Abstract
Parkinson’s Disease (PD) is a neurodegenerative illness associated with dopaminergic loss in the basal ganglia circuit which can lead to heterogeneous motor symptoms such as tremor, rigidity and bradykinesia. The electrophysiological phenomena underlying these symptoms is not completely understood, which imposes a major challenge for designing customized and more efficient Deep Brain Stimulation (DBS) protocols to match patients’ specificities and needs. Recently, it has been shown that elevated and prolonged beta (13–35 Hz) oscillations (i.e. beta bursts) from the subthalamic nucleus (STN) are associated with motor impairment in PD. Furthermore, motor improvement induced by pharmacological treatment relates to attenuation of intermittent beta activity. This work aims to analyze beta burst dynamics of two phenotypes of PD patients—the tremor dominant (TD) and the postural instability and gait difficulty (PIGD)—to better understand how features of beta oscillations correlate with the motor symptoms in such different PD’s categories. Through a wavelet analysis of 35 LFPs recorded in the sensorimotor portion of the STN from 15 TD and 20 PIGD patients, we show that PIGD patients exhibit longer beta bursts, while TD patients exhibit higher beta burst probability and an inverse significant correlation of burst duration with the rigidity score. These findings may provide critical markers for characterizing the electrophysiological mechanism underlying PD phenotypes and their symptoms, as also contribute to more efficient and customized DBS strategies.
Access provided by Autonomous University of Puebla. Download conference paper PDF
Similar content being viewed by others
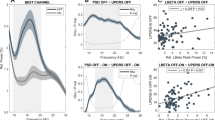
Keywords
1 Introduction
Parkinson’s disease (PD) is a neurodegenerative ilness associated with dopaminergic loss in the basal ganglia circuit, resulting in bradykinesia (i.e. slowness of movement), rigidity and tremor as main core motor symptoms [1,2,3]. Bradykinesia/rigidity clinical scores are related to abnormal beta band levels (13–35 Hz) in local field potential (LFP) in subthalamic nucleus (STN) [4, 5], a surgical target for continuous deep brain stimulation (cDBS) when pharmacological treatment is no longer efficient. The sucess of cDBS can be attributed, at least in parts, to the attenuation of the STN’s LFP beta power [6,7,8] under electrical stimulation at a fixed frequency (e.g. 140 Hz).
Albeit efficacious, cDBS can introduce side effects such as dysarthria, sleep disorders, sensory complains and pain, besides higher battery drainage [9, 10]. These clinical and technological drawbacks can be minimized with a better understanding of the PD electrophysiological mechanisms, as evidenced by the recently proposed adaptive DBS (aDBS) strategies [9, 10].
It was shown that aDBS can be more efficient in terms of battery life-time when a dual-threshold dependent on motor symptoms (tremor and bradykinesia) was adopted [12]. The beta-band power has also been used to determine control setpoints for driving the voltage amplitude applied to the STN in conditions such as free-movement [9] or when accompanied by treatment with levodopa [11]. Despite these advances [13,14,15,16], it is unclear whether electrophysiological features, especially beta band power, differ between the main PD’s phenotypes—tremor dominant (TD) and postural instability and gait difficulty (PIGD).
Moreover, beta activity occurs in an intermittent way, and thus is better characterized in terms of time–frequency events. This led to the concept of beta bursts, i.e. a supra-threshold “package” of beta band activity, the dynamics of which has been correlated with on and off dopaminergic conditions [18]. In fact, prolonged beta bursts has been proposed as a potential biomarker of motor impairment in PD [17,18,19] and a candidate for driving the voltage-levels in aDBS [20].
This work aims to analyze the beta bursts dynamics in TD and PIGD phenotypes of PD observed in low (13–22 Hz) and high- (22–35 Hz) beta frequency bands, according to functional spectral division previously reported in the literature [19, 21,22,23]. Our findings may help to partially elucidate the differences of voltage amplitude-responsiveness between the PD phenotypes during aDBS [12], therefore contributing to this therapeutic strategy, being customized based on patients’ main clinical symptoms.
