Abstract
This paper investigates computationally the following research hypotheses: (1) Higher flexibility and discretion in organizational culture results in better mistake management and thus better organizational learning, (2) Effective organizational learning requires a transformational leader to have both high social and formal status and consistency, and (3) Company culture and leader’s behavior must align for the best learning effects. Computational simulations of the introduced adaptive network were analyzed in different contexts varying in organization culture and leader characteristics. Statistical analysis results proved to be significant and supported the research hypotheses. Ultimately, this paper provides insight into how organizations that foster a mistake-tolerant attitude in alignment with the leader, can result in significantly better organizational learning on a team and individual level.
Access provided by Autonomous University of Puebla. Download conference paper PDF
Similar content being viewed by others
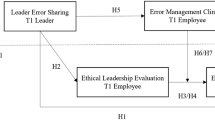
Keywords
- Mistake management
- Hebbian learning
- Organizational learning
- Vicarious learning
- Organizational culture
- Transformational leadership
1 Introduction
Committing mistakes in a professional field is usually perceived as a complete aberration. It is often associated with adverse feelings of guilt, embarrassment, and even lower self-esteem. However, mistakes are an inevitable aspect of an organization whether it acknowledges it or not. Although, the organization’s conception and reaction to making mistakes can affect its long-term learning and performance [6]. Tacit beliefs of individuals regarding committing mistakes are often a by-product of the environment, including the organization’s culture and leader’s attitudes [13]. It has been empirically established that mistake tolerance aid organizational learning and as a result its performance [23]. A mistake non-tolerant environment makes it difficult to report mistakes and that often perpetuates the mistake and harms an organization’s long-term capacity to learn and improve. Whereas a mistake-tolerant environment is warier of long-term repercussions and less concerned about the immediate costs of the mistake. This attitude toward managing mistakes not only develops a healthier psychological response to mistakes but also promotes deliberate experimentation and critical analysis, as opposed to hiding mistakes and trying to move past them swiftly [6].
An organization’s culture, in addition to a leader’s behavior regarding mistake tolerance, is crucial to organizational learning [13, 14]. The idea, however, is not to cultivate an environment that encourages making mistakes, but one that encourages ‘fail intelligently’ to maximize the learning value of mistakes and promote intelligent risk-taking [23]. In essence, this paper aims to carry out computational simulations to explore the effect on organizational learning twofold: in relation to the organizations’ culture and leader’s behavior, and in relation to mistake management which is influenced by the former.
2 Background Literature
This section provides background literature revolving around the relevant concepts and acts as a basis for the experimental simulations in the paper.
The company culture is a shared mindset or as Hofstede et al. [9] says a shared “software of the mind”, that strongly determines the perception of company values, employees’ attitudes, beliefs, social norms, and behaviors. Schein [17] distinguished three fundamental levels of organizational culture consisting of (1) observable artefacts such as physical layout, the dress code, the manner in which people address each other; (2) values such as organizational norms and believes manifesting in people’s behaviors; and (3) basic underlying assumptions, least apparent and based on an organization’s historical events that determine perceptions, feelings and behaviour. Organizational culture is typically evaluated based on organizational values that organizational members have in common.
Cameron and Quinn [2] identified the key competing company values framework determining company culture (See Fig. 1). According to this framework, organizational culture can be derived from a grid of four quadrants that vary in two dimensions. The first-dimension ranges from flexibility and discretion on one end, to stability and control on the other end. This dimension focuses on the distinction of flexibility which values individuality versus stability which values top-down control. The second-dimension ranges from external focus and differentiation on one end, to internal focus and integration on the other end. This dimension addresses whether an organization values progress within the organization (internal focus), versus progress in juxtaposition to other organizations or competitors (external focus). This is also sometimes referred to as the external and internal positioning of an organization respectively. The four types of cultures that arise from these dimensions are clan, adhocracy, hierarchy, and market.
