Abstract
Given the importance of Northern Eurasia for global ecosystem and climate processes, improved characterization of land cover and land-cover change in the region is a scientific priority. Most studies on Central Asia focus on the changes in land cover and land use rather than on landscape dynamics. This chapter gives an overview of approaches and existing frameworks to describing landscape dynamics. The landscape dynamics are presented by state variables. Potential state variables are for example, NDVI, GPP, NPP, fPAR, or LAI. The progression of a state variable might fluctuate over time in response to disturbance and succession processes and fluctuate within a “natural range of change or variability”. This range of variability is also called as the “normal multiple states operating range” of a state variable. It is important to note that this range of variability is relative to a specific spatial and temporal scale. We present two case studies, one from Kazakhstan and one from Mongolia, which show different results in the course of important state variables (e.g. NDVI). The analysis of changes in vegetation indices in Kazakhstan (differential – NDVI, VCI and integral – IVI, IVCI), computed over the territory of Kazakhstan for the period 2000–2016, shows that the values of integrated indices of vegetation have an expressed tendency to decrease. While long-term NDVI trends in Mongolia, typically analyzed using AVHRR or MODIS data starting from the early 1980s, have often been described as negative, we could show that this is not true anymore in recent years. Both case studies show that Land-use change modelling is a highly dynamic field of research with many new developments. The main current developments presented in these special case studies concern progress in modelling of scale dependency of drivers of land-use / cover change.
Access provided by Autonomous University of Puebla. Download chapter PDF
Similar content being viewed by others
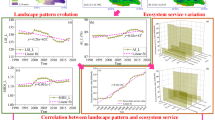
Keywords
- Landscape dynamics
- Ecosystem state variables
- State-and-transition models
- NDVI
- IVI
- SAVI
- Kazakhstan
- Mongolia
9.1 Introduction
Central Asia’s countries, including Mongolia, have been undergoing an extensive transformation of land cover (biophysical attributes of the earth’s surface) and land use (human purpose-oriented land) change over the 19th, 20th and 21st centuries. Satellite remote sensing in combination with intensive field research has been an important approach in monitoring these land-cover and land-use changes in Central Asia.
Most landscapes of Central Asia belong to the category of drylands, which include arid, semi-arid and dry-sub-humid ecosystems. Many people live in these landscapes at subsistence level (Millennium Ecosystem Assessment 2005a) and these systems play also a role in storing terrestrial carbon (mostly soil-carbon; Millennium Ecosystem assessment 2005b). Moreover, these landscapes support a huge amount of the world’s livestock (Allen-Diaz et al. 1996) and own areas with high biodiversity (Myers et al. 2000).
The most important transformations are triggered by the following changes such as land abandonment (e.g. in the twentieth century) and re-cultivation, new agricultural expansion in combination with increase and decrease of land-use intensity. But also processes of land degradation and vegetation recovery are important indicators of changing ecosystem conditions and land dynamics. A trend that exists in all Central Asian countries is the depletion of rural areas and the growth of cities known as the process of urban sprawl and contraction (see also Chap. 1, Henebry et al. 2020a). Given the importance of Northern Eurasia for global ecosystem and climate processes, improved characterization of land cover and land-cover change in the region is a scientific priority. This region is the largest landmass out of the tropics, the largest terrestrial reservoir of organic carbon, an area of active land-use changes and socio-economic transformations, and a major source of uncertainty in many global-scale estimates. The important role humans play in land-cover dynamics is widely recognized, however quantitative understanding of this role remains a challenge. Availability and quality of social science data is a cause for concern and methods for combining the remotely sensed and social science data need to be developed further so that robust projection of future changes in land cover and land use can be made (see Chap. 10 of this book, Chen et al. 2020).
In the last decades, huge data sets about the stage of the Eurasian drylands were created that help in studying landscape dynamics. In the last years, a lot of research focused on land-cover and land-use change in Central Asia and documented the underlying trends (e.g., Chen et al. 2020). One major and challenging question remained in this connection, namely the question of the stability, persistence, resistance, resilience and recovery of ecosystems in the different landscapes of Central Asia. It is evident that there is a need for dynamic system-related research to deliver a framework for future oriented decision tools.
Hereinafter the current chapter will provide an overview of important concepts underpinning the study of landscape dynamics and alternative concepts of system thinking and landscape equilibrium. Existing frameworks for the assessment of social-ecological-systems (SES) , system thinking and new combined ecologic key concepts will be used. Finally, the theory of spatial and temporal scaling of disturbance regimes and the influence on equilibrium/non-equilibrium dynamics are highlighted. At the end of the chapter, two case studies (i.e., Kazakhstan and Mongolia) will underpin the dynamics of important state variables such as NDVI.
9.2 Landscape Dynamics and Ecosystem Stewardship
At the beginning, an elementary conceptual framework for investigating landscape dynamics is used. In this simple framework, system dynamics is characterized as a curve over time in a state variable. State variables are any variables that describe the state or condition of the system at a single point in time. They are in general present measures of system structures or functions. For example, NDVI , GPP, NPP , fPAR, or LAI are all examples of potential state variables. The progression of a state variable might fluctuate over time in response to disturbance and succession processes and fluctuate within a “natural range of change or variability”. This range of variability is also called as the “normal multiple states operating range” of a state variable (see also Chap. 3 of this book, Henebry et al. 2020b, where important ECV’s of Eurasia and their natural range of variability are analyzed). It is important to note that this range of variability is relative to a specific spatial and temporal scale.
Under human influence, the course of the state variable could change its behavior and develop a new range of variability. In Fig. 9.1a, there is a simple model shown for assessing the outcome of human activities on system dynamics. In Fig. 9.1b, two alternative human-altered curves of the state variable are drawn. The question is whether the system condition drawn by each curve is “normal” in reference to the natural range of change. In both cases, the dynamics of the state variable are modified (dampened.) In the first case (red curve), the state variable remains within its natural range of change, while in the second case (blue curve), the state variable moves to an equilibrium state outside of its natural range of change. In both cases, it is open whether the curves show a “resilient” system. This is not easy to answer in either case.
Thinking in system dynamics automatically brings up the question of scale because the range of change in any state variable can only be seen in reference to a specific spatial and temporal scale. For example, as the spatial extent of the system grows up (i.e., coarser scale), the expected range of variability is likely to go down as the system increasingly is able to incorporate the disturbances. This is called as the balance of disturbance against succession in relation to Watt’s unit pattern (Watt 1947). A central question is how this scaling relationship changes under a human-modified disturbance regime. For example, if the human-altered curve looks like the one in Fig. 9.2, it is shown that the range of change is increasingly dampened as the spatial scale increases.
A major question understanding landscape dynamics is whether recent trends in state variables (e.g. NDVI , LAI or populations) are biologically significant and signal the need for corrective action. For example, if the curve is decreasing (e.g., population decrease), we might think that immediate management action is needed. An understanding of system dynamics may reveal that the decrease is perfectly natural and within the range of natural change.
A quantitative understanding of system dynamics can be important for giving a reference framework for interpreting measures of landscape structure, because in most cases it is difficult or even impossible to determine the ecological significance of a derived value of a landscape metric without understanding its natural range of change.
In landscape dynamics, many expressions have been defined to explain the state of a landscape over time. Moreover, these expressions describe, how the landscape state changes, and how the trajectory of change for the landscape system will response to a disturbance. Discovering system state changes requires identifying state variables that are representative of the system state. Keeping in mind that it is extremely difficult to identify state variables that are sensitive enough to fine-scale changes in ecosystem states and that can be used to detect system changes due to a human activity.
In the following, the most important system descriptors are shortly explained that offer a conceptual frame for the study of systems dynamics:
The term “Stability” means the tendency of a system state variable or an entire landscape system to shift away from a stable state but only within defined bounds. In the case of a landscape systems, which are ecological systems, stability refers not to stagnation of all state variables but to variations within defined borders. A system gets unstable when the system or state variables cross some thresholds from which a recovery to the former state is either impossible or develops only over relatively long periods. Therefore, we call a system “persistent” when the system remains in a defined state. Persistence is the length of time a system stays in a defined state but within some variations. The term resistance describes the capacity of a system to absorb or alter disturbances and protect the system from larger perturbations. Resistance mechanisms can be seen as a filter that diminish the impact of large disturbances. Another important term is “Resilience”. Resilience is the capability to return to the initial state after a disturbance. The resilience is related to the borders in state space in which a system will vary. If the system crosses these borders, the system will change to another state with new system state borders. Most important is that a resilient system is able to support the same key processes as before the disturbance and after recovering. The system integrity is preserved. The “Recovery” of the system describes the speed with which a landscape system returns to the initial state after a disturbance.
Stability of landscape systems can be obtained in different ways: Systems, which changed relatively easily, have therefore a low resistance but will return to the initial system state quickly. These systems have a rapid recovery time and high resilience and are mostly characterized by low biomass.
Other systems, which own a high resistance to disturbance and have a high persistence (stay in a stable state for long time), are mostly characterized by high biomass.
Changes in landscapes are natural processes and take place constantly. A simple concept of order is that of balance or equilibrium in the sense of constancy. This means that are no great changes over time. However, this is a very theoretical concept and disturbances and change are integral parts of landscape dynamics and make this concept of equilibrium unrealistic. Nevertheless, Turner et al. (1993) reviewed several concepts of landscape equilibrium.
First, the “shifting mosaic steady-state concept” (Bormann and Likens 1979) is introduced, which means that vegetation existing at individual patches in the landscape changes, but looking over a long time period or large area, the vegetation of the landscape is relatively constant or in an equilibrium. This concept describes a balance between disturbance (creation of new patches, e.g. by fire) and succession (e.g. regrowth of old patches). New patches inside the landscape system are balanced after disturbance by the maturation of old patches (by succession). The entire landscape system remains in a “steady-state” or “equilibrium” situation. The “shifting mosaic steady-state concept” is usable when the disturbances are small and frequent in a large area of a mostly homogeneous habitat. That’s why larger areas show a more stable mosaic in the landscape than small areas. In the last years, stable mosaics could be identified over large areas. Therefore, the concept supposes that influences of topography, soils, soil moisture or other landscape factors have an effect on disturbance frequency or recovery time inside the landscape. A major challenge of this concept is that it is scale-dependent. The right choice of temporal and spatial scales to examine these landscape mosaics is challenging.
