Building a Greener Tomorrow. ISSSI 2023. RILEM Bookseries, vol 48. Springer, Cham. https://doi.org/10.1007/978-3-031-53389-1_42
Download citation
DOI: https://doi.org/10.1007/978-3-031-53389-1_42
Published:
Publisher Name: Springer, Cham
Print ISBN: 978-3-031-53388-4
Online ISBN: 978-3-031-53389-1
eBook Packages: EngineeringEngineering (R0)