Leaders have a powerful influence over the organization’s environment and indirectly on individuals’ behavior. Given certain leadership qualities, leaders can foster an environment that facilitates multilevel learning and institutionalization of desired behaviors. Leaders who empower their employees manifest better results. Certain leadership practices, such as delegation, coaching, and recognition, are complementary Leadership empowerment behaviors (LEB) and fulfill Lawler’s [15] four basic conditions of empowerment [7]. All three of these practices can encourage employees to more improvement and feedback which can manifest better results.
Moreover, delegating tasks to employees can improve task reproduction and also collaboration—team learning. Similarly, coaching behavior guides the employees more and has the same effect as a delegation. Finally, leader recognition empowers employees and improves reinforcement [7]. Furthermore, transformational leaders can contribute significantly to better organizational learning [13]. Next to professional skills, such attributes as social status and consistency in the behavior of the leader support their transformational power. A leader’s status comprises both social status and formal organizational status. Having both types of status high can enhance the view and attention to the leader and improve vicarious learning. High social status is also important to captivate learners to capture attention to the desired learned behavior. Further, it is important that the leader acts consistently in order to increase the chance of employees memorizing behavior patterns via mirroring [15]. The assumptions and choices made for the computational model were mainly based on the already mentioned literature and [16, 18].
Organizational learning is a dynamic multi-level cyclical process that has learning flows directed from individual or team level to organizational level and vice versa. There are two learning flows in an organization: feed forward and feedback directions [8, 24]. Feedback learning is a common method where the learner receives information from the organization for improving ensuing behaviors in relation to the present performance. Contrastingly, feed-forward learning focuses on the organization receiving input from the learner or employee to improve future-oriented solutions or options. The multilevel learning theory introduced by Crossan et al. [8] is an interdependent theory suggesting learning flows are not necessarily linearly, from micro-level individuals to team level–known as meso-level—to systematic organizational levels–known as macro-level [24]. The learning from micro and meso levels to macro levels is categorized as feed-forward learning, whereas feedback learning occurs from macro to meso and micro levels, when individuals and teams learn from the organization.
There are numerous of learning theories, but one of the most renowned theories is the social learning theory. The theory is based on vicarious learning—also known as observational learning—where the individual learns simply by observing and imitating a model person’s behaviors; this process is often referred to as mirroring the model. Vicarious learning has three distinct stages [1]. The first stage is the observational phase where the learner requires attention to observe the model in order to memorize the pattern of behavior—a process referred to as retention. The second stage is the imitation phase where the learner mirrors the model and reproduces the desired behavior, which can be strengthened by reinforcement. Reinforcement is a process that rewards proper reproduction and enhances learning. The final stage is the participation phase where the behavior is fully learned. These intricate learning stages can be modeled in an organizational context and adapted according to the existing culture and the behavior of the leader—model.
The neurophysiological basis of vicarious learning can be elucidated by understanding mirror neurons and learning processes in the brain. Mirror neuron activations in the brain extend to vicarious activations in the somatosensory cortex which is in the same region mirror neurons are located [10]. The presence of such activations during vicarious learning could be partially explained by the Hebbian learning theory.
Hebbian learning comes from Donald Hebb’s theory which provides a neurophysiological account of learning and plasticity. The core concept of Hebbian learning emphasizes how one neuron that is continuously and persistently involved in firing a signal to another neuron elicits metabolic changes in one or both neurons. Hebbian learning mainly takes into account the causality of the neuron firing, indicating temporal precedence, but also contingency as the activity of the presynaptic neuron can be indicative of that of the postsynaptic activity [11]. The essence of temporal precedence in Hebb’s learning theory can be translated to temporal-causal networks in an organizational context.
Based on the existing insights from the organizational culture, leadership and organizational learning literature, we argue that when organizational culture is in alignment with the leader it then manifests presumptions on mistakes, making individuals either try to cover up their mistakes or exploit the learning value and alter the shared mental model of the organization to learn from it. For example, when cultural values are focused on employee involvement, experimentation, learning from mistakes and favouring change and at the same time when the leader aligns with these values by modelling relevant culturally aligned behaviours, their subordinates are more likely to reveal their shortcomings and learn from them. Conversely, when the culture and the leadership style promote values and behaviours that discourage talking about and sharing mistake, employees are more likely to hide their shortcomings, resulting in not learning.