State-and-Transition Models (STMs) have emerged as the leading conceptual framework to describe vegetation dynamics over a range of management and restoration scenarios (Asefa et al. 2003; Chartier and Rostagno 2006; Quetier et al. 2007; Tietjen and Jeltsch 2007; Sankaran and Anderson 2009; Standish et al. 2009). These STM models are mostly qualitative models that show potential alternative stable vegetation states based on a particular combination of site conditions (e.g., soil, soil moisture) and climate. Further, they give an idea about possible transitions between states. These transitions indicate thresholds between alternative stable states that are normally viewed as irreversible without intensive management inputs.
These models also are useful for communicating complex ecosystem dynamics to diverse stakeholders in a simple form (mostly flowcharts). The major weaknesses in these models and other related conceptual models is that they have limited predictive capability. Further, they do not address management uncertainty and have only restricted ability to link management issues to multiple ecological processes in a quantitative way that foster ecosystem and landscape change. These constraints limit application of these models to scenario analysis and evaluation of landscape dynamics. The two case studies about Kazakhstan and Mongolia focus on the vegetation dynamics as an important state variable providing an overview about possible transitions between long-term states of vegetation cover.
9.3 Case Study from Kazakhstan
Most of the territory of the Republic of Kazakhstan, located in the arid and semi-arid zones, is vulnerable to the observed climate changes. The period 2004–2014 for the territory of Kazakhstan is characterized by an increase of continentality of climate and, as a consequence, an increase of situations with adverse and extreme weather events, among which the most dangerous is the drought.
The III-VI National Communication of the Republic of Kazakhstan to the UN Framework Convention on Climate Change was prepared in the framework of a joint project of the United Nations Development Program in Kazakhstan and the Ministry of Environment and Water Resources of the Republic of Kazakhstan. This National Communication synchronizes the timing of the submission of National Communications with other countries and includes reports for the period 2006–2012. The document is intended for government agencies, scientific and public organizations.
As noted in the National Communication of the Republic of Kazakhstan (2013), the increases in mean annual and seasonal surface air temperatures, especially in the summer months, are observed ubiquitously. Particularly rapid rates of warming began in the 1980s, which led to a high frequency of warm years. On average in Kazakhstan in all years in the period 1997–2010 (14 consecutive years), the average annual air temperatures were above the climatic norm calculated for the period 1971–2000 by 0.3–1.4 °С. The average annual air temperature increased at a rate of 0.28 °C every 10 years. More significantly, average annual temperatures increased in the north, west and south of Kazakhstan – by 0.30–0.37 °С/10 years, in other regions by 0.25–0.29 °С/10 years. Increasing temperature due to climate change contributes to expansion of dry areas and increase of frequency of droughts, which negatively affects crop yields . Due to climate change it is expected that the frequency of strong and medium droughts will change. Precipitation distribution for the territory of Kazakhstan has significant spatial irregularity. The average annual rainfall (without snow) in the territory of Kazakhstan ranges from 130 to 1600 mm and in general rainfall decreases from north to south. In the north oblasts (e.g., Kostanai, Akmolinsk, North Kazakhstan oblast), rainfall is about 400 mm, in the central belt (semiarid steppe zone) – up to 300 mm and in the south (desert) – up to 150 mm. The deficit of precipitation is the most important component of the drought. According (III – VI National Communication 2013) the expected climate change in the near decade will reduce the moisture availability for agricultural crops, enhance of aridity, shift of the zones with sufficient humidification towards northern latitudes and decrease in grain yields.
The system of vegetation monitoring in Kazakhstan is based on traditional methods using the data of ground meteorological network and remote sensing data. Network of meteorological stations is rare and measurements are spatially interpolated across large area. Satellite observing systems do not have these disadvantages, enabling regular monitoring of ground surface in different parts of the spectrum and across large areas, including sparsely populated, inaccessible places. In addition, the accumulated long time series of satellite data allow us to evaluate not only the current state of the vegetation, but variation and changes through different periods. At the present time detection of vegetation changes in the Republic of Kazakhstan, the estimation of the scale of these changes, and the ability to forecast of further development have practical significance and require special attention. Forecasting of future changes is done empirically on the basis of past changes.
9.3.1 The Study Area
Kazakhstan is a transcontinental country, located on the border of Europe and Asia between N40°32′ – N55°16′ and E46°30′ – E87°18′. At 2.72 km2, Kazakhstan is the ninth largest country on the planet. The territory of the Republic is characterized by a latitudinal distribution of natural areas with different vegetation productivity. Most of the country belongs to arid and semi-arid areas: 44% of Kazakhstan territory is desert; 14% is semi-desert; 26% is steppe; 4.6% is forests. Arable land is primarily located in northern Kazakhstan where rainfed agriculture is the dominant land use. Most of the pastures are located in central and southern Kazakhstan. State of vegetation is highly dependent on weather and climatic conditions. The overall goal of the Kazakhstan study is to derive the best possible vegetation cover of Kazakhstan territory including arid and semi-arid zones, which is largely affected by the stress depending on weather and climatic conditions.
9.3.2 Methods
The Long-term series of differential and integral vegetation indices calculated from NOAA and MODIS satellite data of low space resolution (1000 m and 250 m, respectively) for the growing season (April–September) of 2000–2016 were used for monitoring the natural and agricultural vegetation of Kazakhstan’s arid and semi-arid areas.
Vegetation indices, such as the NDVI (Rouse et al. 1973) and the VCI (Kogan 1990), enable monitoring of the state and development of the vegetated land surface at specific periods. They are typically composited in time to minimize obscuring cloud cover, e.g., 10-day maximum NDVI composites. Integrated vegetation indices (IVI) are summarize effectively used for the analysis of long-term changes in productivity of vegetation (Spivak et al. 2012). Index IVI characterizes the amount of green biomass accumulated during the vegetation season and is calculated by summing the NDVI- composites in each pixel. Index IVCI (Integral Vegetation Conditions Index) is used for the analysis of inter seasonal variations of the effect of weather conditions influence on the state of vegetation. Values of VCI, IVCI less than 30% are a good indicator of drought because a decrease of yield is noted equal or more 20% in this case (Kogan 1990).
Vegetation indices used in the space monitoring of the vegetation cover in Kazakhstan are presented in Table 9.1. Time series of vegetation indices calculated from NOAA and MODIS satellite data for vegetation seasons (April–September) 2000–2016 are used to describe the state of the vegetated land surface and its variation and change over large areas (Ogar and Bragina 1999; Spivak et al. 2009, 2017).
9.3.3 Long-Term Changes in the Integral Vegetative Index (IVI) across Kazakhstan
Kazakhstan is characterized by latitudinal temperature and precipitation gradients that define yield zones of vegetation productivity. The vegetation cover of Kazakhstan is also characterized by a pronounced latitudinal sequence of the location of zones of different productivity, associated with the geographical features of the location of the republic. When moving from south to north, there is a change of vegetation types from desert to forest-steppe. Accordingly, the density and productivity of vegetation cover changes.
Zonation of Kazakhstan was carried out according to the values of the normalized integral vegetation index IVI, which were calculated on the basis of satellite data for the period 2000–2016. A similar variant of zoning showed that the selected zones almost coincide with natural geographic zones. Thus, the zone with the values of the normalized index IVI equal to (0; 0.1), practically coincides with desert zones and desert steppes with very low productivity of vegetation; (0.1; 0.2) – semi-deserts with low productivity; (0.2; 0.3) – dry steppe and moderately dry steppe with average productivity; (0.3; 0.4) – arid and moderately arid steppes with moderate productivity; more 0.4 – forested steppe with high productivity. For example, Fig. 9.3 shows the location of five productivity zones over several years with contrasting weather conditions: the growing season of 2002 was one of the most favorable (wet) for the reporting period, 2008 – one of the unfavorable (arid).The quantitative sizes of zones with high and low productivity essentially depend on seasonal weather conditions.
Long-term dynamics of areas of zones with different vegetation productivity are shown in Fig. 9.4. According to the remote sensing data for the period of 2000–2016, there is a negative trend in the areas of high productivity zones; on the contrary, zones with low productivity have tend to increase their area. This principle of zoning showed a strong dependence of the changes of areas of highlighted zones, and their movement in the latitudinal direction due to the weather conditions of each year. In years with favorable weather conditions, a significant increase in the areas of high productivity zones and a reduction in the areas of low productivity zones are observed. At the same time, in the average range of values of normalized IVI (0.2–0.3), no significant changes in the area of the zone occur. In dry years, there is a spatial expansion of areas with low productivity and, as a result, the middle zone moves to the north.
The overlapping of the boundaries of the zone with the values of the normalized IVI index (0.2–0.3) for all the years of observation reveals an interesting pattern. The locations of this zone are determined in the latitudinal direction for different years, differing in weather conditions: for all years favorable for vegetation, the zone (0.2–0.3) is between 46° and 49° N and for all unfavorable (arid) years he lies between 47°30′ and 54° N. The locations of the middle zone with IVI = (0.2–0.3) are shown for examples of wet (2002) and dry (2004) growing seasons (Fig. 9.3).
An IVСI value of 30% indicates drought. Thus, according to the remote sensing data, dry, low productivity years are 2008, 2010, 2012 for Akmolinsk oblast, 2004, 2010, 2012 for the Kostanay oblast, and 2010, 2012 for North-Kazakhstan oblast. Similar decrease of IVI and IVCI values are celebrated for all oblasts of Kazakhstan for period 2000–2016. Values of coefficient in the equations of the linear trend factically shows the rate of decrease of IVCI. Calculation of the coefficients in the equations of the linear trend of long-term changes of IVCI values and zoning of the territory of Kazakhstan by the their values were executed in the scale of oblasts of Kazakhstan (Fig. 9.5a) and of districts of the three north oblasts: Akmolinsk, Kostanay and North-Kazakstan (SKO) (Fig. 9.5b).
As the results of the calculation of the coefficients of the linear trend, the rate of deterioration in the condition of vegetation is more pronounced in the western regions of the republic. There is a positive trend of increasing of zones areas with IVCI <30%, which characterizes the growing impact of drought conditions on vegetation productivity in Kazakhstan. The results of calculation of areas of plots with low values IVCI for some regions of Kazakhstan are shown in Fig. 9.6.
Digital matrices of IVCI index allow visualization of the location of the plots with oppressed vegetation with indication of their geographic coordinates, as well as the definition of the areas of these sites. Digital map of frequency of low index values is formed by long-term values of satellite index IVCI to assess the probability of occurrence of droughts in Kazakhstan. This map was built for the time period of 2000–2016 with annual update. In Fig. 9.7 a schematic map of drought frequency after an algorithm of Spivak et al. (2010) is shown, where according to seasonal digital maps IVCI each pixel is assigned to value of 1, if IVCI <30% (1), and value of 0 assigned, if IVCI >30%; (2) such binary cards are summed over a certain time period; (3) the final map shows the frequency of occurrence of index values less 30%.
