Abstract
The long-run effects of disasters on economic growth have been studied since the pioneering work of Dacy and Kunreuther (The economics of natural disasters: implications for federal policy. The Free Press, New York, 1969). The recent empirical studies on this subject presented mixed results about whether or not disasters affect economic growth. Some studies that employed socio-economic indicators for disaster intensity, such as the number of casualties and/or the value of economic damages, to analyze the effects on growth found inconsistent or inconclusive results among them. Some more recent studies that utilized physical intensity indices, such as the Richter scale for earthquakes and the maximum wind speed for storms, revealed statistically significant negative effects on economic growth. In order to improve our understanding of disaster’s effects on economic growth and to evaluate these empirical results, this chapter examines a set of theoretical growth models from both the neoclassical perspective and the Keynesian perspective. The insights gained from the analysis include: the speed of recovery depends on the changes in saving rate, which can be raised through more patient preference toward future (lower rate of time preference and higher intertemporal elasticity of substitution); and cumulative changes (either growth or decline) of a damaged region can be caused by the changes in economic structure through either elasticities of demand for imports or of demand for exports from the damaged region. The latter result supports the findings in the recent empirical studies that evaluated the structural changes caused by a disaster and the subsequent reconstruction process.
This chapter is a significantly extended and revised version of Okuyama (2003).
Access provided by Autonomous University of Puebla. Download chapter PDF
Similar content being viewed by others
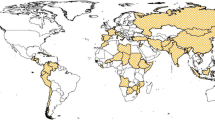
1 Introduction
The book, The Economics of Natural Disasters, by Douglas C. Dacy and Howard Kunreuther was published in 1969, following the 1968 National Flood Insurance Act of the United States and devastating losses from the 1964 Alaska earthquake. Chapter 3 of the book is titled “Economic Theory and Natural Disaster Behavior,” and discusses the theoretical analysis of behavior under natural disasters with the following two sub-sections: (1) a short-run recuperation phase and (2) long-run recovery problems. In their analysis, the short-run recuperation phase is dealt with through microeconomic theory, such as decision-making theory and laws of demand and supply, whereas the long-run recovery problems are investigated using macroeconomic theory, such as economic growth theory. In particular, they defined the long-run recovery as “the rebuilding process that brings the community back to its pre-disaster economic level” (page 70).
In order to analyze the long-run recovery problems, Dacy and Kunreuther employed a simplified version of the Solow-Swan growth model (Solow 1956; Swan 1956). They divide capital stock, K, into three-fold in terms of their use: public capital, Kp, business capital, Kb, and residential capital, Kr. Then, the production function of an economy becomes as follows:
After a disaster, capital stock is reduced to \( {K}_p^{\ast} \), \( {K}_b^{\ast} \), and \( {K}_r^{\ast} \) for each type of capital stock. For simplicity, it is assumed that the levels of labor and outside aid for capital recovery are fixed at \( \overline{L} \) and \( \overline{K} \), respectively. During the recovery from disaster damages, the production function, Eq. (4.1), is transformed to the following form:
The labor and outside aid, \( \overline{L} \) and \( \overline{K} \), need to be allocated to recover damaged capital in order to maximize the total output, Y.
With this formulation, it is possible to investigate the resource allocation of aid across the different types of capital stock so that, as they claimed, the optimum path of recovery can be analyzed. Unfortunately, they did not elaborate the model any further theoretically or analytically. It may have become more useful if their model specified the relationships of productivity between the different types of capital. For example, public capital, Kp, such as infrastructure and lifelines, can have a meaningful improvement to the productivity of undamaged business capital, Kb, and to the recovery process (accumulation process after a disaster) of both damaged business capital and residential capital, Kb and Kr.
To complement and extend Dacy and Kunreuther’s pioneering work in this regard, this chapter examines the long-run effects of a disaster, namely the effects on economic growth, using two distinctive perspectives with their theoretical models: one is the neoclassical growth model from the supply side, as Dacy and Kunreuther did; and the other is the Keynesian growth model from the demand side. These theoretical models can provide insights about how a disaster affects the economic growth of a nation or a region, focusing on the transitional dynamics of the recovery and reconstruction process. In Sect. 4.2, the empirical studies on long-run effects of disasters are reviewed and discussed in order to pave the way to the theoretical models through highlighting whether or not disasters affect the trajectory of economic growth empirically. Section 4.3 presents the analysis from the neoclassical perspective, utilizing various models, from Solow-Swan’s to the open economy models. Section 4.4 turns to the Keynesian perspective and discusses the Kaldor-Dixon-Thirlwall (KDT) model and its use in disaster analysis. Finally, Sect. 4.5 concludes this chapter with some discussions on policy implications, making connection with the empirical studies discussed in Sect. 4.2, and presents a few potential extensions.
2 Macroeconomic Analysis of Disaster Impacts
The long-run effects of disasters on economic growth have been investigated empirically, using cross-country macroeconomic statistics and disaster data, which are oftentimes extracted from the EM-DAT database. While most studies employ some form of econometric models with various techniques to test the relationship between economic growth (usually the growth rate of per capita GDP) and disaster impact (number of casualties, damages in monetary value, etc.), their conclusions do not agree with each other. For example, Albala-Bertrand (1993a) used Latin American disaster cases and found that capital damages are unlikely to cause significant effects on growth. This conclusion is echoed by Cavallo et al. (2013) that even extremely large disasters do not display any significant effect on economic growth when political changes of the country are controlled. On the other hand, various other studies concluded that some types of disasters cause negative or positive impacts on economic growth of a national economy. For instance, the findings in Noy (2009) indicate that disasters have a statistically observable impact on economic growth when they are measured by the amount of property damage incurred. Some other studies estimated the effects from different disaster types and found some mixed results. Skidmore and Toya (2002) showed no effect by geophysical hazards but a positive effect from climatic hazards, while Fomby et al. (2013) found positive effect of floods, negative effect of storms and droughts, and mixed results from earthquakes.