This paper aims to utilize adaptive network modeling to computationally demonstrate how mistake-tolerant organizations learn better when using mistake management as a source of learning. Ultimately, the research paper explores the effect of culture and leader characteristics on mistake management and individual and team learning. The paper aims to utilize- in silico- experiments and statistically compare the results to investigate the following proposed research hypotheses:
-
Hypothesis 1: Higher flexibility and discretion in organizational culture results in better mistake management and thus better organizational learning.
-
Hypothesis 2: Effective organizational learning requires a transformational leader to have both high social and formal status and consistency.
-
Hypothesis 3: Company culture and leader’s behavior must align for the best learning effects.
3 Methodology: Self-Modeling Networks
This section delves into more detail about the network modeling approach along with the computational mechanisms behind it, in addition to the motives for the final adaptive network model used to run the simulations.
Network-oriented modeling can be used to better understand a myriad of social, cognitive, and biological phenomena. By graphically conceptualizing a complex process, the modeling approach acts as a basis of the conceptualization by representing the dynamic processes without separating or isolating different states. Temporal-causal networks specifically, incorporate the timing of dynamic or cyclical processes based on a continuous-time temporal dimension to time causal effects [19,20,21]. This network-oriented modeling approach has shown to be an appropriate method for modeling a complex phenomenon such as handling mental models in mental processes [22] and organizational learning and the roles of different contextual factors; e.g., [3,4,5].
Organizational learning [8, 12, 24] is a non-linear process where causal relations underlying knowledge can change over time. This has been modeled in [3,4,5] by adaptive reified network models, also referred to as self-modeling networks [21]. To elaborate, a complex process can be represented conceptually as a base-level network, but to add adaptivity of some of its network characteristics to the model, a self-model representing these network characteristics can adapt the network structure of the base-level network during the process. This next-higher level in a network for a base-level network is called a first-order self-model level. Along the same line, a second-order self-model level can be added to make the first-order self-modeling level model adaptive as well; this enables control of adaptation. Different context factors can influence higher-level nodes, such that a high extent of context-sensitivity of base processes and also of adaptation processes can be modeled.
In the current paper, a second-order adaptive self-modeling network model is used to conduct different simulation experiments (Sect. 4). The aim is to demonstrate the effect of the independent variables: organizational culture and leader’s consistency and status, on the dependent variables: mistake management and organizational learning. A temporal-causal network takes into account the following characteristics:
-
Connectivity characteristics: connections from a state X to a state Y have a connection weight value, represented by ωX,Y which indicates the strength of the connection.
-
Timing characteristics: for all states Y, a speed factor represented by ηY indicates how fast the state is changing upon a causal impact.
-
Aggregation characteristics: for all Y states, a combination function represented by cY(..) is referred to; it determines the way in which the causal impacts from other states combine for state Y; see Table 1 for them.
A dedicated software environment based on these network characteristics is utilized to generate the simulation output; see Chap. 9 of [20]. An overview of the role matrices description can be found in Sect. 2 of the Appendix (see the Linked Data at https://www.researchgate.net/publication/361481262. Based on the background literature provided, a generalized conceptual model (see Fig. 2) was first constructed to form a basis for the final self-modeling network representation used. This section discusses key decisions in the formation of the self-modeling network model. The concepts discussed in the background literature are translated into a computational network model using the software indicated above.
The basis of this model lies in the variation of culture types of organizations. This notion is captured by modeling context factors that affect the flexibility and focus in ways that align with the different culture types. The focus of the company is discussed in terms of positioning, where internally focused organizations have low positioning and externally focused organizations have high positioning. Each one of the four culture context factors has varying connection weights to the flexibility and positioning, allowing to model different types of cultures without manually altering the values of flexibility and positioning initially.