Detected areas with a maximum frequency of dry conditions in 2000–2016 are in the map-scheme (Fig. 9.7). These include: (1) the western part of Kostanai region; (2) land on the borders of Akmola and Karaganda oblasts and the East Kazakhstan region; (3) land on the border of Kostanay, Aktobe and Kyzylorda oblasts; (4) Pribalkhashie. High degree of mosaic of the distribution of this index makes it difficult to identify areas subject to arid conditions. In this regard, zoning of the territory of the northern regions of Kazakhstan (regional scale) was carried out according to the values of IVCI <30% for 2000–2016. This made it possible to identify groups of regions with different degrees of risk of arid states (see Fig. 9.8).
VCI values of less than 30% correspond to the stress state of vegetation, characteristic of drought foci. (Kogan 1990). VCI-techniques are mainly used for monitoring of drought conditions in Kazakhstan. A drought can have different impact on the productivity of vegetation. After Spivak et al. (2009, 2017), it was proposed to estimate the intensity of drought on a three-point scale: 0.2 ≤ VCI <0.3 indicates moderate drought; 0.1 ≤ VCI <0.2 indicates severe drought; and VCI <0.1 indicates a very strong drought. Analysis of remote sensing data shows that areas of lots with a very strong damage of vegetation sharply increase as result of increasing drought duration.
Thus, using the example of Kostanay region, the diagram of changes in the characteristics of areas with low VCI values for arid 2012 is shown in Fig. 9.9. A more stringent condition VCI < 0.1 of the time period 2000–2016 becomes significant for the entire territory of the Republic actually since 2006. This fact indicates an increase in the frequency of dry years in the second half of the period under review. This is also confirmed by the negative trend in long-term changes of the integral index IVI, IVCI, both for Kazakhstan and for its individual parts (region, district) (Fig. 9.10).
To interpret the analysis of vegetation indices VCI/IVCI, the results of monitoring of the vegetation state and its dependence on weather conditions according to the data of remote sensing are compared with long-term statistical data on the yield of grain crops. Such a comparison of the time series of crop production and IVCI for the period 2000–2013 was performed for the northern regions of Kazakhstan. It is here that the main part of the production of grain crops is located in the non-irrigation zone of the republic. IVCI values are calculated for May–August of each growing season. The correlation coefficients between the two data sets are calculated on examples of regional and district levels (see Table 9.2).
9.3.4 Spatiotemporal Changes of the Steppes
The steppe zone for Kazakhstan’s agriculture plays an important role. It is here that the main grain lands of the Republic and pastures are located. The steppe zone covers more than two thirds of the Republic territory. These lands are traditionally used for rainfed agricultural production, making them highly vulnerable to climatic variation and extremes.
Climate change scenarios for the territory of Kazakhstan based on the use of the IPСС AR5 model show an increase in average annual temperatures (The III-VI National Communication 2013). For precipitation, the presence of such a clear trend is not noted, but their redistribution is predicted. Such changes have a negative impact on climate-dependent sectors of the economy, especially agriculture. One of the negative consequences could be a reduction in the yield of some crops, in particular, grains. Due to the change in the temperature the boundaries of the moisture zones will shift to the north by an average of 50–100 km and, at the maximum, of 350–400 km. Accordingly, it is projected that the zone of insufficient precipitation, where most of the commodity crops are grown in Kazakhstan (irrigated farming), will increase from 6% to 23%. Experts predict that crop losses of up to 30% are possible due to climate change.
The steppe zone of Kazakhstan is the most vulnerable from among all natural zones in the Republic to the effects of arid conditions, which intensify the processes of aridization and desertification. Space monitoring of the steppe and dry steppe zone of Kazakhstan is of special interest. The object of the research are space-temporal changes in the spectral characteristics of the vegetation cover of the steppe zone of Kazakhstan. To determine the patterns of their changes, depending on the state of the vegetation cover in different agro-climatic zones, including dry conditions, a territory was selected as a transect with a width of 100 km and a length of 730 km (Fig. 9.11). The allotted territory is located on the border of the main grain-bearing oblasts – Kostanay, Akmola, North-Kazakhstan and includes areas with natural vegetation of almost all subzones of the steppe zone of Kazakhstan.
The differences of coefficients in the linear trend equations relatively deviation of IVI from the mean multiyear value for each selected section are shown in Fig. 9.12. The coefficient in the linear trend equation of the multi-year variation IVI can serve characterizes the intensity of the changes that occur.
9.3.5 Summary
The analysis of changes in vegetation indices (differential – NDVI, VCI and integral – IVI, IVCI), computed from satellite data over the territory of Kazakhstan for the period 2000–2016, shows:
-
values of integrated indices of vegetation have an expressed tendency to decrease;
-
an increase in the areas of plots with low IVCI values (<30%) during the observation period is noted;
-
analysis of the distribution of the VCI index for the territory of Kazakhstan for the range (0–0.3), which is an indicator of arid conditions, revealed a positive tendency to increase the areas with low VCI values (<0.1) for the whole territory of the Republic since 2006;
-
comparison of the time series of the integral index of vegetation conditions and yield of grain crops shows a fairly high correlation. Low yields coincide with the droughts (IVKI <30%);
-
according to the zoning of the steppe zone of Kazakhstan on the state of vegetation based on satellite information, since 2004, the growth of areas with low productive vegetation located between 48°N and 53°N parallels has been recorded.
Analysis of vegetation indices showed the intensification of the stress effect of aridity on the vegetation cover of the steppe zone of Kazakhstan in 2000–2016. Such information is important in the study of the processes of aridization and desertification in the Republic.
9.4 Case Study Mongolia
Mongolia is a country of fragile ecosystems, a continental climate, relatively high altitudes and is occasionally described as being quite sensitive to climatic changes (Dagvadorj et al. 2014). Climate Change affects the environment, water supply, desertification and natural disasters that already led to financial, environmental and human losses. An average increase of annual mean air temperatures in Mongolia of 2.14 °C between 1940 and 2008 as compared to 0.85 °C worldwide from 1880–2012 is impressive evidence for the change that Mongolia has already undergone. Increased occurrences of drought, water sources depletion and decreasing biological diversity, all benefiting the process of desertification, accompany these changes (Dagvadorj et al. 2014; UNCBD 2014). The process of land degradation in these arid or semiarid environments is further affected by human activities that may include overstocking and overgrazing, undergrazing at more remote locations, switching from sheep to goat herding or deforestation (UNCCD 2012; Rosales and Livinets 2005; Dorj et al. 2013).
Mongolia’s steppe grasslands include one of the largest grassland ecosystem complexes of the world. Over 70% of Mongolia is built up by three major ecological zones: the desert-steppe, steppe and mountain steppe. These grasslands support most of Mongolia’s domestic livestock (camels, cattle, yaks, horses, sheep and goats). To date, relatively little is known about the vegetation composition and dynamics of these ecosystems. Three national-scale classifications of Mongolia’s vegetation (Hilbig 1995; Ulziikhutag 1989; Yunatov 1977) are available, of which only the first is in English. The scientific data situation about the response of Mongolian grassland vegetation to increasing grazing intensity or the effect of grazing removal is scarce. A few studies (Chogni 1989; Tserendash and Erdenebaatar 1993) concentrate on the impact of grazing intensity or removal grazing, but these studies were mostly conducted in the mountain-steppe and steppe zones in the center or north of Mongolia. Studies about grazing and climate influences on the composition of desert-steppe grasslands in southern Mongolia are so far non-existent. Moreover, Mongolia as a country at the southern edges of the Eurasian cryosphere, is a country partly under the influence of permafrost. Three basic forms of frozen soils (permafrost, sporadic permafrost, and seasonal frozen soil) exist in Mongolia. The spatial distribution of these “permafrost types” is determined by annual mean air temperature. Frozen soils may have influence on the phenological shifts of dry and cold grasslands in Mongolia (Sun et al. 2015). Frozen soils play a decisive role in storing water from precipitation of the previous autumn for the subsequent grass green-up in the next year.
In contrast, long-term (1982–2010) NDVI development in the region, evaluated using AVHRR (1982–2006) and MODIS (2000–2010) data, describe an upward trend in vegetation greenness until the mid to late 1990s (Bao et al. 2014). During the 2000s this trend apparently reversed in coherence with a significant decline in precipitation in the same period. The authors attribute the decline on 60% of the landmass to a hotter and drier climate that is associated with drought stress. (Eckert et al. 2015) reported mostly positive significant trends on 50% of Mongolia’s surface between 2001–2011 using the MODIS 16-day composite vegetation indices product. They also conclude that precipitation changes during this time are related to changes in NDVI, though identifying further areas where deforestation and mining are predominant factors in declining NDVI’s. The largest part of the country has none or a positive trend, while negative trends are clustered to the far west and the Dundgovi province. The study could however only identify significant trends for about 50% of the country due to the relative short time-series. Chu and Guo (2012) used a similar 12-year MODIS time-series from 2000–2011 to characterize vegetation responses to climate change in the Hövsgöl area, northern Mongolia. They concluded that the growing season that normally starts in late April and ends in late October, with full growth in July, does begin earlier in more recent years. They therefore assumed that NDVI dynamics in the research area are mainly controlled by the pattern of temperature variations rather than due to precipitation. Purevsuren et al. (2012) used SPOT VEGETATION data for a short time-period (2003–2009) and they also detected a slight increase in NDVI during this time-span. They also concluded that NDVI and precipitation are well correlated in wet years but quite weak during dry years, if at all. Several studies exist in which the suitability of NDVI is raised for various vegetation related changes and responses in Mongolia (Nandintsetseg et al. 2010; Zhang et al. 2009; Iwasaki 2009; Adyasuren et al. 2005; Javandulam et al. 2005) or Central Asia in general (Yu et al. 2003; Yu et al. 2004; Vostokova and Gunin 2005; Huang and Siegert 2006; Dulamsuren et al. 2016).
A common feature of these studies is the use of rather short time-series (< 10 years), the use of similar remote sensing products (mostly MODIS and GIMMS) or that the end of the considered time-series lies somewhere between 2006 and 2010. As such we will evaluate if there is again a trend reversal towards a positive NDVI development in Mongolia as indicated by previous studies mentioned above. To achieve this we incorporated more recent years of available NDVI data of SPOT-VGT data in combination with the ERA-interim climate data-set in a comparably high resolution of 0.125 degrees and a modern 4D-var sampling technique. The driving environmental factors for the purported changes are then assessed and discussed. The assessment is done on an annual and monthly basis.