One of the reasons that these studies provide conflicting results is that disaster impact data, such as the number of casualties and damages in monetary value, as an independent variable creates an endogeneity problem,Footnote 1 in which such an independent variable may correlate with the error term in regression models, violating the basic assumption of regression analysis. Moreover, socio-economic data on disaster impacts, such as economic damage, are typically much harder to find, let alone the data being consistent, as Skidmore and Toya (2002) claimed that “disaster variables are somewhat crude measures.” In order to overcome this problem, Hsiang and Jina (2014) employed the physical intensity index, i.e., wind speed exposure and energy dissipation, for analyzing the effects of cyclones on economic growth. They found robust evidence that national incomes decline relative to their pre-disaster trend and do not recover within 20 years to the pre-disaster level, and that income losses arise from a small but tenacious suppression of annual growth rates over the 15 years following a disaster, generating significant cumulative negative effects. Strobl (2012) also studied the effect from hurricanes in the Central American and Caribbean regions using a physical intensity index, called the hurricane destruction index, and found that the average hurricane strike caused GDP growth rate to fall by around 0.84 percentage points. Using the rainfall and GDP data of 153 countries during the period of 1960–2002, Berlemann and Wendzel (2016) examined the economic effects of drought, and their results show significantly negative long-run growth effects of droughts in both developed and developing countries. Furthermore, Felbermayr and Gröschl (2014) covered a few types of natural hazards and used corresponding physical indicators as disaster intensity. For example, they used the disaster intensity index of the Richter scale value for earthquakes, the maximum wind speed for storms, the maximum difference in monthly precipitation for drought or flooding events, and so forth. Their analysis reveals that disasters lower GDP per capita temporarily. It is interesting to observe that all the studies using physical intensity indicators of disaster intensity found negative effects of disaster on economic growth, whereas the studies using socio-economic disaster indicators provide somewhat mixed results. In fact, these results with physical intensity indicators vindicate the use of a simple neoclassical growth model, such as the above Dacy and Kunreuther’s, for the analysis of long-run effects of disasters, since the neoclassical growth model predicts the lower per capita output when a disaster destroys a part of capital accumulation.Footnote 2
While the above empirical studies investigate the long-run effects of a disaster based on cross-country data, there are a series of case studies that trace the effects of a particular disaster over time to examine the long-run effects on a regional economy, including Odell and Weidenmier (2002), Baade et al. (2007), Hornbeck (2009), and Coffman and Noy (2011). In particular, the 1995 Kobe earthquake in Japan has been receiving more attention, partly because of the data availability and the well-documented reconstruction process. For instance, Chang (2010) employed simple indicators to measure the recovery process of the City of Kobe after the earthquake, and the results illustrate a 3–4-year temporary gain of production from the reconstruction demand injection, followed by a decline of around 10% below pre-disaster levels. DuPont and Noy (2015) analyzed the long-run economic trend of the Hyogo Prefecture, instead of the City of Kobe, after the 1995 earthquake, using econometric models with the synthetic control methodology to examine the earthquake’s effect on Gross Regional Products (GRP) and local government expenditures. Their results show a persistent and continuing adverse impact of the event after 15 years. They also obtained the similar level of long-run decline to Chang’s study, where GRP per capita in 2007 was 13% less than the projected economic trend without the earthquake. In contrast, Okuyama (2016) employed the GRP per capita of the City of Kobe with a general form of linear autoregressive-distributed lag model to examine the long-run trend of the Kobe economy before and after the event. The results are consistent with the aforementioned two studies, displaying a steady decline of Kobe’s GRP per capita following the reconstruction boost of only 3 years. On the other hand, Fujiki and Hsiao (2013) did not find such persistent declining trends in the Hyogo Prefecture, utilizing econometric models based on macroeconomic data between 1955 and 2009. Their results indicate that the stimulation effects from the recovery and reconstruction activities occurred from 1995 to 1998, while smaller negative impacts from the end of the intense demand injections were found between 1999 and 2000. They concluded that the long-run decline of the Hyogo Prefecture resulted from the underlying structural change of the economy rather than from the earthquake and its related activities.
In order to investigate the structural changes caused by the 1995 Kobe Earthquake in a more detailed manner, Okuyama (2014, 2015) performed structural analyses of the Kobe economy before and after the Kobe Earthquake based on a time series of the Kobe regional input-output tables. Changes in gross output of the Kobe economy are decomposed into different factors, such as changes in final demand, in the technological coefficient matrix, and in the regional purchase coefficient matrix, while the changes specific to Kobe are set apart from the macroeconomic disturbances through the shift-share analysis. The results pointed out the significant structural changes that occurred in the Kobe economy after the event, and the most influential factor for such changes appears to be the decline of regional final demand, while the changes in regional interindustry relationships also acted as a crucial role but to a smaller degree.
While the above empirical studies shed light on how an economy is affected by and responds to a disaster, theoretical considerations based on various growth models can provide some insights that can explain these empirical observations and lead to policy implications for mitigating negative effects. The following sections deal with two distinctive perspectives for theoretical investigation: the neoclassical perspective and the Keynesian perspective.
3 Investigation from the Neoclassical Perspective
Catastrophic disasters can create significant and intense damages to capital stocks, and sometimes to labor. These damages become quite serious in the context of sub-national regions and of developing countries (Albala-Bertrand 1993b). In this section, the long-run effects of a disaster are investigated based on neoclassical growth models.
3.1 The Solow-Swan Model
The basic but popular neoclassical Solow-Swan growth model (Solow 1956; Swan 1956) is examined first to see how disasters affect the growth path of an economy. Consider, for a moment, if technological progress can be neglected,Footnote 3 the production function of an economy can be set as:
where Y is the total output, K is the level of capital accumulation, and L is the level of labor population. The use of per capita terms for output and capital makes Eq. (4.3) the intensive form:
where \( y=\raisebox{1ex}{$Y$}\!\left/ \!\raisebox{-1ex}{$L$}\right. \), and \( k=\raisebox{1ex}{$K$}\!\left/ \!\raisebox{-1ex}{$L$}\right. \). Suppose that the constant saving rate is s, the constant capital depreciation rate is δ, and the constant population growth rate is n. The changes in per capita capital stock over time become as follows:
where \( \dot{k}=\raisebox{1ex}{$ dk$}\!\left/ \!\raisebox{-1ex}{$ dt$}\right. \). Thus, the steady-state level of capital accumulation, k∗, where \( \dot{k}=0 \), satisfies the following condition:
This steady-state condition can be seen as the point A in Fig. 4.1. Now, assume that an economy is at the steady stateFootnote 4 and that a catastrophic natural hazard, such as a large earthquake, occurred and the capital stocks were severely damaged, but with no or minimal casualties to the labor population. The damages from the natural hazard can be reflected as a temporal increase in the depreciation rate, from δ to δd, where δd is the increased depreciation rate accounting for the damages, seen as the move from (n + δ)k to the broken line of (n + δd)k. Consequently, the per capita capital level goes down to the decreased level, kd, where kd < k∗. However, this increase in the depreciation rate is instantaneous, just reflecting the damages on capital stock, thus it momentarily moves back to the pre-disaster depreciation rate of δ. The economy’s production level decreases due to the damages from the steady-state level, y∗, to the damaged level, yd. Because of the lower level of per capita capital, the economy is now out of its steady state, and returning to the steady-state production level requires an increase in capital accumulation, from kd to k∗.