Further, leader traits were considered in relation to the existing culture so that scenarios would not increase in complexity of the possible variations; as discussed in Sect. 2, the main inspiration for modelling choices and assumptions made has been based on [7, 15, 16, 18]. Table 2 below details how the context factors, when high, influence the culture and therefore leadership empowerment characteristics and openness in relation to the existing culture.
Other leader qualities such as consistency and status were modeled independently of the culture. Consistency was a simple abstraction modeled through one state with the initial value varying in different simulations. Contrastingly, status varies across two dimensions where both social and formal organizational status are considered important in leader behavior. In this model both these concepts were considered as one state, that is stat_L. In scenarios where high status is modeled, the social and formal organizational status can be determined by the according culture type.
The final model constructed comprises 81 different states, an overview of all states with their according level can be seen in the table in Sect. 5 of the Appendix (Linked Data at https://www.researchgate.net/publication/361481262). The second-order adaptive network model is depicted in Fig. 3 below with the key components highlighted in Table 3.
The base model represents two individual mental models along with a leader and a shared mental model, the individuals interact with the leader periodically and learn a set of tasks (a, b, c). The individual and team learning is influenced by the organizational culture and mistake management. The model was also constructed in a way in which context factors facilitate organizational culture change over time, specifically transitions from one culture to another. An overview of the key stages of the designed network are summarized below:
Individual mental models: Oval encapsulations in the base plane represent mental models of certain tasks. Individuals A and B have additional inputs from world observation states that can alter their mental model and reproduction of the tasks. These states are a result of observations and mirroring the leader’s mental model combined with Hebbian learning which involves the W-states in the middle of the middle plane.
Shared mental model: Represented by the parallelogram encapsulation and is formed by taking input indirectly from individual learning of A and B in by connections between W-states in the first-order self-model level (middle) plane: feed forward learning. It occurs by transfer of knowledge from individual learning states (weight states) to shared learning weight states which directly affect the base-level shared mental model. In turn, individuals can learn from a shared mental model by connections between W-states in the opposite direction in the middle plane: feedback learning.
Mistake Handling: The dotted oval represents impartial knowledge for task c. This has a negative weight on mistake recognition (mistM1) so that if both b and c task states are high mistake noticing will be low. Mistake management (mistM2) depends on the mistake noticing state and organizational culture.
Leader interactions: Two interaction rounds of leader and individuals regarding each task takes place. All interaction states influence the attention of individuals and thus attention to observations states in the first-order self-model level.
Leader Qualities: All leader qualities (status, consistency, openness, recognition, and delegation) are represented individually. Recognition state in base-level influences persistence of Hebbian learning (in second-order level) as it reproduces the same effect of strengthening learning.
Attention: World observations along with interactions affect the base-level attention state. The attention base-level state indirectly influences individual’s mental model by affecting attention first-order level weight states. First-order level weight states comprise attention to leader interactions and world observations. Leader attention weight states are influenced by status and consistency of the leader.
Organizational culture: Adaptiveness of culture is modelled by weight factors in first-order plane which are influenced by the context factors. The thick red lines represent four downwards connections, representing influences from each context factor.
Individual learning: Hebbian learning in first-order self-model plane takes place by weight states that influence the connections between mental model components in the base-level. The learning quality is also influenced by second-order states.
Learning quality: Persistence in the second-order self-model plane influence learning forgetfulness and learning rate; lower persistence values means more forgetfulness.
Learning flows: Feed forward and feedback learning control in the second-order self-model plane influences shared and individual weight states respectively in first-level self-model plane. They are affected by context states in second-order self-model plane which control timing of different learning flows. Thick pink lines from the WW feedforward state represent two downwards connections from individual learning weight states.
Through variation of settings of the model described above, it was possible to generate 24 different scenarios that model the 4 types of culture with constant factors as a baseline, and for each type of culture, additional simulations will vary in consistency and status of the leader. An overview of all simulated scenarios is highlighted in Table 4.