2 Materials and Methods
-
A. Patients
All patients (N = 25) were clinically diagnosed with PD by a movement disorders specialist at Santa Marcelina Hospital (São Paulo, SP, Brazil). The study was carried out in agreement with ethical standards in which prior written consent from Santa Marcelina Hospital Ethics Committee (CAAE: 62,418,316.9.2004.0066) was obtained from each patient. Patients were assigned as TD or PIGD phenotypes according to the “Unified Parkinson’s Disease Rating Scale” from the “Movement Disorders Society” (MDS-UPDRS) as previously proposed in [24]. This classification considers the ratio of mean tremor scores to mean gait-related scores [3]. For UPDRS III scale, patients with scores larger than or equal to 1.5 were assigned as TD, while those with scores lower than 1.0 were assigned to PIGD. For MDS-UPDRS scale, patients with scores larger than or equal to 1.15 were assigned to TD group, while those with scores lower than 0.9 were assigned to PIGD group. Patients out of these ranges were considered indeterminate and were not considered in the analysis. The resulting cohort (N = 24) was composed of 11 TD, and 13 PIGD including 16 males and 8 females with an average age of 55 years and an average disease duration of 8 years. Thirteen patients received bilateral DBS electrodes (5 TD and 8 PIGD), while 9 patients received unilateral implants (5 TD and 4 PIGD), which amounted to a total of 35 LFP signals acquired (15 TD and 20 PIGD).
-
B. Surgery, LFP Recordings and Analysis.
The Surgical procedure was performed as previously described in [7]. Briefly, the stereotactic localization of the sensorimotor STN target was directly visualized (Fig. 1a) using susceptibility weighted imaging (SWI) and T2-weighted Magnetic Resonance Imaging (MRI) sequences which are digitally fused with the stereotactic Computed Tomography. Microelectrodes recordings were performed by a set of three parallel 1 MΩ impedance tungsten microelectrodes (microTargeting® electrodes, FHC, Greenville, MA, USA) in patients completely awake with their eyes opened. Action potentials were amplified (10,000-fold) and filtered (0.5–10 kHz, notch filtered at 60 Hz)—(Lead Point—Medtronic, Minneapolis, MN, USA). Recordings started at 5 mm above the STN and its corresponding dorsal region was identified by an increase in the amplitude of the background noise and irregular burst pattern. LFP monopolar recordings were performed at 1 mm ventral to the most dorsal border of the STN over the implantation trajectory of the electrodes. Therefore, LFPs were recorded in the sensorimotor STN sector, as close as possible to the chronic DBS stimulating contacts (Fig. 1b). Signals were sampled at 24 kHz, band-pass filtered (1 Hz to 200 Hz) and recorded during 60 s at the resting-state condition.
Beta bursts estimates. Anatomical STN localization for DBS surgery a and LFP recording site signal acquisition at 1 mm ventral to the most dorsal border of the STN b. CWT coefficients heat map for beta 1 estimates at each time–frequency point c. LFP filtered (black continuous line), threshold (blue dashed line), beta burst envelope (red continuous line) and shape (red shaded area) for beta 1 d
-
C. Signal Pre-processing and Beta Burst Estimates.
The LFP STN signals were exported and post-processed in Matlab 2018b. Signals were downsampled to 1 kHz, followed by notch filtering at 60 Hz, band-pass filtering (6th order bandpass Butterworth filter, 2–200 Hz) and z-scored normalized. The frequency bandwidth aimed to preserve all the main LFP sub-bands: theta (4–8 Hz), alpha (8–13 Hz), low beta (13–22 Hz), high beta (22–35 Hz), low gamma (35–100 Hz) and high gamma (100–200 Hz). The beta band was divided into low beta (beta 1) and high-frequency beta (beta 2) sub-bands as previously described in the literature [19, 21,22,23] for capturing functional beta-band division.
Intermittent beta-band analysis was performed after LFP filtering (6th order bandpass Butterworth filter) at low (13–22 Hz) and high-frequency (22–35 Hz). Evaluation of beta oscillations was obtained by means of continuous wavelet transform (CWT) using the Fieldtrip toolbox [17,18,19,20]. Briefly, filtered LFP was convolved with a Morlet wavelet function, which is composed of complex exponential function modulated by a Gaussian envelope. In order to estimate a reliable intermittent beta activity, the number of cycles and the length of the Morlet, were set following [18], respectively to 10 cycles and 5 standard deviations of the Gaussian kernel. The CWT coefficients were obtained for each time–frequency point after the convolution operation for each beta sub-band as shown in (Fig. 1c). Such coefficients were averaged within the respective beta sub-band at each time instant, and, finally, z-scored normalized to provide the respective beta envelope (continuous red line in Fig. 1d). Beta bursts intervals (shaded area in red depicted in Fig. 1d) were determined by thresholding at 75th percentile (blue horizontal dashed line) the envelope of beta envelope strength according to the proposal presented in [17, 18]. Bursts with duration shorter than 100 ms were not considered for further analysis, since they may be associated with background activity. In addition, a minimum interval of 60 ms between bursts was considered as a separation criterion.
