Abstract
Risk management and assessment is a multi-criteria decision-making problem involving various factors, according to literature research and expert opinions. In order to establish effective models and resolve the relationship between these criteria’s; Researchers and Academics have presented a wide range of methods or models in the literature. In order to overcome the most strategic issue that affects the project performance scales, such as Risk Management, the most appropriate method and criterion selection is required. In this study; A case study conducted using the multi-criteria Adaptive Neuro Fuzzy Inference System (ANFIS), which provides the rating of 105 recorded risk types covering the design and construction processes. Combining the structures and advantages of adaptive networks with fuzzy inference methodology has shown that a more comprehensive and effective risk management and assessment made. It has been shown with Root mean square error (RMSE), mean absulate percantage error (MAPE) and R2 performance indicators that it gives better results in artificial intelligence supported risk management or assessment created with Adaptive Neuro Fuzzy Inference System. The main contribution of this study; is the approach of artificial intelligence to evaluate and rate the risk correctly with hybrid learning method and then to obtain risk maps by integrating them into BIM as visual and linguistic term or pop-up expressions.
Access this chapter
Tax calculation will be finalised at checkout
Purchases are for personal use only
Similar content being viewed by others
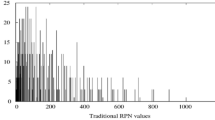
References
Gadd S, Keeley D, Balmforth H (2004) Pitfalls in risk assessment: examples from the UK. Saf Sci 42:841–857
Carr V, Tah JHM (2000) A proposal for construction project risk assessment using fuzzy logic. Constr Manag Econ 18:491–500
Dikmen I, Birgonul MT, Han S (2007) Using fuzzy risk assessment to rate cost overrun risk in international construction projects. Int J Project Manage 25(5):494–505
Lee HM, Lin L (2010) A new fuzzy risk assessment approach. Knowl-Based Intell Inf Eng Syst 98–105
McKim RA (1993) Neural networks and identification and estimation of risk. Trans AACE Int p 5.1
Wang YM, Elhag TM (2007) A fuzzy group decision making approach for bridge risk assessment. Comput Ind Eng 53(1):137–148
Wang YM, Elhag T (2008) An adaptive neuro-fuzzy inference system for bridge risk assessment. Expert Syst Appl 34(4):3099–3106
Wenxi Z, Danyang C (2009) Expressway management risk evaluation based on fuzzy neural networks. In: Proceedings of the 2009 second ınternational conference on ıntelligent computation technology and automation 02:700–703
Kunz J, Fischer M (2008) CIFE research questions and methods. Stanford University, Centre for Integrated Facility Engineering
Bais P (2020) medium.com. Retrieved from https://medium.com/@sakshisingh_43965/biological-artificial-neural-network-471722148217
Ross T, Donald S (1995) A fuzzy multi-objective approach to risk management. In: Mohsen JP (ed) Proceedings 2nd congress on computing in civil engineering. Held in conjunction with A/E/C Sys. ’95, vol 2, ASCE, New York, pp 1400–1403
Xınqıng L, Tsoukalas LH, Uhrıg RE (1996) A neurofuzzy approach for the anticipatory control of complex systems. In: Proceedings of IEEE 5th ınternational fuzzy systems. IEEE, pp 587–593
Ghorbanzadeh O, Rostamzadeh H, Blaschke T, Gholaminia K, Aryal J (2018) A new GIS-based data mining technique using an adaptive neuro-fuzzy inference system (ANFIS) and k-fold cross-validation approach for land subsidence susceptibility mappi. Natural Hazards, 497–517
Eastman CM et al (2011) BIM handbook: a guide to building information modeling for owners, managers, designers, engineers and contractors. Wiley
Chen TC (2000) Extensions of the TOPSIS for group decision—making on fuzzy environment. Fuzzy Sets Syst 114:1–9
Carr V, Tah JHM (2001) A fuzzy approach to construction project risk assessment and analysis: construction project risk management system. Adv Eng Softw 32(10–11):847–857
Zeng J, An M, Smith NJ (2007) Application of a fuzzy based decision making methodology to construction project risk assessment. Int J Project Manage 25(6):589–600
Howard R, Bjo ̈rk B-C (2007) Use of Standards for CAD layers in building. Autom Const 16(3):290–297
Author information
Authors and Affiliations
Corresponding author
Rights and permissions
Copyright information
© 2023 The Author(s), under exclusive license to Springer Nature Switzerland AG
About this paper
Cite this paper
Altunhan, İ., Sakin, M., Kaya, Ü., Fatih AK, M. (2023). Strategic Framework for ANFIS and BIM Use on Risk Management at Natural Gas Pipeline Project. In: Smart Applications with Advanced Machine Learning and Human-Centred Problem Design. ICAIAME 2021. Engineering Cyber-Physical Systems and Critical Infrastructures, vol 1. Springer, Cham. https://doi.org/10.1007/978-3-031-09753-9_8
Download citation
DOI: https://doi.org/10.1007/978-3-031-09753-9_8
Published:
Publisher Name: Springer, Cham
Print ISBN: 978-3-031-09752-2
Online ISBN: 978-3-031-09753-9
eBook Packages: Intelligent Technologies and RoboticsIntelligent Technologies and Robotics (R0)