4 Simulation Results
This section displays graphs for the simulation results of some of the scenarios. The graphs display a gradual increasing effect of learning and mistake management increasing as the flexibility of the organization increases and its positioning decreases. Prior to displaying the effect of culture on mistake management and learning. Temporal context will be given in terms of the learning flows and interactions. As indicated in Fig. 1 of Sect. 3 of the Appendix (see https://www.researchgate.net/publication/361481262), there are two rounds of the individual and leader interactions followed by feed forward learning with feedback learning after.
As seen in Fig. 4, interaction time extends from time point 50 to 150, which is followed by feed forward and feedback learning. The team learning peaks are aligned with the time of feed forward learning. That is because feed forward learning involves learning flows from micro-level (individual) to meso-level (team). Whereas individual learning does not only depend on the timing of feedback learning flows and is consistently higher because individuals can also learn from their environment, i.e. via world observations. Figure 4 shows the effect of flexible culture types on mistake acceptance and organizational learning.
Figure 5 displays the effect of less flexible cultures on the dependent variables when both consistency and status are low. In comparison to Figs. 2 and 3 of Sect. 3 of the Appendix, the same culture types when consistency and status are high display a big impact on individual learning and mistake management. Team learning on the other hand displays a smaller deviation where consistency and status of the leader are high. The most noticeable difference in team learning values was depicted in the hierarchy culture type.
Further, Sect. 3 in the Appendix displays the effect of the culture type on the dependent variables when consistency is low and status is high. These results are identical when status is low and consistency is high. This supports the notion that both status and consistency play an equally important role in organizational learning and mistake tolerance. Figure 5 displays the simulation where hierarchical culture transitions to clan culture. The graph is in line with the expected results as the transition to a culture with more flexibility has a positive correlation on the dependent variables. The hierarchy culture exists from the beginning till time point 450, whereas clan begins from that point on. Statistical analysis was carried out to compare the 24 scenarios according to the culture type and leader characteristics. The numerical simulation output was measured for the three dependent variables considered: individual learning, team learning, and mistake management. Since the scenarios only vary in culture and leader behavior, the simulations reproduce consistent mistake management, individual and team learning variances. Thus, the simulations were seen as one population that assumes homoscedasticity, that is equal variances among the dependent variables. Moreover, since each simulation is independent of the other simulation its compared to, an independent samples statistical test is used. A Wilcoxon-rank-sum nonparametric test, equivalent to Mann Whitney U-test, was utilized. The test considers the summed rank scores to derive a statistical score and a p-value which is considered with a 95% confidence level (\(\alpha = 0.05\)).
Overview of effect of culture on dependent variables when consistency and status are high (upper graphs) and when consistency and status are low (lower graphs). In these graphs and the one in Fig. 5, the horizontal axis represents time (using an abstract time unit) and the vertical axis activation level (in the 0–1 interval)
The results were processed using a Python-IDE and an open-source scientific library (SciPy) to carry out the rank-sum test. The graphical output was first stored as excel files that show the state values at each time point (\(\Delta t = 0.25\)). The files were then converted to pandas data frame split into individual data frames for each dependent variable, which were then refined into a smaller time frame which excludes the first and last 50 time points in order to ensure significant data points. The scenarios were then compared with each other according to different cultures or the same culture and different leader behavior. A total of 54 comparisons were calculated measuring the statistical difference in individual learning, team learning, and mistake management. An overview of the statistical test scores can be summarized in the table of Sect. 4 in the Appendix. All statistical comparisons were significant as the p-value was much lower than the significance level (p-value \(\le 0.03\), where \(\alpha = 0.05\)) except for the gray-colored cells which proved to be insignificant differences. Thus, we can reject the null hypotheses as the presence of these non-significant results are still inline and support the research hypotheseses.