9.4.1 Study Area
Mongolia is situated at the northern fringe of Central Asia in the transition zone between the Siberian Taiga to the north and the Gobi Desert in the south (Fig. 9.13). Spatially it extends from 87°45′E to 119°56′E and from 41°34′N to 52°09′N and covers a total area of 1,562,950 km2. The wide basins of the interior drainage system lie at elevations between 900 and 1500 m asl with the lowest areas below 720 m asl. The mountains are shaped by pronounced flat planation surfaces in elevations between 2500 and 3500 m asl, while the highest peaks rise above 4000 m asl. There are four main mountain regions in Mongolia. The Mongolian Altai in the west (highest peak is Tavan Bogd, 4374 m asl), the Gobi Altai in the south (Ikh Bogd, 3957 m asl), the Khangai Mountains in the center (Otgon Tenger, 3964 m asl) and the Khentei Mountains in the northeast (Asralt Kharj khan, 2799 m asl). Modern glaciation occurs exclusively within the Mongolian Altai with a small ice field at Otgon Tenger as the sole exception.
The largest lake in Mongolia is Uvs Nur (3585 km2), which is situated in the northern part of the “Valley of Great Lakes”. Large lakes like Khiargas Nur (1365 km2), Khar Nur (918 km2) and Khar Us Nur (934 km2) are distributed throughout this north to south trending endorheic basin too. This catchment area lies east of the Altai mountain range, which is drained by the Khovd Gol, and in the west of the Khangai Mountains, which are drained by the rivers Tes and Zavkhan Gol. Large dune fields exist in the east of the lakes. The west to east trending “Valley of Gobi Lakes” with Bon Cagan Nur (269 km2) and Orog Nur (106 km2) lies between the Khangai Mountains and the Gobi Altai in the south. Lake Khovsgol Nur (2723 km2) in northern Mongolia drains to the Selenge river, which together with the Orchon river is flowing into lake Baikal in Siberia.
The climate of Mongolia is characterized by high continental semi-arid to arid conditions. In wintertime the Siberian high pressure cell produces cold and dry weather with few snowfall and average temperatures between −15 and −30 °C. The main rainy season occurs from June to August during a short summer period, induced by westerlies and cyclone precipitation, but is soon ending when the dry season starts again in autumn. Mean summer temperatures range between 10 and 27 °C. The mean annual precipitation is below 50 mm in the interior basins, around 125 mm in the southern desert and up to 350 mm in the northern steppes, while it increases to more than 500 mm in the high mountains. There is a great annual variation of precipitation in the amount and spatial pattern which is strongly influencing the density of the vegetation cover (Barthel 1983). In accordance with these climatic environments a latitudinal zonation of the vegetation exists, which is modified by an altitudinal zonation in the relief (Fig. 9.1a). In northern Mongolia taiga vegetation with mixed needle and deciduous broadleaf forests occurs. The vegetation belt in the middle of Mongolia consists of steppe, crop- and grasslands in the basins and forest steppe in the mountain area. In this forest boundary ecotone of semiarid climate conditions the relief is controlling the spatial vegetation pattern. While the deciduous needleleaf forests, consisting of larch trees (Larix sibirica), are exclusively growing on northern aspect slopes, the south facing slopes are covered by steppe vegetation. The southern part of Mongolia consists of desert steppe and sparse desert vegetation. Sand dune, playas and takirs are widely distributed. In the high mountains dense alpine meadow vegetation occurs between forest steppe and the periglacial zone of frost debris. The main perennial rivers are accompanied by floodplain meadows and forests.
9.4.2 Materials and Methods
SPOT VGT satellite data: The satellite data used in this case study primarily consists of SPOT VEGETATION 10-daily NDVI atmospherically corrected composites at 1 km resolution for the time January first 1999 to December 31st 2013, equaling 540 distinct time-steps. In addition, the SAVI was calculated from raw SPOT-VGT reflectance data to verify the NDVI signal in areas with sparse vegetation (Huete 1988). These were re-sampled to monthly rasters using the maximum value of all three. The annual means were calculated from the 12 re-sampled rasters. In a first step pixel based NDVI trends were calculated by estimating the coefficients of a linear regression where the Mann-Kendall trend test indicated a significant trend.. As the sole consideration of monthly NDVI values and annual means might not be sufficient, a seasonal evaluation of NDVI changes was additionally executed. This includes the consideration of the winter months only (November–March) to detect possible NDVI changes that occur when no vegetation is present and those months that can be generally described as the growing period (May–September) (Dagvadorj et al. 2014; Wang et al. 2007; Suttie and Reynolds 2003). For each combination, the linear regression and the Mann-Kendall test were calculated on a pixel-by-pixel basis. For each pixel the best combination (lowest p-Value, most positive or negative r-value) and the corresponding value are determined and logged.
AVHRR satellite data: To evaluate the validity of the SPOT-VGT data-set it was compared against the GIMMS AVHRR Global NDVI-3G dataset. The AVHRR data was atmospherically corrected as well and features a resolution of 0.0833 degrees, making it more coarse than the SPOT-VGT data (by about a 1D factor of 10). For a direct comparison in the form of a regression analysis the AVHRR data was re-sampled to match the resolution of the SPOT-VGT data. In a first step both datasets where cropped to the same extent before the AVHRR data was actually re-sampled using a spline interpolation by the order of 3. The original dataset covered the time from 1982–2012 in a bi-monthly resolution. The monthly maximum values and from this derived annual means from 1999–2012 were then used for the comparison. If p-values are mentioned in regard to the comparison of both data-sets, these refer to a simple two sided t-test with H0 being the slope of the regression line is equal to zero. Significance is assumed for α < 0.05, meaning that if p < 0.05 the correlation at a certain pixel is considered significant.
Climate data: The dataset used to investigate climate induced changes to the NDVI is the ERA-interim data-set (Berrisford et al. 2011; Dee et al. 2011). This was chosen over the NCEP/NCAR Reanalysis products for its higher spatial resolution (here 0.125 degrees) and more modern sampling technique, as the longer temporal series of the NCEP, going back until 1948, was not necessary for this approach. We did use monthly data from January 1999 until December 2013 to cover the same period as our NDVI data-set. Temperatures were calculated from the “Monthly Means of Daily Means”, precipitation from the “Synoptic Monthly Means” section of the ECWMF website as no pre-computed daily means were available. The daily 8 synoptic mean values were added to form a monthly mean value. This was done by adding together the synoptic monthly means at time 00, step 12 and time 12, step 12, as suggested by the ECWMF. The original ERA-interim units for temperature (Kelvin) and precipitation (mm/day) were maintained. A possible source of error might be found in the context of the used climate dataset. Though the confidence in this kind of data has steadily risen in the past years and it is more often regarded as equivalent to observational data, this is not always justified (Dee et al. 2011). Validation of ERA-interim data for Mongolia is however sparse. A comparison of soil moisture on the Mongolian Plateau did show that ERA-interim data is capable of representing temporal dynamics, though slightly overestimating it (Wen et al. 2014). Global assessments of the quality of ERA-interim precipitation data (Lorenz and Kunstmann 2012) conclude that it is still the most reliable data-set in areas with a large number of gauges. Though this is not given to the same extent in Mongolia as in Europe or North America, regions that were pointed out as problematic were mostly parts of Africa and South America.
We included a validation of the ERA-interim dataset used in this publication with monthly data from 4 weather stations in Mongolia (Matthew et al. 2012). Figure 9.14 displays the monthly correlations between both data sources. From this, it should be clear that the ERA-interim dataset is well suited to depict the temperature development in the study area. For precipitation it is always more difficult to be reliably reproduced in models, which is also the case for ERA-interim. Though not as good as for temperature, the precipitation data is in general agreement for Bulgan or Choir, a bit worse for Dalanzadgad station and worst at the Altai station. The agreement for all stations mostly diminishes when precipitation becomes rather low, in these cases ERA-interim sometimes returns precipitation while none was reported by the station data. The rather weak correlation for the Altai station was also to be expected, as apart from the low overall precipitation, relief influences complicate its translation into the ERA model.
Mann-Kendall trend test: This study combines two approaches to determine the significance and strength of existing trends. The Mann-Kendall (MK) test for trends is first applied to determine which locations have a significant trend. MK can assess if any monotonic trend exists within the data (positive or negative, without regarding it to be a linear trend) and is also a non-parametric test. We assumed existing trends for p < 0.05.
As Mann-Kendall only ascertains the level of confidence that any trend is existent within the data, it does not give information on its strength or direction. We therefore used the r-value of a linear regression to approximate the strength and direction of each pixel where MK was significant.
Exemplary Points A–E
As a map-only depiction of trends hides the underlying changes and is limited to 1 value per pixel, we chose 5 exemplary points in Mongolia to highlight annual values and their progression. Figure 9.15 shows where these points are located, Fig. 9.23 gives in-detail NDVI, temperature and precipitation information for each point. All points where chosen at locations with strong and highly significant trends. After identifying these locations, the exact points where chosen in their respective centers. Points A and B lie within the areas of strongest NDVI decline. While A was chosen for its location at the largest absolute NDVI decline in Mongolia, B lies within the largest contiguous area of decline. C is located in the vicinity of A and B, but shows a significantly positive NDVI trend, and can as such be seen as a contrast to A and B. All three points are located in an area that is dominated by forest. D and E were chosen for their location in the center of the largest continuous areas of positive NDVI trends in Mongolia. While D lies in the midst of the desert, E lies in a transition zone commonly labeled as Desert Steppe.
9.4.3 Results
As a consequence of the prevailing arid climate , Mongolia consists of vast areas of land that stay well below annual average NDVI values of 0.2 (see Fig. 9.15). About 48% of the total land area are even below 0.1 while less than 4%, mostly within the northern mountainous area, show annual NDVI averages of >0.3. Thus even small differences on the annual scale might be an indication for an apparent change within the area. A rough comparison of the first (1999–2005) and subsequent half (2006–2013) of the time-series gives an initial impression of supposed NDVI changes. In this comparison (Fig. 9.15) almost all of Mongolia shows an increase in annual mean NDVI, on average by 0.02. Parts with a negative development are scattered throughout western Mongolia and to a lesser extent in the far east. However, these declines are mostly fairly below −0.10. Another small area (about 620 km2) in the Selenge province north of Ulaanbaatar shows an average decline in NDVI of around −0.10 that is selectively as low as −0.27. To give a better impression of the inter-annual dynamics, results were not only calculated spatially but also for five point locations (compare Fig. 9.15 and Fig. 9.23).