The transitional dynamics of recovery can be further illustrated by the use of the growth rate of k. The growth rate of per capita capital, γk, can be given based on Eq. (4.5):
Figure 4.2 illustrates the transitional dynamics around the steady state. At the steady state, the growth rate becomes zero, thus s ⋅ f(k)/k = (n + δ). Due to the disaster damages, the level of per capita capital becomes kd, and because of this deviation from the steady state, the growth rate of per capita capital becomes positive (the distance between B and C in Fig. 4.2). Thus, the economy starts re-accumulating capital stock toward the previous steady-state level. The speed of re-accumulation is determined by Eq. (4.7). If the economy desires to return to the previous level of per capita income as soon as possible, the respective government may introduce some temporary measures to finance reconstruction activities for a faster recovery. In this modeling framework, savings are equal to investment as shown in Eq. (4.5), thus the reconstruction activities as investment can be established as a temporary increase of the saving rate for accelerating the growth rate, as the saving rate becomes sr(where sr > s) in Figs. 4.1 and 4.2. Consequently, the growth rate of per capita capital becomes much higher (the distance between D and C, which is much wider than between B and C with the original saving rate in Fig. 4.2), thus the recovery of per capita income becomes faster. As the reconstruction progresses, the government makes the saving rate gradually return to the previous level, s, and the economy returns to the original steady state (from D to A in Figs. 4.1 and 4.2). It can be concluded that the more resources are allocated to the recovery and reconstruction, the faster the speed of recovery (capital re-accumulation) becomes. At the same time, since a sizable increase in the saving rate leads to a severe decrease in current consumption, this will become a difficult option for policy makers. As Healy and Malhotra (2009) suggested, ex-ante mitigation strategies and/or disaster insurance against future disaster damages require much less cost than the ex-post reconstruction projects. Therefore, in order to allocate resources effectively and efficiently, the saving rate will be a bit higher for including such mitigation investment to countermeasures, and it leads to a slightly lower per capita production level over the years.
3.2 Technology Update in the Solow-Swan Model
As discussed in Okuyama (2003), the damages of a catastrophic disaster tend to be found more often at older and outdated facilities and equipment than newer and retrofitted ones, mainly because of having weaker structure and being fitted to outdated regulations (for instance, building codes) that are applied for older capital stocks. Through the recovery activities, these damaged older facilities and equipment are replaced with updated and/or upgraded newer facilities and equipment with more advanced technologies. Thus, this technology update during the recovery and reconstruction period has some potential to influence the speed of recovery and/or the growth path. In order to examine the effect from the technology update during reconstruction, we need first to introduce variable technology in the above Solow-Swan model of Eq. (4.3). Suppose the level of labor-augmented technology, At, and the progress of the technology is determined by At = ext, where x is the rate of technological progress. The production function of the above Eq. (4.3) becomes:
The changes in per capita capital over time is written as:
where \( \hat{k}=\raisebox{1ex}{$K$}\!\left/ \!\raisebox{-1ex}{$ AL$}\right. \). As long as x is the constant value, the results of the above analysis also hold with this specification.
Hallegatte and Dumas (2009) examined the effect of these technological updates on growth based on their extended Solow-Swan growth model. Their model, called the Non-Equilibrium Dynamic Model (NEDyM), not only reproduces the behavior of the Solow-Swan model over the long-run but also allows disequilibria during transition phases, which is a suitable feature under a disaster and during a recovery situation. Whereas the detailed descriptions of the NEDyM can be found in Hallegatte et al. (2007), Hallegatte and Dumas (2009) extend the NEDyM to evaluate the effects of technological updates in a neoclassical framework.Footnote 5 They set the latest technology level in an economy at time t as At, while the installed capital for production has a mixture of current and various levels of old technology. Thus, the technology level of the installed capital at time t can be measured as a mean technology level, Λt, which is Λt < At. While newly installed capital has the latest technology, the mean technology level can be determined as the weighted average of technology levels as follows:
Based on this, the growth of technology level becomes:
If a natural hazard damages a part of the existing capital stocks (higher depreciation rate in (4.10) at the time of the natural hazard) and the economy tries to reconstruct them with the latest technology, the above mean technology level becomes higher than the case without such a disaster. In this way, the technology updates can be dealt more realistically than just raising the overall technology level of an economy. Based on a series of simulations using NEDyM, it is concluded that because the growth rate is only determined by the rate of technological progress, not by the average level of technology that does not influence the rate of technological progress, disasters can only boost production levels through updates of damaged capital but cannot lead to the overall technological progress, thus cannot increase the long-run growth rate. Empirical studies on this issue found conflicting results, however. Based on the cross-country analysis, Skidmore and Toya (2002) concluded that climatic disasters are positively correlated with economic growth, investment in human capital, and total factor productivity growth through technology updates, whereas geophysical disasters are negatively correlated with economic growth. Meanwhile, Cuaresma et al. (2008) employed the cross-country and panel data among developing countries for examining the relationship between technological transfer and disaster risk. Their results show that disaster risk is negatively correlated with the extent of technological transfer, while only countries with higher levels of per capita income can benefit from technological transfer after a disaster. Their analysis indicates that the reconstruction period after disasters are not considered as a good trigger to install newer technologies in developing countries, whereas it cannot be compared directly with the Skidmore and Toya’s. Further empirical studies and theoretical development are needed to evaluate this issue.