The beta bursts dynamic was characterized in terms of the burst probability (BP, i.e. the number of bursts per unit of time—bursts/s), the averaged burst duration (AVD, s) and the averaged burst strength (AVS, a.d.) for each subject within the TD and PIGD cohorts. Moreover, a dichotomization in terms of short and long bursts was introduced according to previous work of [17,18,19]. The burst duration was also considered separately for short (lasting from 100 to 600 ms) and long (lasting more than 600 ms) bursts. Subsequently, it was possible to introduce and analyze the percentage of shorter durations (PSD) and the percentage of prolonged durations (PPD), respectively. This dichotomized classification is justified by previous works, in which prolonged and elevated bursts (i.e. durations > 600 ms) were associated with abnormal motor symptoms [17, 18].
-
D. Supervised Learning.
A supervised learning approach was employed aiming to characterize how informative the attributes are for predicting the PD phenotypes, supporting the electrophysiological characterization. PD phenotypes were predicted based on burst dynamics parameters defined by the BP, AVS, AVD, PSD and PPD. A leave-one-out cross-validation scheme was adopted for data partitioning and linear discrimination analysis (LDA) was employed for predicting PD phenotypes [25]. Classification performance was accessed in terms of the accuracy (acc), and the area under the receiving operating characteristics (ROC) curve (AUC).
-
E. Statistical Analysis and Clinical Correlation.
The data is presented as mean ± standard deviation (SD). Normality of parameter distributions was checked using at least 3 statistical approaches: D’Agostino-Pearson, Shapiro–Wilk and Kolmogorov–Smirnov tests. Nonpaired t-student (or Mann–Whitney for non-normal distribution) hypothesis tests were applied when suitable for comparing the BP, AVS and AVD. The correlation coefficient (ρ) for burst parameters and UPDRS clinical scores (tremor, rigidity and bradykinesia) were evaluated for both PD phenotypes. Pearson’s and Spearman’s coefficients were employed, respectively, for normal and non-normal distributions.
Interaction between burst duration category (i.e. short < 600 ms and prolonged beta burst > 600 ms) and PD phenotypes (TD and PIGD) was investigated by a two-way mixed measurement analysis of variance with 2 vs. 2 design (two burst lasting intervals vs. two PD phenotypes). PSD and PPD were taken as the dependent variables underlying burst duration categories. We hypothesized that the categorized burst duration distribution may be indicative of the PD phenotype and be correlated with the predominant PD motor symptoms, (e.g. tremor for TD and bradykinesia for PIGD). These comparisons for different categorized bursts for specific phenotypes were performed using post-hoc tests with Bonferroni’s corrections for multiple comparisons. In all statistical analyses, the significance level for statistical differences was defined lower than 0.05.
3 Results
Beta 2 sub-band did not present any significant difference in the beta burst dynamics between the PD phenotypes, while beta 1 revealed that PIGD patients exhibited bursts with longer durations and TD patients exhibited a higher probability of bursts. Phenotypes classification performance based on such parameters agrees with their statistical distribution distinction, as evidenced by AUC for AVD and BP. Statistical and classification analyses are summarized in Table 1.
The strength of beta burst was positively correlated with burst durations (data not shown) for both phenotypes in both beta sub-bands as previously obtained for burst evaluated in patients with and without dopaminergic medication [18]. Moreover, it was observed that beta 1 AVD were moderately negatively correlated with tremor symptom score (Fig. 1a) with a negligible correlation for both phenotypes (ρ = −0.073, p = 0.803, TD; and ρ = −0.073, p = 0.912, PIGD). Bradykinesia exhibited analogous results, (ρ = −0.295, p = 0.285, for TD and ρ = −0.001, p = 0.997 for PIGD), while TD patients exhibited a significantly inverse negatively correlated behavior with rigidity (ρ = −0.704, p < 0.001) as shown in (Fig. 2b).