9.4.4 Annual and Monthly NDVI Trends
Though small in absolute numbers, the NDVI in Mongolia generally shows a rising trend during the presented time period, thus Mongolia is getting greener on average. This paragraph’s scope is to determine if this is a significant trend or rather an anomaly, if it is valid for entire country or only parts and if it is detected throughout the year or just on a monthly basis. While Fig. 9.15 gives an impression of the absolute change in NDVI, around 0.01–0.08 between both half’s of the time-series, Fig. 9.16 describes trends on an annual basis. This was done spatially on a pixel by pixel basis. A Mann-Kendall test to determine significant trends within the data (top), regardless of strength and direction, was applied first to account for trends in general.
To give an impression of the quality and direction of trends a linear regression was conducted (middle). Finally, both calculations were intersected (bottom) so only r-values at locations with significant trends remain in the map. A significant annual trend is detected on about one third of the Mongolian landmass. In accordance with the basic mean value comparison between 1999–2005 to 2006–2013 these trends are mostly positive. The only coherent area with a negative development is the aforementioned area north of Ulaanbaatar with r-values generally well below −0.5. However, the other areas with a slighter negative development, e.g. those to the west, do not show up as being significant.
The strongest and most extensive indication of a positive NDVI development can be found in the Gobi desert, namely in the south of the provinces Govi-Altay, Bayanhongor and Ömnögovi as well as the northern part of Dornogovi. Most of the area south of Govi-Altay is a strictly protected area (national conservation area or national park). Here most areas show Mann-Kendall p-values <0.01 (highly significant) an r-values >0.8. Locations D and E are located in these areas. Though the changes during these 15 years are small in absolute numbers, they are quite obvious and well enough described linearly. If trends are estimated on a monthly basis (Fig. 9.17) it becomes evident that the described annual trends can largely be attributed to positive changes in the time from March to June with average r-values of 0.66. These are closely followed by July to November (also February) with average r-values of 0.62 while December and January averages around 0.48. April is clearly the one month with the strongest and most widespread uptrend. Not only are the average r-values at 0.68 the highest of all months but it is also the month with the largest area of significant changes in NDVI. Half of Mongolia is affected, almost twice as much as in March, May or June, while >99% of these areas show a positive trend. Interestingly, there is even a significant increase in NDVI from December to February, mostly in the southern part of the country. The trends are comparably weak and cover only small areas but are clearly present in the data. This is interesting, as temperatures in these areas are well below freezing during this period and one would not assume large scale vegetation growth in this period.
9.4.5 Seasonal NDVI Trends
If the months are combined into seasons, e.g. winter (November–February) and growing season (May–September) as shown in Fig. 9.18, considerable trends exist throughout the growing season. About 40% of Mongolia is affected by a mostly positive trend which is distributed throughout the entire country. Though sparse, significant trends occur during the winter season as well. Then only about 5% of the country is affected with a clear concentration on the southern desert parts.
9.4.6 SAVI Changes and Trends
The SAVI was calculated from raw SPOT-VGT reflectance data to verify the NDVI signal in areas with sparse vegetation like the Gobi desert, because background soil brightness can heavily influence NDVI values (Huete 1988).
A cross-validation of the SPOT-VGT NDVI with SAVI values (see Fig. 9.19) mostly agrees with the NDVI changes. There exists a significant correlation between both indices for 78% of Mongolia, of which >99% represent a positive correlation (r > 0.5). There are two larger areas where the SAVI returns a different signal than the NDVI: namely in the southern desert areas, as well as the eastern part of the Dundgovi province. However, if the SAVI data is probed for trends using the Mann-Kendall trend test, significant SAVI trends are only detected for about 13% of Mongolia as compared to 33% for the NDVI during the same period. This area reduction is most prominent in the southern desert and steppe areas.
9.4.7 Climatic Trends
The scope of this paragraph is to address presumed climatic changes during the 15 years that are used to analyze potential influences on a locally changing NDVI. The presence or lack of any trends during these years should not be regarded as a description of a long term climate change in Mongolia, as this would at least involve a testing against 30-year climate normal. It should be noted that since 1940 Mongolia has undergone an extensive rise in temperatures (+2.14 °C) and region specific changes in precipitation (1).
Between 1999 and 2013 Mongolia as a whole features no significant trends in temperature or precipitation on an annual basis (Fig. 9.23 bottom right). However, some parts of the country do (Fig. 9.20). The annual mean temperature trend is here negative in general, meaning that it is getting slightly cooler. This affects only a few distinct clusters throughout Mongolia with an average decline of 0.08 K per year. The precipitation trend is concentrated in a more contiguous north-south corridor in the center of Mongolia, though two smaller spots in the west and far east exist. Only the small far eastern part shows increasing precipitation while the larger areas get drier. Figure 9.23 gives a more detailed description of the annual sequence of temperature, precipitation and NDVI at five distinct locations as well as for the Mongolian Average. For none of the five chosen points a significant trend in temperatures exists (see also Table 9.3 – right side). However, sites B and C do show a significant downtrend in precipitation for α = 0.05, while site A only slightly fails to be significant. However, the downtrend at site A is still stronger than at D or E. At location A and B, though still relatively weak, there is also a correlation to be found between NDVI and precipitation (r2 = 0.27 and r2 = 0.23). Especially at A the role of precipitation as the major influence on NDVI seems quite unlikely. Not only is the decline in NDVI incomparably steeper, but even gets contradictive from 2007 to 2009 when precipitation is slightly rising and NDVI values decline drastically from 0.46 to 0.29. This also constitutes the largest difference between any 2 years at the selected locations. At B the downtrend in NDVI is more moderate but still not really explicable through changes in temperature or precipitation. For example in years with a local minimum in precipitation (2005 or 2010) the NDVI is actually rising.
Figure 9.21 gives an overview how climatic variables and NDVI values are connected during years 1999–2013 within the different vegetation zones. 1000 random samples were distributed throughout every vegetation zone and probed monthly for their NDVI, temperature and precipitation. We further sub-divided the points into three groups of 5 years each, to highlight changes in the shown relationships during our period of interest. However, there is no clear difference present, though the maximum of precipitation is a little higher in the earlier years in the Desert and Desert Steppe and the years 2004–2008 have slightly elevated maximum temperatures.
Scatterplot between NDVI values and precipitation (left) and temperature (right) by general vegetation units for the main vegetation period (May–September). Each vegetation unit is represented by 1000 randomly distributed dots, where each dot represents the growing periods average NDVI vs. average climate variable. Points are distinguished into three different time periods, given R2 values represent the entire 15 years
The strongest relationship between precipitation and NDVI can be found in the Steppe areas, followed by the bordering Desert Steppe and Forest Steppe. While desert areas seem to indicate a relationship as well, their values are generally too small, and as such within the error margins of the NDVI and precipitation datasets, that no strong relationship is apparent. Alpine Vegetation shows a very weak NDVI response to precipitation increases while Subtaiga and Dark Taiga are more or less free of direct influences. However, these last two, and here especially the Subtaiga, show an increase in NDVI with increasing temperatures. This is similarly true for Forest Steppe. The Desert Steppe also shows a connection, which this is directed downwards, meaning that increases in temperature here lead to a reduction in NDVI. It should be noted however that there is a considerable amount of noise, and while these connections exists, they are relatively weak.
Lastly locations D and E are both located within a warmer and dry environment, though the climate at E can be interpreted as slightly milder. The significant rise in NDVI values is visually perceivable and not linked to the annual behavior in precipitation patterns. No correlation exists between the two parameters at either of both locations. This is especially remarkable for D, as in the years 1999–2002 about no NDVI signal at all is detected, combined with a lack of precipitation. From 2003–2008 a ‘normal’ and expectable pattern can be seen: even slight precipitation leads in the year or in the year to follow to an emerging NDVI. After 2009 however, though about no annual precipitation is detected, NDVI values continue to rise to a frail 0.04 annual average in 2013. The site at E behaves similar, however the slightly higher precipitation causes also higher NDVI values. By pure correlation there seems to be no connection between precipitation and NDVI pattern at neither of the two sites. Figure 9.22 shows trends distinguished on a monthly basis. Generally, temperature changes only occur from January to July, varying largely in their spatial extent and location. With the exception of March and April, which have the smallest area featuring a trend (only at 3 or 6 pixels), these temperature trends are negative, i.e. it is getting colder. Monthly precipitation is decreasing at most significant trend sites, when April, May and August are largely affected. In May, almost a quarter of the country sees a decline in precipitation of up to −0.1 mm/day p.a. Furthermore, one must consider Dzud-events, extreme winters and following summer droughts, as at least three of those are reported during the time-span of this study (1999–2002, 2005 and 2009). Dzud events have a severe impact on the present vegetation (John et al. 2013). However, these events are not clearly visible in the presented climatic data, possibly obscured by the computation of annual means using January to December instead of a winter to summer mean. These Dzud events however appear to be visible in the SPOT-VGT NDVI series, even for the Mongolian annual average (Fig. 9.23).
Trends of average precipitation values from 1999–2013 by month. Each month shows the r-values of a linear regression where Mann-Kendall is significant for α = 0.05. Gray overlays indicate temperature trends, while the gray arrows explain if the monthly temperature trend is generally positive, negative or absent
9.4.8 Comparison of SPOT VGT and AVHRR
AVHRR NDVI data is used to validate the SPOT-VGT signal over the area of Mongolia. Figure 9.24 gives an overview on trends that can be detected using AVHRR. Long-term positive trends (1982–2012) only exist on 0.1% of Mongolia.
When NDVI values of SPOT-VGT and AVHRR are compared against each other on a pixel-by-pixel basis their annual means (see Fig. 9.24) show a positive correlation (r > 0.3) on 61% of the Mongolian territory. 31% of all pixels do not seem to correlate at all (0.3 > r > −0.3) and only 8% are negatively correlated. These correlations are significant for 40% of all pixels when α = 0.05 and would only see a negligible rise to 50% if α was assumed to be 0.1. Generally, both data-sets agree well in the northern part of Mongolia, where in most provinces >70% of all pixels correlate positively. The best agreement can be found in the north-east (Hentiy and Sühbaatar region) with >95% positive correlation. Here almost all of these correlations are continuously significant. Greater areas with no disagreement are found on a north-west to south-east axis through the country, with the most western Sum, Bayan-Ölgiy, featuring nearly 60% of pixels with no agreement. However, this is the only province with a disagreement on more than half of its area. The two next biggest provinces, Hovd and Govi-Altay, show a disagreement on 45% of their area.
However, there exists a relatively small stripe of significant and strong negative correlations as well in the south western borderlands of Mongolia, namely in the south of Hovd, Govi-Altay, Bayanhongor and Ömnögovi. This correlation is almost entirely significant and generally features r-values <−0.5. This means that the strong positive trends that are described in this area using SPOT-VGT are not present in the AVHRR data. Even worse, the AVHRR data shows a strong negative trend in this area, even if the longer time series 1982–2012 is regarded.