3.3 Determining the Behavior of the Saving Rate: Ramsey Model
The above analyses guide toward a policy implication that the speed of recovery depends on the resource allocation for recovery activities, i.e. changes in saving rate. However, an increase in saving rate implies a decline in current consumption level, while the changes in consumption level are determined by the consumers’ preference. In this context, the analysis should extend to employ the Ramsey model with consumer optimization (Ramsey 1928; Cass 1965; Koopmans 1965). In this way, the optimum allocation of resources for recovery and reconstruction can be explored.
In the Ramsey model, each household wishes to maximize overall utility, U, as follows:
where c(t) is per capita consumption at t, ρ is the rate of time preference and ρ > 0. The utility function, u(c), is assumed to have the following form:
where −θ is the constant elasticity of marginal utility and θ > 0. The households’ utility maximization problem of (4.12), subject to (4.9), will derive the optimal path of consumption as:
Equation (4.14) and the steady-state consumption growth, \( \dot{\hat{c}}=0 \), imply:
where \( {\hat{k}}^{\ast} \) is the steady-state level of capital per effective labor. In order to analyze the transitional behavior of the saving rate, the production function is assumed to be a Cobb-Douglas form as follows:
At the steady state, \( \dot{\hat{k}}=f\left(\hat{k}\right)-\hat{c}-\left(x+n+\delta \right)\hat{k} \) and \( \dot{\hat{c}}/\hat{c} \) from Eq. (4.14) above are each equal to zero. These and \( f\left(\hat{k}\right)/\hat{k}={f}^{\prime}\left(\hat{k}\right)/\alpha \) from (4.16) yield the steady-state saving rate as:
The transversality condition of this system implies that the steady-state rate of return, \( {f}^{\prime}\left({\hat{k}}^{\ast}\right)-\delta \), exceeds the steady-state growth rate, x + n (Barro and Sala-i-Martin 2004). This condition can be rewritten based on Eq. (4.15):
This can be transformed to ρ + θx > x + n, and with Eq. (4.17), it leads to s∗ < α. This indicates that the steady-state gross saving rate is smaller than the gross capital share.
Based on the above Ramsey model, especially with (4.17), the behavior of the saving rateFootnote 6 under the recovery and reconstruction period after a disaster can be examined. Since α, x, n, and ρ are assumed not to be affected by a disaster, the changes in other parameters in (4.17) are considered. First, the depreciation rate, δ, momentarily increases to indicate the damages on capital stock, leading to a lower level of capital accumulation, as above. However, it will return to the original level, and the long-run depreciation rate remains the same as before. Second, the reciprocal of θ is determined as the intertemporal elasticity of substitution, σ = 1/θ. Under a disaster situation, current consumption is reduced for a faster growth in order to accelerate the recovery and reconstruction activities to return to the steady-state capital and income levels, and the intertemporal preference becomes more toward the increase in future consumptions during the recovery period, leading to a higher σ. This can be realized as a higher saving rate. The increase in saving rate during the recovery and reconstruction period is consistent with the above discussion using the Solow-Swan model, in which the saving rate is determined exogenously.
Calzadilla et al. (2007) carried out a simulation analysis, adapting a standard Ramsey model to examine the impact of an extreme event’s damages on a regional economy. The basic structure of the model is similar to the one described above, and the parameter values are set at the ones consistent with the GTAP 5 data set for the U.S. economy in 1997. The simulation is completed with terminal conditions for the final simulation year to make the results consistent with their theoretical model with an infinite horizon. The simulation period was set from 1997 to 2050, and it is assumed that some disaster will occur during the simulation period, but the specific timing of its occurrence is not determined. Since the economy faces a positive probability of the event occurrence each year, the economy prepares for the unexpected happening of such event through a slightly higher saving rate. An unexpected disaster is assumed to occur in 2030. Their simulation results illustrate that at 2030, a part of capital stock is destroyed, and the levels of production and consumption fall. Moreover, the level of consumption further falls due to the higher return from capital that now becomes scarce. This also implies a higher interest rate, leading to a higher saving rate. In their simulation, after 2030, the accumulations of capital and consumption become steeper with this new saving plan, reaching higher levels than the ones without such an event.
The conclusion of Calzadilla et al. (2007) about a higher saving rate and faster growth (recovery) after the event is consistent with the analysis in this section, but it is not coherent with the empirical evidence from the recent studies on the 1995 Kobe earthquake, as discussed in the previous section. One of the reasons for such inconsistency can be that the models presented in this section are closed models, whereas economies are open in terms of capital and labor, especially in the regional context. The next sub-section discusses such open models.
3.4 Interregional Considerations: Open Economy Models
In the age of globalized economic activities, industrial clustering, and vertical specialization of production, economies have become more open and interdependent on each other than before. While disaster impacts are mostly localized (Albala-Bertrand 2007), recovery and reconstruction activities after a disaster rely more on interregional trade, such as importing capital and labor from other regions (sometimes from other countries in the case of small island nations). Therefore, analyzing the growth path of a regional economy after a disaster necessitates the use of open economy models in order to take into account its relationships (constraints) with trade, capital flows, and migration with other regions.Footnote 7
In the neoclassical perspective, regional growth models are assumed to have the features that the economy is perfectly competitive, that the production factors are paid according to their marginal products, and that the production factors are perfectly mobile across regions (Harris 2008; McCann 2013). As in the Solow-Swan model for a closed economy, it is assumed that capital and labor are complementary inputs for production, and their relative quantities are defined as a capital-labor ratio, K/L. Suppose that two regions comprise a nation, and the relationship of their capital-labor ratios between two regions are as follows:
Because the marginal product of capital in region 1 is lower than in region 2, capital in region 1 will move to region 2 to seek higher marginal profits from capital. Meanwhile, because the marginal product of labor in region 2 is lower than in region 1, labor in region 2 will migrate to region 1 for higher wages. In the long-run, these factor migrations will continue until the capital-labor ratios in both regions reach the following equilibriumFootnote 8:
If a catastrophic natural hazard destroyed the capital in region 1, the capital-labor ratio of region 1 becomes smaller than in region 2. Hence, while labor in region 1 will migrate to region 2, capital in region 2 tends to move to region 1, a part of which can be considered as reconstruction of capital in region 1. Overall production level of this nation is determined by the level of total factors, K = K1 + K2 and L = L1 + L2. Since the amount of labor is assumed not to change by the disaster, the total production level after a disaster can be decreased in the short-run due to the damaged capital in region 1. While the production factors are reallocated to reach a new equilibrium in the long-run, there will be further capital accumulation in this nation as in the Solow-Swan model, and the economy will return to the original growth path under this framework. Empirical evidences discussed in Sect. 4.2, such as DuPont and Noy (2015) and Okuyama (2016), found contradictory evidence that the regions with a catastrophic disaster appear not to return to the previous growth path, but rather move toward a different growth path. This contradiction can be attributed to the assumptions of the neoclassical perspective, such as perfect competition, perfect mobility of factors, and marginal returns, and/or to the lack of spatial and internal externalities (McCann 2013).