The mixed two-way analysis of variance showed no significant interaction between the percentage of short and long bursts with the PD phenotypes (Fratio = 2.591, p = 0.117) for beta 1 as shown in Fig. 3a, whereas a significant difference was observed between percentage of short and long bursts (Fratio = 4434, p < 0.0001). Main effect for phenotype was also detected (Fratio = 4.684, p = 0.038), although post-hoc comparison between TD and PIGD revealed that PSD and PPD did not differ between TD and PIGD (p = 0.225 for both variables). The phenotype classification performance (Fig. 3b) indicated that the burst duration did not aid phenotype discrimination, suggesting that burst duration category definition (duration threshold) and their distributions in phenotype classes can play a key role.
4 Discussion
In this work, we aimed to employ wavelet analysis to characterize the intermittent beta activity of LFP recordings from STN in different PD phenotypes (TD and PIGD), under the hypothesis that beta activity may be associated with predominant motor symptoms (i.e. tremor vs. bradykinesia-rigidity). We show that the beta burst dynamics for TD and PIGD patients differs in terms of burst duration and probability, providing informative features for phenotypes prediction. PIGD patients exhibit longer bursts, while TD patients exhibit higher burst probability. The average burst duration was also negatively correlated with the rigidity score only for TD cohort. This suggests that prolonged beta burst affect the rigidity differently in the TD and PIGD phenotypes. Previously, Eisinger et al. (2020) [26] have investigated the burst dynamics in STN and globus pallidus recordings, showing no significant correlation between rigidity and beta burst parameters. The divergence with our findings may be related to the lack of a phenotypic division of PD patients and the definition of a narrow beta sub-band (14–18 Hz) in their work. Interestingly, our findings support the findings to the works of [19, 21], in which beta 1 seems to manifest the physiopathology of the basal ganglia circuit, whereby the beta 1 bandpower was significantly and positively correlated with hemibody bradykinesia/rigidity [21].
Since it has been previously shown that prolonged bursts (> 600 ms) are associated with pathological behavior in dopaminergic off medication [18], we have analyzed the burst dynamics under the dichotomized categories of short and longer bursts following the same criterion. In this case, we verified that systematic differences of PSD and PPD bursts were the same for the different PD phenotypes, i.e. no interaction was found. In addition post-hoc tests did not reveal any difference between short and long burst duration percentages of PD phenotypes, despite main effects have been found. Classification based on PPD bursts was performed also slightly better than a random classifier. As a general explanation, the dichotomization into short and long burst shows that PIGD tends to exhibit longer bursts than TD, but the difference in terms of the BP (i.e. number of bursts for PIGD is lower than TD) may also impact on the statistics of such division, justifying the observed results. It is also conceivable that the original burst duration threshold definition was introduced based on longer recordings and chosen in terms of specificities of the distributions obtained in that situation [18], which, probably, would require a more detailed analysis for a suitable extrapolation to other datasets.
From the methodological standpoint, the multiparametric adjustments of wavelet analysis to extract beta bursts envelope is also a matter of debate. Accurate measurement of beta burst features, although promising, still may be suboptimal and the differences between conditions/phenotypes may be masked by several parameters. The approach employed here was recently proposed [17,18,19] in order to facilitate further comparisons. In fact, thresholding the envelope at 75th percentile may bias the results [27] and more robust strategies have been discussed recently [17,18,19]. Finally, apart of the CWT, beta bursts can be also estimated via the Hilbert Transform (HT). Depending on the adopted approach (i.e. CWT or HT), a proportion of bursts of different duration in free-movement conditions is different for each phenotype [28]. The employment of HT to this dataset could arguably provide different results than the current findings, since HT-based method usually results in higher distribution of shorter bursts [27, 28] when compared to CWT. This alternative approach illustrates the different results observed in comparison to CWT.
5 Conclusions
In this work, we have shown through a statistical and supervised learning approach that beta burst dynamics differs between TD and PIGD phenotypes. More specifically, PIGD patients exhibit longer bursts, while TD patients exhibit a higher burst probability. Both variables provided phenotype classification performance considerably superior than a random classifier, suggesting that beta burst dynamics is a meaningful electrophysiological feature to distinguish the different PD phenotypes. Moreover, mean burst duration was negatively correlated with rigidity just for TD patients, illustrating how PD’s electrophysiological LFP correlates can be distinct for different phenotypes. As a major conclusion, we observed that TD and PIGD phenotypes of PD exhibit different electrophysiological fingerprints, as captured here through the burst dynamics. These findings deserve careful attention since burst dynamics can provide crucial control variables for distinct aDBS strategies according to PD phenotypes.