5 Discussion
According to the simulation results and statistical analysis, it is clear that culture in accordance with certain transformational leadership qualities plays a role in mistake acceptance and organizational learning. The simulations provide a clear visual indicator of the effect on the dependent variables: individual learning, team learning, and mistake acceptance. But they also demonstrate significant statistical results from each other. The statistical analysis in Sect. 4 aimed to provide statistical significance in simulation comparisons to support the research hypotheses:
-
1.
Higher flexibility and discretion in organizational culture results in better mistake management and thus better organizational learning.
-
2.
Effective organizational learning requires a transformational leader to have both high social and formal status and consistency.
-
3.
Company culture and leader’s behavior must align for the best learning effects.
Twelve of the non-statistically significant results are in accordance with the second hypothesis. These non-significant values show that alternating low consistency or status when the other is high is not significantly different from each other; as one of them being low demonstrates the same effect as the other being low. Lack of high consistency or status hinder the imitation phase making the retention much lower in such scenarios. In that regard there is limited learning from the leader and only team learning and learning from world observations. This supports that for optimal results both consistency and status require to be high. Further, this aligns not only with the second hypothesis but also the third hypothesis as having a culture with high flexibility and discretion and high transformational leadership qualities, such as consistency and status, achieves the best results in terms of organizational learning.
Finally, all statistically significant results (non-gray cells) support the first hypothesis as higher flexibility always resulted in better individual learning and mistake management. Notably, team learning did not result in statistically significant differences. There are two instances, besides culture transitioning scenarios, in which team learning had insignificant difference (p-value \(\ge 0.05\)). This can be due to the fact that team learning values are completely dependent on the incoming connections from individual learning values. Thus, when individual learning is significantly high, team learning values will consistently result in the same pattern displaying higher values than individual learning. This pattern however was not evident in all culture simulations. Hierarchy with low status or consistency, for instance, resulted in significantly low level of individual learning which was not enough to surpass the threshold to increase team learning level more.
References
Boone, T., Reilly, A.J., Sashkin, M.: Social Learning Theory. Prentice-Hall, Albert Bandura Englewood Cliffs, N.J. (1977)
Cameron, K., Quinn, R.: Diagnosing and Changing Organizational Culture: Based on the Competing Values Framework, 3rd edn. Jossey-Bass (2011)
Canbaloğlu, G., Treur, J., Roelofsma, P.H.M.P.: Computational modeling of organisational learning by self-modeling networks. Cogn. Syst. Res. 73, 51–64 (2022a)
Canbaloğlu, G., Treur, J., Wiewiora, A.: Computational modeling of the role of leadership style for its context-sensitive control over multilevel organisational learning. In: Proceedings of the 7th International Congress on Information and Communication Technology, ICICT'22 (Lecture Notes in Networks and Systems). Springer Nature Switzerland AG (2022b) https://www.researchgate.net/publication/355186556
Canbaloğlu, G., Treur, J., Wiewiora, A. (eds).: Computational Modeling of Multilevel Organisational Learning and its Control Using Self-Modeling Network Models. Springer Nature Switzerland AG, forthcoming (2023). https://www.researchgate.net/publication/355582177
Cannon, M.D., Edmondson, A.C.: Failing to learn and learning to fail (Intelligently). Long Range Plan. 38(3), 299–319 (2005). https://doi.org/10.1016/j.lrp.2005.04.005