The open-economy version of the Ramsey model can be formulated to analyze how factor mobility interacts with the saving rate. As Barro and Sala-i-Martin (2004) presented, however, an open-economy Ramsey model, with the assumption of perfect capital mobility and immobile labor, exhibits some paradoxical conclusions, such as per effective labor capital, income, and wages converge instantaneously to their steady-state level (i.e. the speed of convergence is infinite), per effective labor consumption tends to be zero for all but the most patient country (with small θ), and the per effective labor asset becomes negative. There are various modifications to avoid these counterfactual results, whereas they become rather too complicated for applying to disaster cases. Nijkamp and Poot (1998) criticized the open-economy Ramsey model because, in general, the assumption of perfect capital mobility is not realistic, and suggest to consider labor mobility instead. However, in the disaster context, the research question is more about the effect of changes in the level of capital accumulation, decreases by destruction, and increases through reconstruction, rather than changes in the labor population.Footnote 9
4 Analysis from the Keynesian Perspective
The studies on the long-run effects of disasters have been dominated using the neoclassical growth models, as in Dacy and Kunreuther (1969). This is mainly because natural hazards bring destruction on both physical and human capitals. Thus, the production-side analysis based on the neoclassical growth models is straightforward to capture the effects of such shocks. Meanwhile, the demand-side analysis on the long-run effects of disasters based on the Keynesian growth models has been quite limited, due mostly to the ambiguity of demand-side changes in a disaster situation.Footnote 10 Nevertheless, the demand-side analysis is valuable, since the recovery and reconstruction process after a disaster can be considered as an intense demand injection, and because the impact from the disaster can create demand-side effects, such as an adverse effect on demand based on rumors, decreased consumption due to self-restraint, decreased demand from lower incomes, and so forth. Furthermore, in the regional context, the Keynesian models can deal with interregional trade relatively well, so that the relationship with other regions can be investigated. The discussion in this section focuses on the Keynesian growth models.
In order to analyze the regional growth process from the demand side, the Kaldor-Dixon-Thirlwall (KDT) model was proposed (Dixon and Thirlwall 1975; Thirlwall 1980; McCombie and Thirlwall 1994). Suppose that a general long-run regional import demand function is as follows:
where Mr is the level of regional imports to region r, Yr is the regional income level, π is the regional income elasticity of demand for imports, Pf is the nominal price of goods produced in other regions, Pr is the nominal price of goods produced in region r, and μ is the price elasticity of demand for imports. Similarly, set a general long-run regional export demand function as:
where Xr is the level of regional exports from region r, Z is the sum of other regions’ income, ε is other regions’ income elasticity of demand for exports of region r, and η is the price elasticity of demand for the exports from region r by the other regions. These demand functions denote that the levels of imports and exports depend on the price and income elasticities of the goods, and on the relative prices of regional and externally produced goods.Footnote 11 Based on (4.21) and (4.22), the growth rates of import and export become:
and
In the long-run, a region cannot sustain a balance of payments deficit. Consequently, the level of long-run regional import growth depends on the region’s growth in exports, and the relative changes in regional and external production costs and prices. This implies:
Plugging (4.23) and (4.24) into (4.25) yields:
It is assumed that the relative price effects, \( \left[{\dot{P}}_r-{\dot{P}}_f\right] \), are relatively unimportant and can be set to null, when the balance-of-payments model like this is applied to domestic regions (McCann 2013).Footnote 12 Thus, Eq. (4.26) becomes:
This represents that the balance-of-payments constrained long-run growth rate of a region is equal to the long-run rate of the other regions’ income multiplied by the ratio of the other regions’ income elasticity of demand for exports from region r over the regional income elasticity of demand for imports. This turns out to be the long-run rate of growth of exports from region r divided by the regional income elasticity of demand for imports. In this formulation, when a disaster occurs and during the reconstruction period, the region becomes more reliant on imports for reconstruction and the production of goods, and also faces declines in exports due to the damaged production capacity within the region. The increase in imports can be expressed as an increase in the regional income elasticity of demand for imports, π. Meanwhile, the decrease in exports leads to a lower or negative growth rate of exports, \( {\dot{X}}_r \). Both of these changes direct to a smaller or negative growth rate of regional income, \( {\dot{Y}}_r \).
Another component in the Keynesian growth model concerns the issue of economies of scale. As in Dixon and Thirlwall (1975), the analysis of economies of scale centers on the Verdoorn’s Law, in which a positive relationship between the growth rate of labor productivity and the growth rate of output (income) is assumed as follows:
where \( \dot{\rho} \) represents the growth rate of labor productivity, a and b are constants, and b is called the Verdoorn coefficient. If Verdoorn’s Law on dynamic economies of scale is included in the above Keynesian growth model, various regional growth trajectories can be traced through the diagrammatic approach of Dixon and Thirlwall (1975).Footnote 13
Figure 4.3 exhibits a steady-state regional growth based on the Keynesian growth model. In the upper right-hand quadrant, given the export growth of x, and the income elasticity of regional demand for imports, π, the balance-of-payments constrained output growth rate is q. Through the Verdoorn effect, the output growth rate of q results in the regional labor productivity of h, in the upper left-hand quadrant. This leads to quality-adjusted real-price reductions at a rate of s. For the given relative output price, the productivity growth h leads to real quality improvements, which in turn result in regional export growth of x, the actual extent of which will depend on the income elasticity of demand for exports, ε (McCann 2013). In Fig. 4.3, the relationship between \( \dot{X} \) and \( \dot{Y} \) is at a steady state. It can be seen from this figure that different regions can have different growth rates, depending on the ratio of the income elasticities of demand for exports and for imports, as seen in Eq. (4.27).