6 Conflict of Interest
The authors declare that they have no conflict of interest.
References
Brown P, Oliviero A, Mazzone P, Insola A, Tonali P, Di Lazzaro V (2001) Dopamine dependency of oscillations between subthalamic nucleus and pallidum in Parkinson’s disease. J Neurosci 21(3):1033–1038
Canessa A, Pozzi NG, Arnulfo G, Brumberg J, Reich MM, Pezzoli G et al (2016) Striatal dopaminergic innervation regulates subthalamic beta-oscillations and cortical-subcortical coupling during movements: Preliminary evidence in subjects with Parkinson’s disease. Front Hum Neurosci. https://doi.org/10.3389/fnhum.2016.00611
Stebbins GT, Goetz CG, Burn DJ, Jankovic J, Khoo TK, Tilley TC (2013) How to identify tremor dominant and postural instability/ gait difficulty groups with the movement disorder society unified Parkinson’s disease rating scale: Comparison with the unified Parkinson’s disease rating scale. Mov Disord 28(5):668–670
Kühn AA, Kupsch A, Schneider GH, Brown P (2006) Reduction in subthalamic 8–35 Hz oscillatory activity correlates with clinical improvement in Parkinson’s disease. Eur J Neurosci 23:1956–1960
Kühn AA, Tsui A, Aziz T, Ray N, Brücke C, Kupsch A et al (2009) Pathological synchronisation in the subthalamic nucleus of patients with Parkinson’s disease relates to both bradykinesia and rigidity. Exp Neurol 215:380–387
Salat D, Tolosa E (2013) Levodopa in the treatment of Parkinson’s disease: Current status and new developments. J Parkinson’s Dis 3:255–269
Godinho F, Thobois S, Magnin M, Guenot M, Polo G, Benatru I et al (2006) Subthalamic nucleus stimulation in Parkinson’s disease: anatomical and electrophysiological localization of active contacts. J Neurol 253:1347–1355
Wingeier B, Tcheng T, Koop MM, Hill BC, Heit G, Bronte-Stewart HM (2006). Intra-operative STN DBS attenuates the prominent beta rhythm in the STN in Parkinson’s disease. Exp Neurol.;
Rosa M, Arlotti M, Ardolino G, Cogiamanian F, Marceglia S, Di Fonzo A et al (2015) Adaptive deep brain stimulation in a freely moving parkinsonian patient. Mov Disord 30:1003–1005
Little S, Pogosyan A, Neal S, Zavala B, Zrinzo L, Hariz M et al (2013) Adaptive deep brain stimulation in advanced Parkinson disease. Ann Neurol. 74:449–457
Rosa M, Arlotti M, Marceglia S, Cogiamanian F, Ardolino G, Di FA et al (2017) Adaptive deep brain stimulation controls levodopa-induced side effects in Parkinsonian patients. Mov Disord 32:628–629
Velisar A, Syrkin-Nikolau J, Blumenfeld Z, Trager MH, Afzal MF, Prabhakar V et al (2019) Dual threshold neural closed loop deep brain stimulation in Parkinson disease patients. Brain Stimul. 12:868–876
Quinn EJ, Blumenfeld Z, Velisar A, Koop MM, Shreve LA, Trager MH et al (2015) Beta oscillations in freely moving Parkinson’s subjects are attenuated during deep brain stimulation. Mov Disord. 30:1750–1758
Telkes I, Viswanathan A, Jimenez-Shahed J, Abosch A, Ozturk M, Gupte A et al (2018) Local field potentials of subthalamic nucleus contain electrophysiological footprints of motor subtypes of Parkinson’s disease. Proc Natl Acad Sci U S a. 36:E8567–E8576
Baldi JB, Bianqueti BL, Fim Neto A, Almeida TP, Yoneyama T, Rocha MS, Soriano DC, Godinho F (2019) Rest and active arm movement classification based on subthalamic nucleus LFPs for Parkinson's disease subtypes. SIIM 2019
Bianqueti BL, Baldi, JB, Fim Neto A, Almeida TP, Yoneyama T, Rocha MS, Soriano DC, Godinho F (2019) Distinct Parkinson's disease symptoms relates to different LFP frequency bands. SIIM 2019