9.5 Discussion
While, based on the SPOT-VGT data, Mongolia is getting greener on average, the question remains as to why? No clear correlation between annual climatic averages and the inter-annual NDVI changes could be established. Even more, if a precipitation trend is detected at all, its general direction is downward, not upward as for the NDVI. However, as we could show in Fig. 9.21, NDVI and precipitation are positively correlated in the Desert Steppe and Steppe, which is exactly where we detected large areas of NDVI increases. No significant precipitation or temperature changes could be detected on an annual basis. As annual averaging might conceal intra-annual changes we also applied a monthly climate analysis. While most months during the vegetation period from May–September show no significant climatic changes in places where NDVI trends were detected, July does. In eastern Mongolia, declining temperatures happen along with a small core area of rising precipitation. While the area of significant precipitation changes is too small to account for all of the NDVI rises alone, a decline in temperature could be the dominant factor here. Figure 9.21 shows a very weak negative correlation between the Desert Steppe NDVI and temperature values. Declining temperatures will reduce evapotranspiration and lead as such to an increased water availability. As precipitation or water availability is clearly the dominating factor in these zones, increases in water availability, regardless of the source, are bound to increase the NDVI. This effect may also be responsible for the small scale NDVI increases on the eastern slopes of the Gobi Altai, where July temperatures are decreasing as well and no change in precipitation pattern could be discovered.
While this explains the NDVI rise in eastern Mongolia and the eastern Gobi Altai, the other region of large scale increase, the southern desert region, shows no climatic changes, even when evaluated on a monthly basis. Furthermore, while the NDVI trend in the east could be confirmed using AVHRR data, this not only fails in the south, but both sensors are diametrically opposed in their results. Also, this trend is not apparent in the SPOT-VGT SAVI data (Fig. 9.19), which is much more suitable for areas of sparse vegetation. As such, before diving into possible environmental reasons for a greening, the robustness and validity of the SPOT-VGT NDVI time series should be put under scrutiny. A single sensor may create biases of its own, which is why we applied a comparison to data derived from AVHRR. As they show a strong agreement for 61% of Mongolia during their time of overlap (1999–2012) we assume that the positive trend in the eastern part is not an artifact from the SPOT sensor. Though the long-term downward trend seen in AVHRR seems to turn into a positive trend in recent years, we acknowledge that a time-series of 15 years (SPOT-VGT) may be too short to capture any sustainable trends. An example for this might be a study from (Zhao and Running 2010) that reported reductions in global carbon between 2000–2009, which was later shown to be due to artifacts in their NPP model (Samanta et al. 2011). The matter becomes even more crucial when we have to consider that the SPOT-VGT data product is a combination of two different sensors, for which it has been reported before that the break between these, which occurred in 2003, resulted in a more positive NDVI trend as compared to MODIS or AVHRR (Fensholt et al. 2009). SPOT-VGT NDVI values before 2003 seem to be systematically lower. If only the data from the more recent SPOT-VGT2 are considered, overall significant trends are reduced, as can be seen in Fig. 9.25.
This almost entirely cancels the positive trends found in southernmost Mongolian desert. NDVI values are reported to be 3,4% higher in SPOT-VGT2 as compared to SPOT-VGT1 due to their different spectral response functions (Fensholt et al. 2009). This difference seems to become much more severe around NDVI values of 0 though, as Point D in Fig. 9.23 illustrates, where NDVI values are generally <0 before 2003 and generally >0 after. Therefore we conclude that the SPOT-VGT time-series from 1999–2013 is not reliable for areas of very low NDVI and at least biased towards positive trends for areas of larger NDVI.
We therefore conclude that there is no strong positive NDVI trend in the southern desert area, but rather an artifact from the break between two sensors. Though there might be an increase even if the data before 2003 is excluded, as displayed in Fig. 9.26 at site D, it is not strong enough to be significant. However, the eastern part of the Gobi Altai, large parts of eastern Mongolia as well as the seamline between desert and steppe in the south, retain a strong positive trend during these 10 years that cannot be explained by the shift between the 2 sensors. As such, we assume that regardless of the drawbacks due to the sensors flaws, there are significant NDVI trends in Mongolia that are mostly positive.
A third area of interest is found primarily north-east of the capital Ulaanbaatar, where significant positive and negative NDVI trends are found close together in the Sub- and Dark Taiga. This area is part of a belt that runs through the center of Mongolia, in which precipitation significantly declines on an annual basis. Mainly the months of April and July show the greatest declines. However, as shown in Fig. 9.21, NDVI values in these regions are predominantly affected by temperature rather than precipitation and there are no large-scale temperature changes that might be responsible here. As environmental factors seem far-fetched to explain the changes happening here, we would like to present another possibility. This greater area, as it harbors more than half of the Mongolian population, is predestined for being under a strong human influence. As the area consists mostly of forest, Eckert et al. (2015) describe that forest fires in this region are a main reason for NDVI changes similar to those described in our study. Points A, B and C (Fig. 9.23) are single pixels in this area, all located within a 50 km radius, that allow for an inter-annual analysis of the events. A and B feature significant NDVI declines, while C shows significant increases. For A and B a one-time event, as would be evidence to a forest fire, is not visible in the data. For example Site B consists of a rather continuous decline in NDVI. Site A does show signs of a single event as the NDVI drops drastically between 2 years 2007 and 2009. Here the question remains as to why the NDVI drops in 2 consecutive years if a single event is responsible? To take a closer look at the environmental changes at these locations, Landsat images of several years are compared in Fig. 9.27. As of August 2002, the area seems more or less undisturbed. Until June 2003 a small forest area east of location A gets lost to what looks like a fire event. This overall picture remains until May 2007. Unfortunately, the images of the crucial year of 2008 were not available. However, in 2009 a large fire event around location A becomes visible. The last image of July 2007 does not show these burnt-down areas, however the image quality is rather bad due to clouding. In accordance with the NDVI data it is also more likely that the fire event took place in 2008. This would explain the 2-year-drop in NDVI, as e.g. a fire in August 2008 would mean high NDVI values before and low after the event, thus averaging in the annual perspective to a value between that of 2007 and 2009. As it turns out the location of B was chosen rather “lucky”, as it is located right between two major fire events. One occurring between May and July of 2007, where a large area south/east of B was affected. Between 2007 and 2009 another large event did happen to the north/west. Both fires might have only partly affected the NDVI development at site B what might explain the lack of sharp inter-annual drops. While negative NDVI changes in the greater vicinity of both sites seem to be a consequence of forest fires by large, logging done by humans might aid to the general trend of deforestation.
Locations A and B in Landsat images for different years. Red rectangles indicate the extent of one SPOT-VGT NDVI pixel (i.e. the area within each rectangle is used for the values in Fig. 9.22)
C and similar sites in the vicinity are interesting for their strong positive trend while being surrounded by areas of absent or negative change. Even more, there is a steady and significant downtrend in precipitation and no trend in temperatures, while the NDVI is steadily increasing. All considered, it is highly unlikely that solely changes in the climatic parameters are responsible for this change. The causing factor might be related to human activity, normally leading to deforestation though.
What is remarkable is the close distance to the Gorkhi Terelj National Park. In the years 1995–2002 the German GTZ (now GIZ, the German government’s program for technical cooperation) established an initial program “Nature Conservation and Buffer Zone Development Project” in the area. Until at least 2008 several related programs did follow. Within those, fire management concepts were established with local communities that were later expanded to account for climate change and biodiversity. In the course of these programs forest user groups in the Mandal Sum developed a management plan that was approved by local authorities (Jamsranjav 2012). This area coincides very well with the area of detected NDVI rise in recent years. The human influence here can also be seen in official data from 1998. It suggests that only one fifth of the forest area here could be classified as natural forest. Another fifth is logged, another open stand and a close quarter of forest area burned (the remaining constituting of shrubs and regenerating forest) (Tanaka et al. 2008). The potential to improve forest quality and quantity through elaborated management plans is thus largely present and seems to be a good explanation for the positive NDVI development in a rather highly populated area. However, as of now, no local observations are available to validate this hypothesis. Optical evaluation of Landsat imagery for sample site C is also inconclusive.
While we discussed the significant changes in NDVI, we mostly omitted the fact that almost all of Mongolia shows some positive NDVI response in the years since 1999. Though this is often not significant, probably due to the shortness of the time-series, there still seems to be a shift towards an increased greening. This is also present in the AVHRR time-series, which shows a long-term down-trend in NDVI but indicates a turning of this in recent years. Other publications have noticed this shift as well (Eckert et al. 2015) and reported it for up to 50% of Mongolia. Apart from the local climatic factors, we want to argue for another and perhaps more subtle underlying effect that may partially cause this emergent greening: atmospheric CO2 and its associated fertilization effect. Several studies indicate a connection between rising atmospheric CO2 and plant growth in arid environments (Berry and Roderick 2002; Higgins and Scheiter 2012). In the years from 1999 to 2013 global mean CO2 concentrations rose quite linearly from 368 to 397 ppm, or roughly 2 ppm per year (Scripps 2016). A correlations of atmospheric CO2 levels with NDVI values at site and E returns an r-value of 0.83. As the vegetation in the affected areas mostly consists of grasses which are C3-plants (Ehleringer and Cerling 2002), an increase in biomass through elevated atmospheric CO2 levels is feasible. This trend will continue for some time, as it is expected that these plants might benefit from CO2 increases up to 1000 ppm. Furthermore, the water saving aspect (increase in water-use-efficiency) due to elevated CO2 can be strong enough to counteract the otherwise negative effects of water scarcity (Morgan et al. 2004). As we could show, precipitation or water availability is directly affecting NDVI values in all of the Steppe areas (Fig. 9.21) and as such, an increased water-use-efficiency should lead to increasing NDVI values.
There are examples where even under increased summer drought stress some Grasslands showed an increase in biomass simply due to changes in the surrounding CO2 concentrations (Taube and Herrmann 2009). Especially within warm and arid environments, where water plays a crucial part for primary production, the CO2-fertilization effect should be particularly pronounced. Donohue et al. (2013) showed that an increase in atmospheric CO2 in warm and arid environments of 14% between 1982–2010 helped to increase green foliage cover on a global average by 11%. This increase did also occur within their study in southern Mongolia and they concluded “that the fertilization effect is now a significant land surface process”.