In order to analyze a disaster situation using this Keynesian growth model, the damages and changes caused by a disaster need to be translated to the parameter values in the model. Since the Keynesian growth model is a demand-side model, no production-side changes, such as increase in the depreciation rate and/or in the saving rate as in the neoclassical models, can be accommodated in the model. Due to a reduced production capacity by the damages and the increased imports for recovery and reconstruction activities, the damaged region can become more dependent on imports, resulting in an increase in the income elasticity of demand for imports. Figure 4.4 illustrates the consequences of such changes. The regional income elasticity of demand for imports becomes πd, where πd > π, and this makes the downward shift of the line in the upper right-quadrant, yielding a lower output growth rate of qd < q. While the Verdoorn coefficients, a and b, do not change by the disaster, the smaller output growth rate causes a lower growth rate of labor productivity in the region hd. Subsequently, the growth rate of exports turns into xd, which is smaller than the steady-state export growth rate of x. This leads to a cumulative regional decline toward a lower equilibrium growth rate, unless the income elasticity of demand for imports returns to the previous or lower values through an increase in the intraregional inter-industry linkages.
In addition to the above increase in import elasticity, if the damaged region were contaminated by some undesirable sources, such as oil spills on the shoreline, the spread of radioactive materials due to a nuclear accident, and so forth, the demand for the products in the damaged region from the outside would be affected adversely based on rumors and asymmetry of information about such contamination, even if the products are tested as safe. This situation can be analyzed with the Keynesian growth model as in Fig. 4.5. The income elasticity of demand for import of the damaged region increases to πd as in Fig. 4.4, and this leads to a lower growth rate of labor productivity. Since consumers in other regions are afraid to consume the products from the damaged region because of potential but unfound contamination caused by the disaster, this makes the other regions’ income elasticity of demand for exports from the damaged region lower, shifting leftwards the line in the lower right-hand quadrant in Fig. 4.5. The gray lines represent the effects of the decreased demand for exports, and it results in a much lower export growth rate at xd2. This results in a further cumulative regional decline, and a set of new equilibrium growth rates becomes even smaller than the above case.
Up to this point, the reconstruction activities are not included in this framework. The intense demand injections of reconstruction activity are certainly a demand-side phenomenon, but cannot be reflected through any of the parameter values in the above Keynesian growth model. Because the parameters signify the economic and production structures in a region, such as changes in export-oriented production or the intensity of intraregional production linkages, temporary increases in production level through reconstruction cannot be handled directly. Meanwhile, if the reconstruction was financed mainly by the national government located outside of the damaged region, the reconstruction demand can be assumed to be a short-run increase in exports because it can be considered as money flowing in from outside of the region and invested within the region. This could lead to a temporary increase in the income elasticity of demand for exports from the other region, ε, and the upward shift of the line in the lower right-hand quadrant of Fig. 4.4. This will further yield a larger growth rate of exports, then a larger growth rate of output. However, this and the increase in income elasticity of demand for imports could be just a temporary event and will not lead to any long-run structural change of the regional economy. Or, the reconstruction policy could create significant changes in regional economic structure, leading to a permanent deviation of these parameter values from the previous ones. Whether the damaged region exhibits cumulative decline or growth is contingent on how the damaged region is recovered and reconstructed.
The results from the Keynesian growth model for disaster cases look different from the neoclassical counterparts. While neoclassical growth models predict the convergence to a steady state even after a catastrophic disaster, the Keynesian model elucidates a possibility of the cumulative decline (or growth) resulting from the disaster, unless the damaged region overcomes the dependency on imports during the reconstruction period, and/or resolves the asymmetry of information for regaining confidence in regionally produced goods. In terms of technology, as seen in Fig. 4.4, the increase in the income elasticity of demand for imports in the damaged region leads to a lower level of output growth rate, and through Verdoorn’s Law, the growth rate of labor productivity becomes lower. This appears contradictory to the notion of technology updates during the reconstruction period discussed in Okuyama (2003). As discussed in Sect. 4.3, the Hallegatte and Dumas (2009) model based on the neoclassical growth model predicts that there will be no technological progress resulting from the reconstruction process, while the production level is boosted by the intense injection of reconstruction demand. At the same time, the neoclassical open economy model, discussed above, showed that if the capital-labor ratio becomes smaller due to the loss of some capital by a disaster, labor in the damaged region will migrate to other regions until the capital-labor ratio reaches a new equilibrium. If this labor migration includes the movement of human capital, and if the labor with higher human capital finds it easier to secure jobs elsewhere, this out-migration from the damaged region will lead to lower technological capacity in the damaged region. These implications of the neoclassical open economy model and of the Keynesian growth model appear consistent with each other, while the models are based on different perspectives.
5 Conclusions
The long-run effects of disasters have been empirically studied both from cross-country and cross-section statistical analyses and from more detailed event-specific investigations. While the cross-country and cross-section studies using socio-economic indicators of disaster damage, such as the number of casualties and/or economic damages, have provided conflicting results in terms of the relationship between disasters and economic growth, more recent research employing physical intensity indicators as explanatory variables, like the Richter scale for earthquakes and maximum wind speed for storms, found statistically significant negative relationships. As Skidmore and Toya (2002), Noy (2009), and Albala-Bertrand (2013) argued, the socio-economic indicators appear unreliable for statistical analysis because of their non-standardized definition and the endogeneity problem, which lead to biased estimates of the relationship.
The findings from the studies using physical indicators are well in line with the predictions of neoclassical growth models, in which the decrease in production capital by a natural hazard leads to a lower per capita income, and the subsequent capital accumulations through recovery and reconstruction temporarily bring a higher growth rate toward the original steady state. The analysis in Sect. 4.3 provides the transitional dynamics of recovery and reconstruction, and the changes in saving rate become a determinant of recovery speed. In this context, the saving rate can be seen as a policy instrument during the recovery and reconstruction period. While the neoclassical growth models can simulate the effects of decreased capital and the process of recovery and reconstruction, they also predict that the declined level of per capita production grows with re-accumulation of capital and inevitably converges to the steady-state level, achieving a full recovery in the end. However, some empirical studies reported cumulative negative effects on per capita production after a disaster, such as Hsiang and Jina (2014) with a cross-country analysis, and Chang (2010), DuPont and Noy (2015), and Okuyama (2016) with the 1995 Kobe earthquake case.