Chénard Poirier, L.-A., Morin, A.J.S., Boudrias, J.-S.: On the merits of coherent leadership empowerment behaviors: a mixture regression approach. J. Vocat. Behav. 103, 66–75 (2017)
Crossan, M.M., Lane, H.W., White, R.E.: An organizational learning framework: from intuition to institution. Acad. Manag. Rev. 24, 522–537 (1999)
Hofstede, G., Hofstede, G.J., Minkov, M.: Cultures and Organizations. McGraw Hill (2010)
Jackson, P.L., Brunet, E., Meltzoff, A.N., Decety, J.: Empathy examined through the neural mechanisms involved in imagining how I feel versus how you feel pain. Neuropsychologia 44(5), 759–761 (2006). https://doi.org/10.1016/j.neuropsychologia.2005.07.015
Keysers, C., Gazzola, V.: Hebbian learning and predictive mirror neurons for actions, sensations and emotions. Philos. Trans. R. Soc. B: Biol. Sci. 369(1644), 20130175 (2014)
Kim, D.H.: A Framework and Methodology for Linking individual and organizational learning: applications in TQM and product development Massachusetts Institute of Technology Doctoral Dissertation (1993)
Kucharska, W.: Leadership, culture, intellectual capital, and knowledge processes for organizational innovativeness across industries: the case of Poland. J. Intellect. Cap. 22(7), 121–141 (2021)
Kucharska, W.: Do mistakes acceptance foster innovation? Polish and US cross-country study of tacit knowledge sharing in IT. J. Knowl. Manag. 25(11), 105–128 (2021)
Lawler, E.E.: The ultimate advantage: creating the high-involvement organization. Choice Rev. Online 30(01), 30–038730–0387 (1992). https://doi.org/10.5860/choice.30-0387
Lawler, E., Boudreau, J., Mohrman, S.: Achieving Strategic Excellence: An Assessment of Human Resource Organizations. Standford University Press, Stanford, CA (2006)
Schein, E.H.: Organizational culture. Am. Psychol. 45(2), 109–119 (1990)
Sims, H.P., Manz, C.C.: Social learning theory: The role of modeling in the exercise of leadership. J. Organ. Behav. Manag. 3(1981), 55–63 (1981)
Treur, J.: Modeling higher-order adaptivity of a network by multilevel network reification. Netw. Sci. 8, 110–144 (2020a)
Treur, J.: Network-Oriented Modeling for Adaptive Networks: Designing Higher-Order Adaptive Biological, Mental and Social Network Models. Springer Nature (2020b)
Treur, J.: Modeling Multi-Order Adaptive Processes by Self-Modeling Networks (Keynote Speech). In: Tallón-Ballesteros, A.J., Chen, C.-H. (eds.) Proceeding of the 2nd International Conference on Machine Learning and Intelligent Systems, MLIS'20. Frontiers in Artificial Intelligence and Applications, vol. 332, pp. 206–217. IOS Press (2020c)
Treur, J., Van Ments, L. (eds.): Mental Models and their Dynamics, Adaptation, and Control: a Self-Modeling Network Modeling Approach. Springer Nature, Cham (2022)
Weinzimmer, L.G., Esken, C.A.: Learning from mistakes: how mistake tolerance positively affects organizational learning and performance. J. Appl. Behav. Sci. 53(3), 322–348 (2017). https://doi.org/10.1177/0021886316688658
Wiewiora, A., Smidt, M., Chang, A.: The how of multilevel learning dynamics: a systematic literature review exploring how mechanisms bridge learning between individuals, teams/projects and the organization. Eur. Manag. Rev. 16(1), 93–115 (2019)
Author information
Authors and Affiliations
Corresponding author
Editor information
Editors and Affiliations
Rights and permissions
Copyright information
© 2023 The Author(s), under exclusive license to Springer Nature Switzerland AG
About this paper
Cite this paper
Samhan, N., Treur, J., Kucharska, W., Wiewiora, A. (2023). An Adaptive Network Model Simulating the Effects of Different Culture Types and Leader Qualities on Mistake Handling and Organisational Learning. In: Cherifi, H., Mantegna, R.N., Rocha, L.M., Cherifi, C., Miccichè, S. (eds) Complex Networks and Their Applications XI. COMPLEX NETWORKS 2016 2022. Studies in Computational Intelligence, vol 1077. Springer, Cham. https://doi.org/10.1007/978-3-031-21127-0_19
Download citation
DOI: https://doi.org/10.1007/978-3-031-21127-0_19
Published:
Publisher Name: Springer, Cham
Print ISBN: 978-3-031-21126-3
Online ISBN: 978-3-031-21127-0
eBook Packages: EngineeringEngineering (R0)