One study for northern China seems to indicate indeed a trend reversal in more recent years, as NDVI data from 1982–2010 shows close to zero trends but a decrease in earlier years (Peng et al. 2011). However, there is still no clear consensus at what is currently happening as a study covering the period 1982–1999 reported a significant rise in NDVI (Piao et al. 2006) while another reported a decline, if 1997–2006 is regarded (Park and Sohn 2010). In a global study by Zhu et al. (2016) Mongolia is among the few countries that saw a negative LAI trend for 1982–2009, which was attributed to negative climatic impacts. At the same time 25–50% of the global vegetated area did show a greening that was directly attributed to CO2 -fertilization effects. As such, we might now see that the fertilization effect is now beginning to surpass the impact of detrimental climatic changes of the past 30 years. If this holds true, this initial greening we described above will continue in the future and will then be detectable with much higher confidence than we are able to now due to the shortness of the NDVI series.
9.6 Summary
While long-term NDVI trends in Mongolia, typically analyzed using AVHRR starting from the early 1980s and MODIS data after 1999, have often been described as negative, we could show that this is not true anymore in recent years. We could identify positive significant trends in small areas around the capital Ulaanbaatar that we attributed towards a change in human practices within these forest areas. Near these locations the only significant NDVI declines for Mongolia could be identified as well. We attributed these to fire events that temporarily reduced the forest cover. A rather large scale rise was identified in eastern Mongolia, where increasing precipitation and decreasing temperatures in July increased water availability and as such NDVI values. We also attributed the smaller scale increases on the eastern slopes of the Gobi Altai to decreasing July temperatures, though the connection here is weaker. Apart from theses significant changes, weak NDVI increases can be found in other parts of Mongolia as well. However, they fail to be statistically significant and as such must remain speculative. We attribute this in part to the general shortcomings of trend studies and the length of our datasets in particular. While the significant trends we described above are found under almost all tested conditions, many other trend locations disappear if the length of the NDVI series is changed (Fig. 9.24 a-b), different sensors are considered (Figs. 9.16 and 9.24), only the most recent SPOT sensor is analyzed (Fig. 9.25) or if different indices are considered (Fig. 9.19).
9.7 Outlook
The case study from Mongolia shows that there are major research gaps existing in relation to landscape dynamics assessments in Central Eurasia. The development of a new method for ecosystem state assessment that combines breakpoint analysis (tipping points), ecosystem response categorization and traditional trend analysis is needed.
A GIS based mapping and assessment tool that can serve for largescale assessment of change in ecosystem functioning that enables the detection of turning (tipping) points in ecosystem functioning over Eurasia should be established. Beside timing, occurrence and type of abrupt shift, the analysis of spatial variability of the type / timing of trend shift as well as in the direction of change is of high relevance for ecosystem assessment studies. Accurate assessment of environmental changes and land dynamics calls for long-term, high quality, continuous, harmonized Earth Observation time series (both climate and vegetation datasets). Further important is more research on scale implication (spatial resolution, observation period). At which scale can be a reliable indicator of ecosystem functioning derived? The Mongolia case study discusses this scale problem. Further, the comprehensive attribution of drivers remains a big challenge (co-occurrence of global and local drivers) that cannot be performed without additional data on the drivers and expert knowledge. Both case studies show that land-use change modelling is a highly dynamic field of research with many new developments. The main current developments presented in these special case studies concern progress in
-
The modelling of drivers of land-use change.
-
Modelling of scale dependency of drivers of land-use change.
-
Modelling progress in predicting location versus quantity of land-use change.
-
The incorporation of biophysical feedbacks in land-use change models.
Much of the interest in landscape dynamics is coming from the desire to understand how human activities have influenced landscapes, and how this understanding can inform future land planning and ecosystem stewardship for the drylands of Eurasia. Much of the focus has been on how anthropogenic changes in disturbance regimes that have altered landscape patterns and dynamics. For instance, changes in fire disturbance regimes due to altered climate conditions or fire suppression activities, and the impacts of forest management activities and land use activities, e.g. logging, have received high attention.
Eurasia, as shown in this book, is a unique region from an ecological point of view with a remarkable mix of climatic and edaphic conditions, vegetation types and land use patterns. Over the twentieth century, drastic changes, both natural and anthropogenic are studied, that profoundly affected landscapes and their ecosystem functioning: Successive institutional changes with large impact on the agricultural sector/landscape, global warming and major droughts (e.g. mid/end-1990s, 2010). We have many reports of greening due to global warming, crop intensification or land abandonment. Other reports tell us of browning due to droughts or land abandonment. One important question that arises when dealing with landscape dynamics is whether changes in ecosystem functioning is only a gradual process? With the increased length of EO time series, we have more and more the possibility to differentiate between gradual and abrupt changes (tipping points). Tipping points in time series (e.g. vegetation cover) can be caused by climate extremes (e.g. severe droughts, floods, etc.), land-cover change, climate / human -induced land degradation or combinations of all. Also data artefacts in the time series (e.g. continuity issues related to changes in platforms/sensors/pre-processing/etc.) could cause such breakpoints in time series and are misleading in the final assessment.
Therefore a new ecosystem-state assessment method for Eurasia that reveals turning points in Eurasian ecosystem functioning must and could be developed in the future. Land dynamics research requires new sophisticated datasets: Long-term, high quality/continuous, global time series; trade-off between length of the observation period and spatial resolution; a common and clear selection of the method for trend assessment (OLS, Mann-Kendall, Thiel-Sen, etc.). Here we have to keep in mind that different methods may lead to different results (e.g. look of the different results in the Mongolia case study using different sensors or length of time series). We have also to focus on the attribution of changes to extricate anthropogenic (local) and climate (regional-global) drivers. To achieve a better ecosystem stewardship, we have to combine historical retrospective studies and spatial modeling to derive important insights in landscape dynamics. As a major outlook of this book, the development of a new method for ecosystem state assessment, which combines tipping point analysis, ecosystem response categorization and traditional trend analysis, is necessary for framing new ecosystem stewardship.
References
Adyasuren T, Sugita M, Erdenetuya M (eds) (2005) Assessment of pastureland change using remote sensing data in eastern steppe zone of Mongolia
Allen-Diaz B, Chapins FS, Diaz S et al (1996) Rangelands in a changing climate: impacts, adaptation and mitigation. In Watson et al (eds), Climate change impacts, adaptation and mitigation, pp. 131–158. Cambridge University Press, Cambridge
Asefa DT, Oba G, Weladji RB, Colman JE (2003) An assessment of restoration of biodiversity in degraded high mountain grazing lands in northern Ethiopia. Land Degrad Dev 14:25–38
Bao G, Qin Z, Bao Y et al (2014) NDVI-based long-term vegetation dynamics and its response to climatic change in the Mongolian Plateau. Remote Sens 6(9):8337–8506
Barthel H (1983) Die regionale und jahreszeitliche differenzierung des klimas in der Mongolischen Volksrepublik. Stud Geogr 34:3–91
Berrisford P, Dee D, Poli P et al (2011) ERA report series: 1 The ERA-Interim archive Version 2.0. 09.12.2014. Available online: http://old.ecmwf.int/publications/library/do/references/show?id=90276. Accessed 9.Dec 2014
Berry L, Roderick ML (2002) CO2 and land-use effects on Australian vegetation over the last two centuries. Aust J Bot 50(4):511
Bormann FH, Likens GE (1979) Pattern and process in a forested ecosystem: disturbance, development, and the steady state based on the Hubbard Brook ecosystem study. Springer. ISBN 0387903216, 9780387903217
Chartier MP, Rostagno CM (2006) Soil erosion thresholds and alternative states in northeastern Patagonian rangelands. Rangeland Ecol Manag 59:616–624
Chen J, Ouyang Z, John R et al (2020) Social-ecological systems across the Eurasian drylands. In: Gutman G et al (eds) Landscape dynamics of drylands across greater Central Asia: people, societies and ecosystems. Springer, Cham
Chogni O (1989) Methods for the protection and restoration of pasture. In: Tserendolam R, Tserendash S (eds) Current state of natural pasture use and protection, Proceedings of a scientific conference (in Mongolian). Ulaanbaatar, pp 12–14
Chu T, Guo X (2012) Characterizing vegetation response to climatic variations in Hovsgol, Mongolia using remotely sensed time Series Data. In: Dagvadorj et al. (UNEP) Mongolia Assessment Report on Climate Change 2009. http://www.unep.org/climatechange/adaptation/ScienceandAssessments/MongoliaAssessmentReport/tabid/29575/Default.aspx
Dagvadorj D, Batjargal Z, Natsagdorj L (2014) Mongolia Second Assessment Report on Climate Change 2014 (MARCC-2014) Ministry of Environment and Green Development of Mongolia
Dee DP, Uppala SM, Simmons AJ et al (2011) The ERA-interim reanalysis: configuration and performance of the data assimilation system. Q J Roy Meteor Soc 137(656):553–597
Donohue RJ, Roderick ML, McVicar TR, Farquhar GD (2013) Impact of CO2 fertilization on maximum foliage cover across the globe’s warm, arid environments. Geophys Res Lett 40(12):3031–3035
Dorj O, Enkhbold M, Lkhamyanjin S et al (2013) Mongolia: country features, the main causes of desertification and remediation efforts. In: Heshmati GA, Squires VR (eds) Combating desertification in Asia, Africa and the Middle East. Springer, Dordrecht, pp 217–228