As Okuyama (2014, 2015) revealed with the 1995 Kobe Earthquake case, significant economic structural changes occurred during the reconstruction period and resulted in a prolonged slump of the Kobe economy. This type of structural change can be adapted in the Keynesian growth model through changes in the parameters of income elasticities of demand for imports and of demand for exports from the damaged region, as described in Sect. 4.4. In particular, Okuyama (2015) found that the intraregional inter-industry linkages among manufacturing sectors in the Kobe economy became temporarily strengthened right after the earthquake through providing originally imported intermediate inputs within the damaged region, but were weakened after several years due partly to the underlying hollowing-out process, in which the dependency on and demand for imports increased. This type of change could be reflected by the income elasticity of demand for imports: the increase in intraregional inter-industry linkages translates to a lower income elasticity of import demand, while the following weakened linkages are made through a higher income elasticity for imports. These features make the Keynesian growth model able to simulate cumulative effects of disasters, which should be empirically examined. Hence, the Keynesian growth model can be considered for analysis during the early stage of recovery, because it allows non-equilibrium adjustments, high-level unemployment, and under-utilization of capital. Yet, the neoclassical growth models fit better with the impact analysis of the reconstruction period, when the damaged capital stocks are being re-accumulated. Because the neoclassical models in this chapter are supply-side models and the Keynesian models are on the demand side, it seems intriguing to link between these two perspectives for a more integrative analysis of the disaster process in the long-run.
Most of the empirical studies discussed in Sect. 4.2 investigated the effects of disasters on national economies, typically from the neoclassical perspective. As Felbermayr and Gröschl (2014) claimed, however, these empirical findings sometimes contradict the predictions of the neoclassical growth model, in which losses of capital lead to a higher growth rate afterwards for converging to the steady state. They found a negative impact on per capita GDP but not a higher growth rate in later periods. Cavallo et al. (2013) even argued that the neoclassical growth theory does not have a clear-cut answer to the question of whether or how disasters affect the growth path of an economy, and concluded that it is ultimately an empirical question. In this respect, the empirical studies from the Keynesian perspective could provide new insights to the question at hand. Meanwhile, as Albala-Bertrand (2007) asserted that a disaster causes localized damages and losses on capital and activities but may not affect negatively (or positively) larger economies, such as a national economy, in both short- and longer-runs, more empirical studies about the relationship between disaster and economic growth at the regional level, rather than at the national level, are desired. Such empirical studies at the regional level to date include Noy and Vu (2010) using the sub-national regions in Vietnam, and Tapia and Pinã (2014) based on the sub-national regions in Mexico. Further empirical analyses at a regional level, which can refine the theoretical analysis of disaster effects in the long-run, are also highly anticipated for a better understanding of disaster effects on economic growth.
Notes
- 1.
The excellent summary and discussions on this endogeneity problem in regression analysis on climate change-related literatures are found in Dell et al. (2014). Much of the recent research in that field has applied panel methods for the analysis.
- 2.
While Felbermayr and Gröschl (2014) found the decline in per capita output by disasters, they did not find the faster growth after the disaster that the neoclassical model foresees toward the convergence to a steady state.
- 3.
This assumption of ‘no technological progress’ will be relaxed and discussed later in this section.
- 4.
Even if an economy is not at the steady state, the results of the following analysis still apply.
- 5.
- 6.
The detailed formation of the model and the derivation of its solution can be found, for example, in Barro and Sala-i-Martin (2004).
- 7.
More detailed discussion about behavior of saving rate in general can be found at pages 106–110 in Barro and Sala-i-Martin (2004).
- 8.
The term regions, used for the discussion in this and following sections, implies sub-national areas, rather than regional blocs consisting of multiple countries. Therefore, the effects of currency exchange rate, trade restrictions, and so on can be neglected in the analysis below.
- 9.
This depends on the availability of production factors in other regions, as well as on interregional trade patterns. Examining such trade relationships requires a multi-sectoral model, but it is out of the scope of this chapter. Interested readers can consult with such literatures, for recent example, Koks et al. (2016) and Koks and Thissen (2016).
- 10.
This interregional adjustment model is refereed as the ‘one-sector’ neoclassical model of factor allocation and migration (McCann 2013).
- 11.
The analysis of long-run changes in migration pattern becomes important if a disaster leads to a negative net migration rate in the damaged region. Such cases include widespread terrorist attacks in a region, the surrounding areas in a nuclear accident case, and so forth.
- 12.
Short-run analysis of disaster impact has been performed with demand-side changes using a multi-sector model, such as input-output and computable general equilibrium (CGE) models. For example, Rose et al. (2017) utilized a CGE model with a ‘Keynesian closure rule’ with the account balance constraint, allowing for unemployment equilibrium to examine the impact of terrorist attacks on U.S. air travel target.
- 13.
- 14.
McCann (2013) elucidated the reasons for this assumption, including the one that transportation costs and spatial competition over regions suggest that differences in nominal prices among regions remain relatively stable in the long-run.
- 15.