Tinkhauser G, Pogosyan A, Little S, Beudel M, Herz DM, Tan H et al (2017) The modulatory effect of adaptive deep brain stimulation on beta bursts in Parkinson’s disease. Brain 140:1053–1067
Tinkhauser G, Pogosyan A, Tan H, Herz DM, Kühn AA, Brown P (2017) Beta burst dynamics in Parkinson’s disease off and on dopaminergic medication. Brain 140:2968–2981
Lofredi R, Tan H, Neumann WJ, Yeh CH, Schneider GH, Kühn AA et al (2019) Beta bursts during continuous movements accompany the velocity decrement in Parkinson’s disease patients. Neurobiol Dis. 127:462–471
Moraud EM, Tinkhauser G, Agrawal M, Brown P, Bogacz R (2018) Predicting beta bursts from local field potentials to improve closed-loop DBS paradigms in Parkinson’s patients. Proc Ann Int Conf IEEE Eng Med Biol Soc EMBS 978:5386–3646
van Wijk BCM, Beudel M, Jha A, Oswal A, Foltynie T, Hariz MI et al (2016) Subthalamic nucleus phase-amplitude coupling correlates with motor impairment in Parkinson’s disease. Clin Neurophysiol. 127:2010–2019
Litvak V, Jha A, Eusebio A, Oostenveld R, Foltynie T, Limousin P et al (2011) Resting oscillatory cortico-subthalamic connectivity in patients with Parkinson’s disease. Brain 134:359–374
Oswal A, Brown P, Litvak V (2013) Synchronized neural oscillations and the pathophysiology of Parkinson’s disease. Curr Opin Neurol 26:662–670
Jankovic J, Carter J, Gauthier S, Goetz C, Golbe L, Huber S et al (1990) Variable expression of Parkinson’s disease: a base-line analysis of the DATATOP cohort Parkinson Study Group. Neurology 40:1529–1534
Theodoridis S, Pikrakis A, Koutroumbas K, Cavouras D (2010) Introduction to pattern recognition: a matlab approach. A Matlab Approach, Introduction to Pattern Recognition
Eisinger RS, Cagle JN, Opri E, Alcantara J, Cernera S, Foote KD et al (2020) Parkinsonian beta dynamics during rest and movement in the dorsal pallidum and subthalamic nucleus. J Neurosci. 40:2859–2867
Schmidt SL, Peters JJ, Turner DA, Grill WM (2020) Continuous deep brain stimulation of the subthalamic nucleus may not modulate beta bursts in patients with Parkinson’s disease. Brain Stimul 13:433–443
Fim Neto A, Baldi JB, Bianqueti BL, Almeida TP, Yoneyama T, Rocha MS, Godinho F, Soriano DC (2020) Estimation of beta burst durations from subthalamic nucleus local field potentials in Parkinson’s disease through hilbert and continuous wavelets transforms. 8th Latin American conference on biomedical engineering and the 42nd national conference on biomedical engineering, CLAIB-CNIB 2019. IFMBE Proceedings
Acknowledgements
Acknowledgments to Federal University of ABC and Santa Marcelina Hospital for providing the resources and contributing for developing this project and also to FAPESP (2018/14283-8, 2018/14285-0, 2019/09512-0, 2017/00319-8), CNPq (117659/2018-5), Capes (2019/1814368), BRAINN (2013/07559-3) and FINEP (n. 01.16.0067.00) for financial support.
Author information
Authors and Affiliations
Editor information
Editors and Affiliations
Rights and permissions
Copyright information
© 2022 Springer Nature Switzerland AG
About this paper
Cite this paper
Fim Neto, A. et al. (2022). Subthalamic Beta Burst Dynamics Differs for Parkinson’s Disease Phenotypes. In: Bastos-Filho, T.F., de Oliveira Caldeira, E.M., Frizera-Neto, A. (eds) XXVII Brazilian Congress on Biomedical Engineering. CBEB 2020. IFMBE Proceedings, vol 83. Springer, Cham. https://doi.org/10.1007/978-3-030-70601-2_325
Download citation
DOI: https://doi.org/10.1007/978-3-030-70601-2_325
Published:
Publisher Name: Springer, Cham
Print ISBN: 978-3-030-70600-5
Online ISBN: 978-3-030-70601-2
eBook Packages: EngineeringEngineering (R0)