Dulamsuren C, Klinge M, Degener J et al (2016) Carbon pool densities and a first estimate of the total carbon pool in the Mongolian forest-steppe. Glob Chang Biol 22:830–844
Eckert S, Hüsler F, Liniger H, Hodel E (2015) Trend analysis of MODIS NDVI time series for detecting land degradation and regeneration in Mongolia. J Arid Environ 113:16–28
Ehleringer J, Cerling T (2002) C3 and C4 photosynthesis. In: Encyclopedia of global environmental change. The earth system: biological and ecological dimensions of global environmental change, vol 2. Wiley, Chichester, pp 186–190
Fensholt R, Rasmussen K, Nielsen TT, Mbow C (2009) Evaluation of earth observation based long term vegetation trends—intercomparing NDVI time series trend analysis consistency of Sahel from AVHRR GIMMS, Terra MODIS and SPOT VGT data. RSE 113(9):1886–1898
First National Communication of the Republic of Kazakhstan under the United Nations Framework Convention on Climate Change (2013). Almaty, p 74
Henebry GM, Chen J, Gutman G, Kappas M (2020a) Multiple perspectives on Eurasian drylands. In: Gutman G et al (eds) Landscape dynamics of drylands across greater Central Asia: people, societies and ecosystems. Springer, Cham
Henebry GM, de Beurs KM, John R et al (2020b) Recent land surface dynamics across the Eurasian drylands. In: Gutman G et al (eds) Landscape dynamics of drylands across greater Central Asia: people, societies and ecosystems. Springer, Cham
Higgins SI, Scheiter S (2012) Atmospheric CO2 forces abrupt vegetation shifts locally, but not globally. Nature 488(7410):209–212
Hilbig W (1995) The vegetation of Mongolia. SPB Academic Pub, Amsterdam
Huang S, Siegert F (2006) Land cover classification optimized to detect areas at risk of desertification in North China based on SPOT VEGETATION imagery. J Arid Environ 67(2):308–327
Huete AR (1988) A soil-adjusted vegetation index (SAVI). Remote Sens Environ 25:295–309
Iwasaki H (2009) NDVI prediction over Mongolian grassland using GSMaP precipitation data and JRA-25/JCDAS temperature data. J Arid Environ 73(4–5):557–562
Jamsranjav J (2012) Key elements for success and weakness of community based conservation in Mongolia
Javandulam T, Tateishi R, Sanjaa T (2005) Analysis of vegetation indices for monitoring vegetation degradation in semi–arid and arid areas of Mongolia. Int J Environ Stud 62(2):215–225
John R, Chen J, Ou-Yang ZT et al (2013) Vegetation response to extreme climate events on the Mongolian Plateau from 2000 to 2010. Environ Res Lett 8(3)
Kogan F (1990) Remote sensing of weather impacts on vegetation in non-homogeneous areas. Int J Remote Sens 11:1405–1419
Lorenz C, Kunstmann H (2012) The hydrological cycle in three state-of-the-art reanalyses: intercomparison and performance analysis. J Hydrometeorol 13(5):1397–1420
Matthew MJ, Durre I, Korzeniewski B et al (2012) Global historical climatology network – daily (GHCN-daily), version 3. NOAA National Climatic Data Center
Millennium Ecosystem Assessment (2005a) Ecosystems and human Well-being: desertification synthesis. World Resources Institute, Washington, DC
Millennium Ecosystem Assessment (2005b) Ecosystems and human well-being: current state and trends. Island Press, Washington, DC
Morgan JA, Pataki DE, Körner C, Clark H et al (2004) Water relations in grassland and desert ecosystems exposed to elevated atmospheric CO2. Oecologia 140(1):11–25
Myers N, Mittermeier RA, Mittermeier CG et al (2000) Biodiversity hotspots for conservation priorities. Nature 403:853–858
Nandintsetseg B, Shinoda M, Kimura R, Ibaraki Y (2010) Relationship between soil moisture and vegetation activity in the Mongolian steppe. SOLA 6:29–32
Ogar NP, Bragina TM (1999) Transformation of ecosystems and their components: basic terms and concepts. In: Transformation of natural ecosystems and their components during desertification. Almaty, pp 28–32
Park HS, Sohn BJ (2010) Recent trends in changes of vegetation over East Asia coupled with temperature and rainfall variations. J Geophys Res 115(D14)
Peng S, Chen A, Xu L, Cao C (2011) Recent change of vegetation growth trend in China. Environ Res Lett 6(4):44027
Piao S, Mohammat A, Fang J et al (2006) NDVI-based increase in growth of temperate grasslands and its responses to climate changes in China. Global Environ Chang 16(4):340–348
Purevsuren T, Hoshino B, Ganzorig S, Sawamukai M (2012) Spatial and temporal patterns of NDVI response to precipitation and temperature in Mongolian steppe. http://hdl.handle.net/10659/3449
Quetier F, Thebault A, Lavorel S (2007) Plant traits in a state and transition framework as markers of ecosystem response to land-use change. Ecol Monogr 77:33–52
Rosales M, Livinets S (2005) Grazing and land degradation in CIS countries and Mongolia: Food and Agriculture Organization of the United Nations. Proceedings of the electronic conference on grazing and land degradation in CIS countries and Mongolia. Available at: http://www.fao.org/fileadmin/templates/lead/pdf/e-conf_05%E2%80%9306_background.pdf
Rouse JW, Haas RH, Schell JA, Deering DW (1973) Monitoring vegetation systems in the great plains with ERTS. In: Proceedings of the third ERTS symposium (NASA SP-351), pp 309–317
Samanta A, Costa MH, Nunes EL et al (2011) Comment on drought-induced reduction in global terrestrial net primary production from 2000 through 2009. Science 333(6046):1093
Sankaran M, Anderson M (2009) Management and restoration in African savannas: interactions and feedbacks. In: Hobbs, Suding (eds) New models for ecosystem dynamics and restoration. Island Press, London, pp 136–155
Scripps (2016) Homepage of the Scripps CO2 program. Available at: http://scrippsco2.ucsd.edu
Spivak L, Terekhov A, Vitkovskaya I, Batyrbayeva M (2009) Use of long-term satellite data of different resolution for a complex assessment of the vegetation cover of the territory of Kazakhstan. Modern problems of remote sensing of earth from space. Moscow IKI RAS 6(2):450–458
Spivak L, Vitkovskaya I, Batyrbaeva M, Terekhov A (2010) Detection of desertification zones using multi-year remote sensing data. In: NATO science for peace and security, series C: environmental security use of satellite and in-situ data to improve sustainability. Springer, pp 235–241
Spivak L, Vitkovskaya I, Batyrbayeva M, Terekhov A (2012) The experience of land cover change detection by satellite data. Front Earth Sci Higher Education Press/Springer, Berlin/Heidelberg 6(2):140–146
Spivak L, Batyrbaeva M, Vitkovskaya I (2017) The system of space monitoring of vegetation and drought conditions in Kazakhstan based on long-term series of remote sensing data. In: Ground-space monitoring of dynamics of geospheres. Almaty pp. 174–189
Standish RJ, Cramer VA, Yates CJ (2009) A revised State-and-Transition model for the restoration of woodlands in Western Australia. In: Hobbs, Suding (eds) New models for ecosystem dynamics and restoration. Island Press, London, pp 169–188
Sun Z, Wang Q, Xiao Q et al (2015) Diverse responses of remotely sensed grassland phenology to interannual climate variability over frozen ground regions in Mongolia. Remote Sens-Basel 7(1):360–377
Suttie JM, Reynolds SG (eds) (2003) Transhumant grazing Systems in Temperate Asia. FAO Plant Production and Protection Series No 31
Tanaka T, Jayakumar R, Erdenechimeg B (2008) Proceedings of the UNESCO chair workshop on sustainable groundwater management in arid and semi-arid regions: IHP VII | Technical Document in Hydrology | No. 1
Taube F, Herrmann A (2009) Relative benefit of maize and grass under conditions of climatic change. In: Schwarz (ed) Optimierung des Futterwertes von Mais und Maisprodukten, vol 331 of Landbauforschung/Sonderheft, vol VTI, Braunschweig, pp 115–126
Tietjen B, Jeltsch F (2007) Semi-arid grazing systems and climate change: a survey of present modelling potential and future needs. J Appl Ecol 44:425–434
Tserendash S, Erdenebaatar B (1993) Performance and management of natural pasture in Mongolia. Nomad People 33:9–15
Turner MG, Romme WH, Gardner RH et al (1993) A revised concept of landscape equilibrium: disturbance and stability on scaled landscapes. Landsc Ecol 8(3):213–227
Ulziikhutag N (1989) Survey of Mongolian vegetation provinces (in Mongolian). Ulsiin Khevleliin Gazar, Ulaanbaatar
UNCBD (2014) Mongolia’s fifth national repo on implementation of convention of biological diversity. Available at: https://www.cbd.int/doc/sworld/mn/mn-nr-05-en.pdf
UNCCD (2012) United Nations Convention to Combat Desertification. Available at: www.unccd.int
Vostokova EA, Gunin PD (2005) Ecosystems of Mongolia. Atlas. Russian Academy of Sciences, Moscow
Wang C, Wang S, Zhou H, Glindemann T (2007) Effects of forage composition and growing season on methane emission from sheep in the Inner Mongolia steppe of China. Ecol Res 22(1):1011–1021
Watt AS (1947) Pattern and process in the plant community. J Ecol 35(1/2):1–22.
Wen X, Lu H, Li C et al (eds) (2014) SPIE Asia Pacific remote sensing, SPIE proceedings, SPIE
Yu F, Price KP, Ellis J, Shi P (2003) Response of seasonal vegetation development to climatic variations in eastern Central Asia. Remote Sens Environ 87(1):42–54
Yu F, Price KP, Ellis J, Kastens D (2004) Satellite observations of the seasonal vegetation growth in Central Asia: 1982–1990. Photogramm Eng Remote Sens 70(4):461–469
Yunatov AA (1977) Fundamental characteristics of the vegetation of the Mongolian People’s Republic (in Mongolian). Ulsiin Khevleliin Gazar, Ulaanbaatar
Zhang X, Hu Y, Zhuang D et al (2009) NDVI spatial pattern and its differentiation on the Mongolian Plateau. J Geogr Sci 19(4):403–415
Zhao M, Running SW (2010) Drought-induced reduction in global terrestrial net primary production from 2000 through 2009. Science 329(5994):940–943
Zhu Z, Piao S, Myneni RB et al (2016) Greening of the Earth and its drivers. Nat Clim Chang 6:791–795.
Acknowledgments
We would like to thank the German Academic Exchange Service (DAAD) for funding the International German Alumni Summer School Mongolia 2015 “Large scale natural landscapes in Mongolia under the pressure of climate change and competition for land and resources’’. This summer school enabled research on vegetation dynamics over Mongolia.
Author information
Authors and Affiliations
Corresponding author
Editor information
Editors and Affiliations
Rights and permissions
Copyright information
© 2020 Springer Nature Switzerland AG
About this chapter
Cite this chapter
Kappas, M., Degener, J., Klinge, M., Vitkovskaya, I., Batyrbayeva, M. (2020). A Conceptual Framework for Ecosystem Stewardship Based on Landscape Dynamics: Case Studies from Kazakhstan and Mongolia. In: Gutman, G., Chen, J., Henebry, G., Kappas, M. (eds) Landscape Dynamics of Drylands across Greater Central Asia: People, Societies and Ecosystems. Landscape Series, vol 17. Springer, Cham. https://doi.org/10.1007/978-3-030-30742-4_9
Download citation
DOI: https://doi.org/10.1007/978-3-030-30742-4_9
Published:
Publisher Name: Springer, Cham
Print ISBN: 978-3-030-30741-7
Online ISBN: 978-3-030-30742-4
eBook Packages: Biomedical and Life SciencesBiomedical and Life Sciences (R0)