References
Albala-Bertrand JM (1993a) Natural disaster situations and growth: a macroeconomic model for sudden disaster impacts. World Dev 21(9):1417–1434
Albala-Bertrand JM (1993b) The political economy of large natural disasters: with special reference to developing countries. Clarendon Press, Oxford
Albala-Bertrand JM (2007) Globalization and localization: an economic approach. In: Rodriguez H, Quarantelli EL, Dynes RR (eds) Handbook of disaster research. Springer, New York, pp 147–167
Albala-Bertrand JM (2013) Disasters and the networked economy. Routledge, Oxon
Baade RA, Baumann R, Matheson V (2007) Estimating the economic impact of natural and social disasters, with an application to hurricane Katrina. Urban Stud 44(11):2061–2076
Barro RJ, Sala-i-Martin X (2004) Economic growth: second edition. The MIT Press, Cambridge, MA
Berlemann M, Wendzel D (2016) Long-term growth effects of natural disasters: empirical evidence from droughts. Econ Bull 36(1):464–476
Calzadilla A, Pauli F, Roson R (2007) Climate change and extreme events: an assessment of economic implications. Int J Ecol Econ Stat 7:5–28
Cass D (1965) Optimum growth in an aggregative model of capital accumulation. Rev Econ Stud 32(3):233–240
Cavallo E, Galiani S, Noy I, Pantano J (2013) Catastrophic natural disasters and economic growth. Rev Econ Stat 95:1549–1561
Chang SE (2010) Urban disaster recovery: a measurement framework and its application to the 1995 Kobe earthquake. Disasters 34(2):303–327
Coffman M, Noy I (2011) Hurricane Iniki: measuring the long-term economic impact of a natural disaster using synthetic control. Environ Dev Econ 17:187–205
Cuaresma JC, Hlouskova J, Obersteiner M (2008) Natural disasters as creative destruction? Evidence from developing countries. Econ Inq 46:214–226
Dacy DC, Kunreuther H (1969) The economics of natural disasters: implications for federal policy. The Free Press, New York
Dell M, Jones BF, Olken BA (2014) What do we learn from the weather?: the new climate-economy literature. J Econ Lit 52(3):740–798
Dixon R, Thirlwall AP (1975) A model of regional growth rate differences on Kaldorian lines. Oxf Econ Pap 27(2):201–214
DuPont W, Noy I (2015) What happened to Kobe?: a reassessment of the impact of the 1995 earthquake. Econ Dev Cult Chang 63(4):777–812
Felbermayr G, Gröschl J (2014) Naturally negative: the growth effects of natural disasters. J Dev Econ 111:92–106
Fomby T, Ikeda Y, Loayza NV (2013) The growth aftermath of natural disasters. J Appl Econ 28(3):412–434
Fujiki H, Hsiao C (2013) Disentangling the Effects of Multiple Treatments – Measuring the Net Economic Impact of the 1995 Great Hanshin-Awaji Earthquake, IMES Discussion Paper 2013-E-3, Institute for Monetary and Economic Studies, Bank of Japan
Hallegatte S, Dumas P (2009) Can natural disaster positive consequences?: investigating the role of embodied technical change. Ecol Econ 68:777–786
Hallegatte S, Hourcade J, Dumas P (2007) Why economic dynamics matter in assessing climate change damages: illustration on extreme events. Ecol Econ 62:330–340
Harris R (2008) Models of regional growth: past, present and future, SERIC Discussion Paper 2, Spatial Economics Research Centre, UK
Healy A, Malhotra N (2009) Myopic voters and natural disaster policy. Am Polit Sci Rev 103:387–406
Hornbeck R (2009) The enduring impact of the American dust bowl: short and long run adjustments to environmental catastrophe, NBER Working Paper Series: W15605
Hsiang SM, Jina AM (2014) The causal effect of environmental catastrophe on long-run economic growth: evidence fro 6,700 cyclones, NBER Working Papers 20352, National Bureau of Economic Research
Koks EE, Thissen M (2016) A multiregional impact assessment model for disaster analysis. Econ Syst Res 28(4):429–449
Koks EE, Carrera L, Jonkeren O, Aerts JCJH, Husby TG, Thissen M, Standardi G, Mysiak J (2016) Regional disaster impact analysis: comparing input-output and computable general equilibrium models. Nat Hazards Earth Syst Sci 16:1911–1924
Koopmans TC (1965) On the concept of optimal economic growth. In: The econometric approach to development planning. North Holland, Amsterdam
McCann P (2013) Modern urban and regional economics, 2nd edn. Oxford University Press, Oxford
McCombie JSL, Thirlwall AP (1994) Economic growth and the balance-of-payments constraint. Palgrave Macmillan, London
Nijkamp P, Poot J (1998) Spatial perspectives on new theories of economic growth. Ann Reg Sci 32:7–37
Noy I (2009) The macroeconomic consequences of disasters. J Dev Econ 88:221–231
Noy I, Vu TB (2010) The economics of natural disasters in a developing country: the case of Vietnam. J Asian Econ 21:345–354
Odell KA, Weidenmier MD (2002) Real shock, monetary aftershock: The San Francisco earthquake and the panic of 1907, NBER Working Paper Series: W9176
Okuyama Y (2003) Economics of natural disasters: a critical review, Research Paper 2003–12, Regional Research Institute, West Virginia University
Okuyama Y (2014) Disaster and economic structural change: case study on the 1995 Kobe earthquake. Econ Syst Res 26(1):98–117
Okuyama Y (2015) How shaky was the regional economy after the 1995 Kobe earthquake?: a multiplicative decomposition analysis of disaster impact. Ann Reg Sci 55(2):289–312
Okuyama Y (2016) Long-run effect of a disaster: case study on the Kobe earthquake. Singap Econ Rev 16(1):1640009-1-18
Ramsey F (1928) A mathematical theory of saving. Econ J 38(152):543–559
Rose A, Avetisyan M, Rosoff H, Burns WJ, Solvic P, Chan O (2017) The role of behavioral responses in the total economic consequences of terrorist attacks on U.S. air travel targets. Risk Anal 37(7):1403–1418
Skidmore M, Toya H (2002) Do natural disasters promote long-run growth? Econ Inq 40(4):664–687
Solow RM (1956) A contribution to the theory of economic growth. Q J Econ 70(1):65–94
Solow RM (1962) Technological progress, capital formation, and economic growth. Am Econ Rev 52(2):70–86
Strobl E (2012) The economic growth impact of natural disasters in developing countries: evidence from hurricane strikes in the central American and Caribbean regions. J Dev Econ 97:130–141
Swan TW (1956) Economic growth and capital accumulation. Econ Rec 32(2):334–361
Tapia AG, Pinã CM (2014) The effect of natural disasters on Mexico’s regional economic growth: growing disparity or creative destruction? Working Paper Series WP68, Latin American and Caribbean Environmental Economic Program
Thirlwall AP (1980) Regional problems are ‘balance-of-payments’ problems. Reg Stud 14:419–425
Author information
Authors and Affiliations
Corresponding author
Editor information
Editors and Affiliations
Rights and permissions
Copyright information
© 2019 Springer Nature Switzerland AG
About this chapter
Cite this chapter
Okuyama, Y. (2019). Disaster and Economic Growth: Theoretical Perspectives. In: Okuyama, Y., Rose, A. (eds) Advances in Spatial and Economic Modeling of Disaster Impacts. Advances in Spatial Science. Springer, Cham. https://doi.org/10.1007/978-3-030-16237-5_4
Download citation
DOI: https://doi.org/10.1007/978-3-030-16237-5_4
Published:
Publisher Name: Springer, Cham
Print ISBN: 978-3-030-16236-8
Online ISBN: 978-3-030-16237-5
eBook Packages: Economics and FinanceEconomics and Finance (R0)